
Recent Advances in Augmented Reality Through Machine Learning Techniques
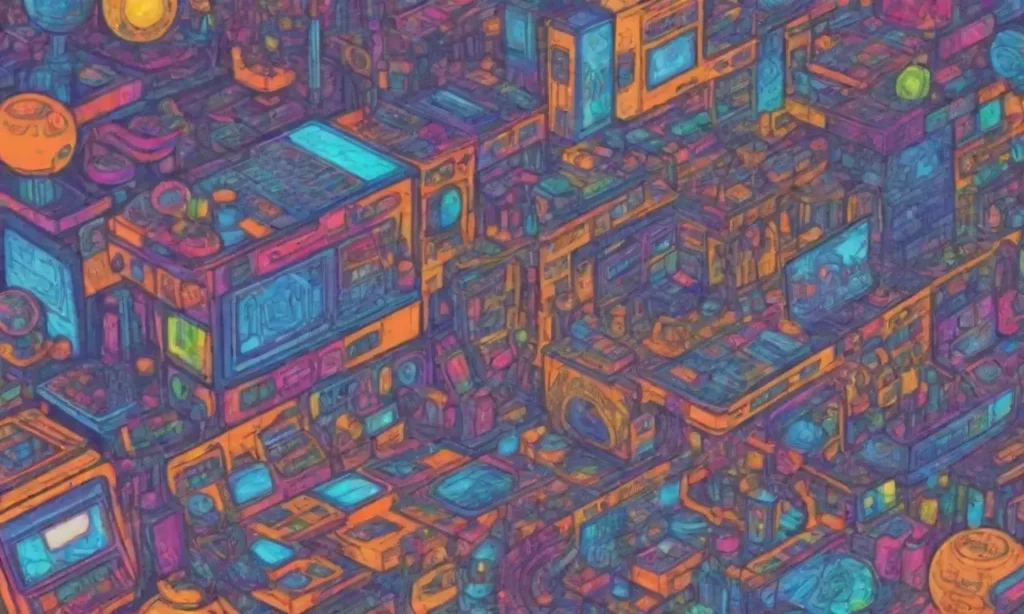
Introduction
Augmented Reality (AR) technology has rapidly evolved in recent years, largely influenced by the strides made in Machine Learning (ML). This intersection of AR and ML not only enhances users' experiences but also opens new possibilities for applications in various fields, such as gaming, education, healthcare, and industrial sectors. The synergy between these technologies signifies a robust shift towards more intelligent and immersive applications, paving the way for innovative tools that can seamlessly blend the physical and digital worlds.
This article will delve into the recent advancements in augmented reality driven by machine learning techniques. We will explore how these technologies intersect, highlight significant breakthroughs in the field, and discuss current applications that exemplify the future potential of AR powered by ML. By the end of this exploration, readers will have a comprehensive understanding of how machine learning is dramatically reshaping the landscape of augmented reality and what that could mean for the future.
The Role of Machine Learning in Augmented Reality
Machine learning serves as the backbone of numerous augmented reality applications. By enabling systems to learn from data and improve through experience, ML enhances AR technology in ways previously thought unattainable. For instance, traditional AR systems often relied on rigid algorithms for object recognition and tracking, which could lead to limited functionality and poor user experiences. In contrast, machine learning offers adaptive algorithms capable of improving accuracy and speed by leveraging vast sets of training data.
The most significant progress in this area involves computer vision techniques such as image recognition, object detection, and scene understanding. These advancements allow AR systems to interpret their surroundings more effectively, recognizing not just individual objects but also spatial relationships and environments. When an AR device uses ML to interpret visual data, it can interact with users more naturally by responding to contextual information. For example, an AR app that recognizes a chair and provides information about its style or functionality can offer more valuable insights compared to traditional systems.
How Machine Learning Transforms 3D Object Recognition in ARFurthermore, ML helps overcome challenges presented by environmentally variable conditions and physical obstructions that can impede system performance. Using deep learning methods, augmented reality devices can be trained with diverse datasets to ensure they can accurately identify and track objects under various circumstances. This leads to a more universal application of AR technology, whether users are in well-lit environments or more challenging situations with noise and dynamic movements.
Breakthroughs in Object Recognition
One of the most crucial advancements in the integration of ML within AR is the enhancement of object recognition capabilities. Modern ML models, particularly convolutional neural networks (CNNs), have made significant strides in accurately identifying objects in real-time. For instance, they can analyze visual inputs swiftly, categorizing objects and generating detailed metadata, thus allowing better interactions between users and their environments.
Recent developments have also led to the emergence of semantic segmentation techniques, which allow devices to not only detect objects but also understand their shapes and boundaries more precisely. Through this understanding, augmented reality applications can provide spatial information about the object's position or size, aiding in tasks like architectural design or retail display. An example includes IKEA's AR app, which allows users to visualize how different furniture pieces would fit into their spaces, using advanced object detection and dimensional analysis powered by machine learning.
Another significant contributor to object recognition's effectiveness is the incorporation of transfer learning. This method involves taking a pre-trained model—trained on a large dataset—and fine-tuning it for specific AR tasks. This drastically reduces the amount of data needed for training while maintaining high recognition accuracy. Consequently, AR applications can be designed and deployed faster, resulting in quicker iterations and more user-friendly updates.
Augmented Reality and Machine Learning: Bridging the GapEnhancing User Interactions and Experiences
Machine learning techniques also play an essential role in improving user interactions within augmented reality environments. By employing algorithms that analyze user behavior, preferences, and real-time feedback, developers can create more intuitive experiences tailored to individual needs. For instance, AR applications can learn user habits and adjust the displayed content based on historical interactions, making the experience more engaging and relevant.
Moreover, Natural Language Processing (NLP) techniques in machine learning allow users to interact with AR applications using voice commands or chat interfaces. This increased accessibility enables non-technical users to engage with AR technology more interactively, expanding its reach beyond specialized fields like gaming or design. For example, an AR-powered educational tool could allow students to ask questions about historical artifacts they see in museums and receive immediate augmented information or visual aids to deepen their understanding.
Another noteworthy improvement lies in the development of gesture recognition systems that leverage ML algorithms. By utilizing models trained on vast datasets of human gestures, AR systems can recognize movements and translate them into actions within a virtual environment. This application can be particularly useful in industries such as healthcare, where doctors could use hand gestures to manipulate charts and data without needing to touch the screens directly, thereby maintaining hygiene.
Current Applications Showcasing AR and ML Synergy
Gaming Industry Innovations
One of the most visible applications of AR powered by machine learning is in the gaming industry. Games like Pokémon GO demonstrate how AR can enhance interactive experiences by overlaying digital elements in real-world settings. Machine learning plays a vital role in making interactions with these elements more fluid and engaging. For instance, the game uses ML algorithms to track player movements, ensuring that virtual creatures appear to interact correctly with their surroundings.
Understanding the Ethics of Machine Learning in Augmented RealityInnovations like adaptive game mechanics are also being explored, where systems adjust challenges based on players’ skills or behaviors. This not only enhances user retention but also provides a more personalized gaming experience that can cater uniquely to each player's style and preferences.
Education and Training Tools
In the domain of education, AR applications enriched with machine learning offer transformative learning experiences. Imagine students engaging in interactive learning modules that allow them to visualize complex concepts through mixed-reality environments. For example, platforms like Google Expeditions enable students to participate in virtual field trips, while machine learning analyzes their learning patterns and preferences, adjusting the presented material accordingly.
Medical training is another area where AR and ML converge impressively. Augmented reality applications provide medical students with immersive simulations of surgeries, where they can practice procedures in a controlled, risk-free environment. AI-driven systems track participants' progress and suggest areas for improvement based on their performance, offering real-time feedback that enhances their learning experience.
Industrial Applications
Industries also benefit significantly from the integration of augmented reality with machine learning technologies. Take, for example, manufacturing and maintenance sectors. AR solutions can guide technicians through complex repair or assembly processes using step-by-step instructions overlayed on real-world items. Machine learning algorithms can analyze the data collected and identify recurrent issues, which can then be addressed proactively in future designs or maintenance schedules.
Furthermore, warehouse management is another area ripe for innovation. AR applications can facilitate inventory checks by superimposing digital information over actual stock, guiding workers seamlessly through their tasks. Machine learning can optimize stock management by analyzing patterns, predicting future needs, and streamlining supply chain processes, making operations more efficient and cost-effective.
Conclusion
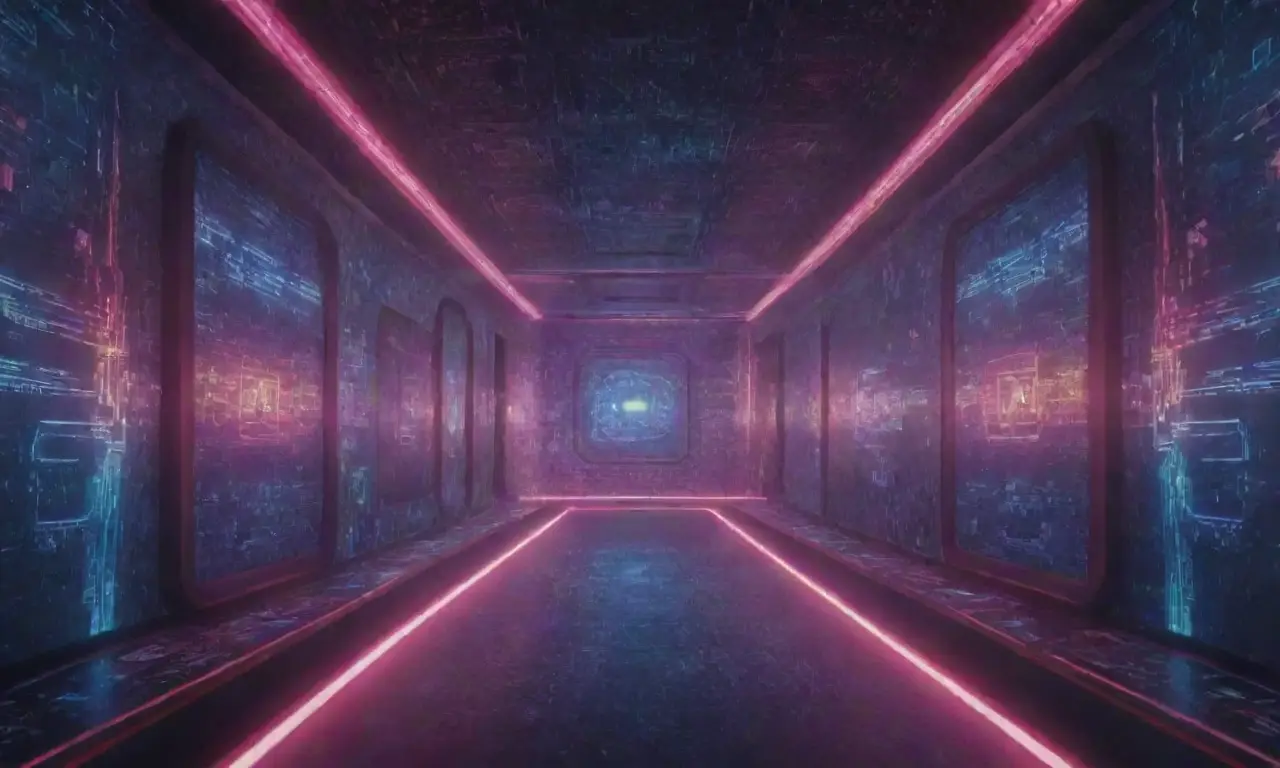
The convergence of augmented reality and machine learning is transforming industries and creating a new era of interactive technology. The developments within this realm are exciting, with rapid advancements improving user experiences in gaming, education, healthcare, and various industrial applications. As machine learning techniques continue to evolve, they will pave the way for even more groundbreaking applications and innovations that challenge our understanding of reality.
The potential of AR powered by machine learning reshapes how we interact with and perceive our environments. As we see from successful applications in gaming and education, this synergy holds countless possibilities. As organizations and developers harness these technologies, we can expect continued enhancements that will improve user interactions, expand accessibility, and hopefully democratize access to augmented realities for all.
Moving forward, the collective focus should be not only on enhancing the technology but also addressing ethical and societal impacts. As augmented reality becomes increasingly integrated into our daily lives, considerations around privacy, accessibility, and the digital divide must be paramount. By embracing a thoughtful and collaborative approach to these advanced technologies, we can ensure a future that enriches human experience and fosters positive growth in every industry.
If you want to read more articles similar to Recent Advances in Augmented Reality Through Machine Learning Techniques, you can visit the Augmented Reality category.
You Must Read