
Geospatial Analysis with Machine Learning: Urban Case Studies
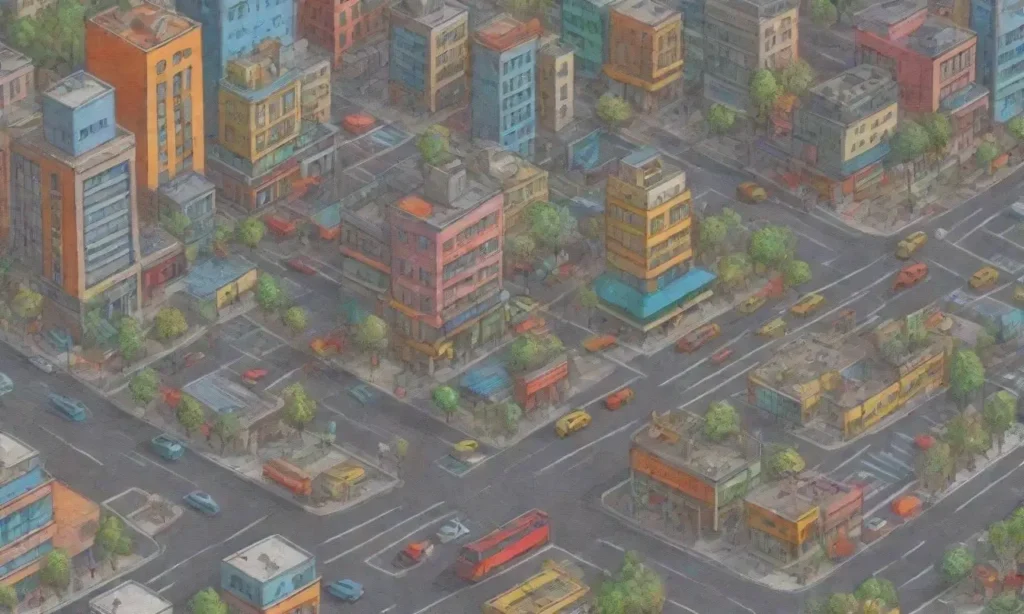
Introduction
Geospatial analysis is a powerful methodology used in various sectors, from urban planning and environmental studies to transportation and public health. It involves the examination of data with geographical or spatial attributes to explore relationships, patterns, and trends. As urbanization continues to accelerate globally, understanding the dynamics within urban environments becomes increasingly vital. This is where the integration of machine learning and geospatial analysis comes into play, offering unprecedented opportunities to analyze complex urban phenomena.
This article delves into the intricate weave of geospatial analysis and machine learning through a selection of compelling urban case studies. We will discuss how machine learning models enhance traditional geospatial tools, the challenges faced by urban ecosystems, and how artificial intelligence (AI) can help solve urban planning and management issues. Furthermore, we will navigate through different applications and methodologies, illustrating the synergy between technology and urban studies through real-world examples.
Understanding Geospatial Analysis
Geospatial analysis is a discipline that combines geospatial technology with various analytical methods, typically employed for understanding phenomena tied to specific locations or regions. With the burgeoning amount of data available from sources such as remote sensing, GPS, social media check-ins, and urban sensors, the importance of geospatial analysis has skyrocketed in urban studies.
Key Components of Geospatial Analysis
The core components of geospatial analysis include geometric, geographic, and spatial data, all of which are crucial for comprehensive urban studies. Geometric data refers to simple shapes and coordinates, whereas geographic data provides context, detailing real-world geographic features. Finally, spatial data encompasses information about the location, size, and relationships of objects within a defined space. Geospatial analysis effectively combines these data types to produce insightful analytics.
Data-Driven Restore Urban Spaces: Machine Learning Success StoriesMoreover, gas emissions, traffic patterns, demographic shifts, and economic status can all be spatially visualized, revealing trends that plain numerical data could obscure. For instance, urban planners can utilize geospatial analysis to identify areas with high traffic congestion, thereby facilitating targeted transportation improvements. This enriches decision-making processes, allowing urban authorities to develop smarter, efficient cities.
The Role of Machine Learning
Machine learning, a subset of AI, applies algorithms to analyze data, identify patterns, and make decisions with minimal human intervention. When melded with geospatial analysis, machine learning can process vast datasets to uncover insights that were previously unattainable. By employing techniques such as clustering, classification, and regression, machine learning can significantly enhance the ability to conduct effective geospatial analyses.
For instance, predictive modeling can be employed to forecast urban growth patterns, while clustering algorithms can help identify areas susceptible to gentrification. These techniques can optimize city planning and development, directly translating into improved community well-being. By leveraging machine learning, city planners gain a robust toolkit for tackling complex urban challenges through data-driven insights.
Application of Geospatial Analysis in Urban Case Studies
Geospatial analysis integrated with machine learning has manifested in numerous applications. We will now explore a variety of case studies demonstrating how these approaches revolutionize urban environments.
Predictive Maintenance for Urban Infrastructure: The Role of MLUrban Traffic Management
One notable example is the application of geospatial analysis and machine learning in urban traffic management. Researchers in Los Angeles utilized machine learning algorithms to evaluate real-time traffic data from sensors across the city. By employing geospatial clustering techniques, they could pinpoint traffic hotspots and develop predictive models to forecast congestion patterns throughout the day.
This analysis offered city planners essential insights, allowing them to program traffic lights dynamically and improve city layouts for optimal vehicle flow. A significant reduction in commuting times and an enhancement in overall public transit efficiency were achieved through this intelligent data usage.
Moreover, similar methodologies have been aimed at reducing the environmental impact of traffic by identifying the best routes for electric vehicles (EVs), thus promoting sustainable urban transportation options.
Land Use Optimization
Land use optimization stands as another critical area where machine learning and geospatial analysis converge to improve urban planning. A relevant case study is the city of Singapore, which leverages machine learning for effective land use planning. The Singapore government has turned to smart city technologies to collect extensive datasets on land usage, population density, and green space allocation.
Urban Land Use Predictions: Leveraging Machine Learning ApproachesUsing machine learning models, city planners can simulate various land-use scenarios and analyze their potential impacts. For example, a simulation might analyze how increasing green space in certain districts could affect local cooling temperatures and improve residents' quality of life. This data-driven approach has enabled a strategic allocation of resources and has facilitated intelligent land management, making Singapore one of the most efficient urban spaces globally.
Disaster Risk Management
Disaster management is an area where geospatial analysis and machine learning have proven invaluable. In vulnerable urban environments, where the risk of natural disasters like floods and earthquakes looms, predictive modeling becomes paramount. The case of New York City illustrates the use of machine learning to assess flood risks.
Researchers integrated historical flood maps, rainfall data, and geographical features into a machine learning framework, which allowed them to predict potential flooding under various scenarios. By employing machine learning classification algorithms, they could classify areas based on their susceptibility to flooding during extreme weather events.
Armed with these insights, city planners have enhanced emergency preparedness measures and optimized response strategies during crises. This proactive approach reduces risks and extends beyond merely responding to disasters; it fosters community resilience in the face of inevitable environmental challenges.
Real Estate Development: Machine Learning Insights for Predictive AnalysisChallenges and Considerations

While the prospects are promising, integrating machine learning with geospatial analysis does encounter several challenges. As urban data sources continue to grow exponentially, the complexity of managing, processing, and analyzing this data rises alongside.
Data Quality and Availability
One of the primary challenges is ensuring data quality. Deriving actionable insights requires accurate, high-resolution data. Often, urban datasets are collected intermittently or suffer from inaccuracies stemming from various sources. In such cases, flawed data can lead to misleading conclusions. Therefore, practitioners must establish robust data validation processes to maintain the integrity of their analyses.
Additionally, the availability of data can create disparities in urban planning and development. While affluent urban areas may have access to copious amounts of data, disadvantaged regions may not. This presents ethical considerations, as relying on available data might inadvertently perpetuate inequities in urban planning.
Realizing Smart Growth: Best Practices for Implementing ML StrategiesTechnical Expertise
Another challenge lies in the technical expertise needed to harness machine learning models for geospatial analysis. The successful integration of these technologies demands professionals with backgrounds in data science, computer programming, and urban planning. As the gap between technological advancement and human capacity grows, addressing this skills deficiency becomes crucial in maximizing the potential of geospatial analysis.
Conclusion
The intersection of geospatial analysis and machine learning has become a cornerstone for modern urban studies, paving the way for smarter, sustainable, and resilient urban societies. The case studies discussed illustrate the multifaceted ways in which these technologies can address urban challenges, from traffic congestion and land use optimization to disaster risk management.
As cities worldwide grapple with increased urbanization, environmental concerns, and inequalities, leveraging advanced analytics becomes ever more critical. The integration of geospatial analysis with machine learning not only provides urban authorities with powerful tools for informed decision-making but also embodies a shift towards data-driven urban governance that truly considers the needs of diverse communities.
To fully realize the benefits of these methodologies, overcoming challenges related to data quality, availability, and human expertise is vital. Training programs, enhanced data initiatives, and collaborative approaches between technologists and urban planners can foster a rigorous environment where solutions to urban issues flourish.
In conclusion, the potential for geospatial analysis coupled with machine learning is vast. As we move forward, it will be exciting to observe how these innovations reshape urban landscapes and lead to enhanced living conditions and sustainable frameworks for future generations.
If you want to read more articles similar to Geospatial Analysis with Machine Learning: Urban Case Studies, you can visit the Urban Planning category.
You Must Read