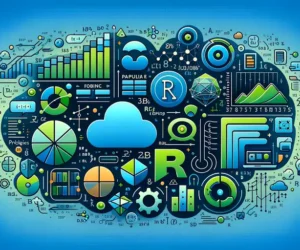
Predictive Maintenance for Urban Infrastructure: The Role of ML
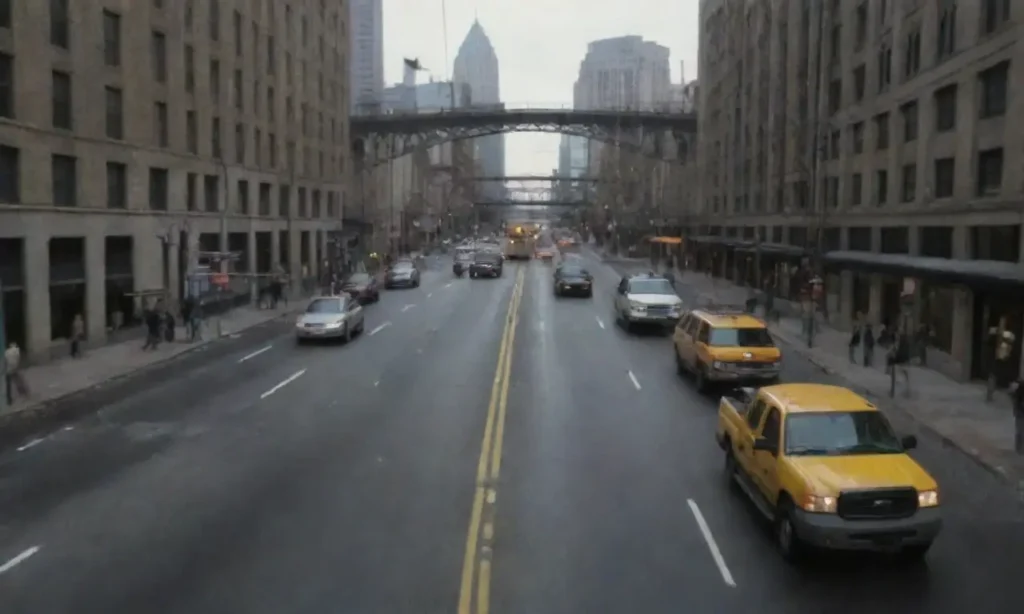
Introduction
In recent years, predictive maintenance has emerged as a critical component in the management of urban infrastructure, allowing cities to optimize their resources and manage their assets effectively. Urban infrastructure, which includes roads, bridges, water supply systems, and more, faces constant wear and tear due to increasing population growth and urbanization. As cities expand, the demand for reliable and efficient infrastructure becomes paramount to ensure safety, productivity, and a high quality of life for residents.
This article aims to explore the concept of predictive maintenance and the pivotal role that machine learning (ML) plays in transforming how urban infrastructure is maintained. We will delve into the various methodologies involved in predictive maintenance, the advantages of utilizing machine learning algorithms, real-world applications, and the future possibilities for smart cities driven by firmware and data management.
Understanding Predictive Maintenance
Predictive maintenance refers to techniques that leverage condition-monitoring tools and techniques to track the performance of urban infrastructure over time. This approach allows for maintenance to occur based on the current condition of an asset rather than following a predetermined schedule. The critical aspect of this strategy is data acquisition, which involves gathering real-time data from various sensors installed throughout urban infrastructures. These sensors could monitor structural integrity, detect vibrations, or measure movements that indicate wear or failure.
One of the primary benefits of predictive maintenance is the reduction in operational costs. Traditional maintenance approaches, which often involve periodic checks or reactive maintenance post-failure, can lead to unnecessary costs and asset downtime. In contrast, predictive maintenance minimizes disruption and allows for focused resource allocation. By anticipating issues before they escalate, municipalities can perform targeted repairs that prioritize public safety and asset longevity.
Urban Land Use Predictions: Leveraging Machine Learning ApproachesMoreover, predictive maintenance helps in extending the life of the infrastructure. By identifying emerging issues early, municipalities can implement the necessary reforms or reinforcements to ensure infrastructures remain functional and safe for users. This extending lifespan translates to significant cost savings and reduced downtime while maintaining service reliability, which is vital for public trust in urban services.
The Role of Machine Learning
Machine learning, a form of artificial intelligence, greatly enhances predictive maintenance by analyzing vast amounts of data to identify patterns and predict failures. ML algorithms can handle complex datasets that would be challenging for traditional statistical methods, making them invaluable in maintaining intricate systems like urban infrastructure. By generating data-driven forecasts, machine learning equips urban planners with actionable insights to establish maintenance schedules effectively.
Machine learning offers various algorithms and methodologies, including supervised learning, unsupervised learning, and reinforcement learning. Each of these has a unique approach to data analysis. For instance, supervised learning can use labeled datasets containing historical breakdown instances to train the model to recognize conditions leading to similar failures. Meanwhile, unsupervised learning can group similar behaviors in operational data that reveal hidden issues even if they haven't materialized yet. This adaptive nature of machine learning allows predictive models to stay relevant and continuously improve as more data becomes available.
In addition, machine learning facilitates real-time monitoring of conditions. As data flows in from sensors installed across a city's infrastructure, machine learning algorithms can process this information instantly. This rapid analysis allows for dynamic decision-making, meaning maintenance teams can intervene immediately when certain thresholds are crossed, preventing larger issues from developing. Thus, the synergy between predictive maintenance and machine learning can create a responsive urban infrastructure that can adapt to challenges in real-time.
Real Estate Development: Machine Learning Insights for Predictive AnalysisReal-World Applications

Cities worldwide are adopting predictive maintenance powered by machine learning to maintain their infrastructure effectively and efficiently. For example, transportation networks are increasingly utilizing ML models to predict road conditions, wear on pavements, and corrosion in bridges. By collecting data on traffic loads, climate influences, and historical maintenance records, these models can predict when and where maintenance should occur.
One case study exemplifying this is the city of Chicago, where predictive maintenance techniques have been implemented to manage their roadways and bridges. By utilizing ML algorithms that process historical data as well as real-time data gleaned from IoT sensors, Chicago's transportation department can allocate resources to repair specific roadways before the general public experiences any issues. This proactive approach has resulted in decreased emergency repairs and improved safety on the roads.
Another remarkable instance can be found in wastewater treatment facilities, where predictive maintenance is applied to monitor equipment performance. Machine learning algorithms analyze data from pumps, tanks, and pipelines to identify anomalies in functioning that could indicate potential malfunctions. By predicting equipment failure before it happens, cities can schedule maintenance without affecting the overall operation of the facility. Consequently, this approach has not only saved money but has also preserved public health standards.
Realizing Smart Growth: Best Practices for Implementing ML StrategiesChallenges in Implementing ML-Powered Predictive Maintenance
While the advantages of predictive maintenance through machine learning are manifold, various challenges exist in implementing these technologies in urban infrastructure. One of the primary hurdles is the availability and quality of data. For machine learning models to be effective, they require robust datasets to train on, which must be accurate and comprehensive. In many cases, cities may not have the existing infrastructure or systems to collect or store the necessary data effectively.
Additionally, integrating new machine learning technologies within existing urban infrastructure systems can be complex. Many municipalities have aging systems that may not accommodate the latest technological advancements without significant retrofitting costs. Budget constraints often limit the potential for investment in both updated infrastructure and the required sensor networks. This issue affects the scalability of predictive maintenance, preventing many cities from capturing its full potential benefits.
Moreover, ensuring public acceptance of machine learning technologies is another consideration. As cities adopt smarter technologies powered by AI, they must also contend with public concerns regarding privacy, surveillance, and accountability. Ensuring transparent processes and educating the community about the benefits of these initiatives can help alleviate fears and foster acceptance.
Future Directions for Smart Cities
The integration of predictive maintenance and machine learning is poised to significantly impact the future of smart cities. As urban areas continue to grow, the challenge of maintaining infrastructure becomes more pressing, highlighting the need for innovative solutions. Machine learning can play a vital role in predictive analytics, allowing cities to evolve from traditional maintenance approaches to proactive strategies that enhance safety and reduce costs.
Geospatial Analysis with Machine Learning: Urban Case StudiesLooking ahead, the concept of digital twin technology is being heavily researched in relation to predictive maintenance. Digital twins are virtual representations of physical assets that reflect their condition, performance, and behavior in real-time. When combined with machine learning analytics, they can provide invaluable insights into the health of infrastructure, enabling predictive maintenance teams to foresee potential failures and create highly informed maintenance strategies tailored to observed conditions.
Furthermore, as more urban areas become interconnected through the Internet of Things (IoT), the data streams available for analysis will increase exponentially. This vast increase in data can enhance machine learning models' accuracy and predictive capabilities, making maintenance tools even more robust. Additionally, collaboration between city officials, private organizations, and academic institutions in developing and deploying machine-learning algorithms can foster innovation and drive the evolution of urban infrastructure.
Conclusion
In summary, predictive maintenance, empowered by machine learning, represents a transformative shift in how urban infrastructure is managed and maintained. This approach not only enables cost-effective operations but also significantly enhances public safety and service reliability. The combination of predictive maintenance and machine learning offers urban planners the ability to anticipate and resolve issues before they adversely impact communities, thereby building resilience into city management practices.
However, for cities to maximize the benefits of predictive maintenance, they must navigate various challenges, including data quality, integration with existing systems, and public acceptance. Addressing these challenges requires cross-sector collaboration and progressive policy frameworks that prioritize innovation while ensuring accountability and transparency.
Data-Driven Restore Urban Spaces: Machine Learning Success StoriesThe future of urban infrastructure lies in embracing the smart city movement, where machine learning can revolutionize maintenance practices and enable cities to evolve into safer, more resilient environments. As technology advances and data becomes increasingly available, the possibilities for enhancing urban infrastructure through predictive maintenance are virtually limitless. Embracing these innovations not only benefits infrastructure management but ultimately enriches the lives of those who live within our urban environments.
If you want to read more articles similar to Predictive Maintenance for Urban Infrastructure: The Role of ML, you can visit the Urban Planning category.
You Must Read