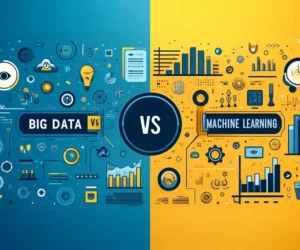
Navigating Real Estate Markets with Machine Learning Applications
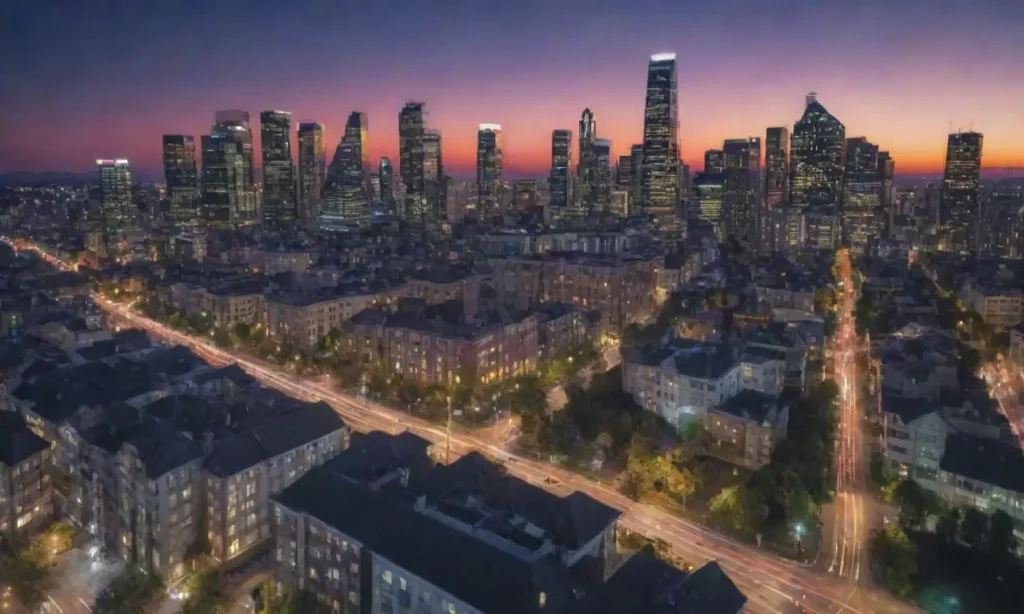
Introduction
In the rapidly evolving world of real estate, data has emerged as a crucial component that drives decision-making processes. Investors and developers alike are increasingly leveraging technology to understand market trends, forecast property values, and assess investment risks. One of the most revolutionary forces in this transformation is machine learning, a subset of artificial intelligence (AI) that enables systems to learn from data and improve their performance over time without explicit programming. As a result, the intersection of real estate and machine learning presents exciting opportunities for stakeholders looking to navigate complex markets with enhanced precision and insight.
This article explores the significance of machine learning in real estate, shedding light on its applications, benefits, and potential challenges. We will delve into how machine learning models function, the types of data they utilize, and the insights they can provide for various market players. Additionally, we will discuss the practical implementation of these technologies and their implications for the future of property investment.
The Role of Machine Learning in Real Estate
Understanding the pivotal role of machine learning in the real estate market requires a grasp of how these algorithms can process vast amounts of data. Machine learning algorithms can uncover patterns and trends in data sets that are too large and complex for human analysts to decode. By utilizing historical property data, market conditions, economic indicators, and demographic information, machine learning models can generate predictions about future property values and trends.
One of the most prevalent applications of machine learning in real estate is the development of predictive analytics. This technology allows real estate professionals to forecast market movements and property values with considerably higher accuracy than traditional methods. For instance, algorithms can analyze historical trends in home prices, rent prices, and economic conditions to predict how these variables will fluctuate in the future. This predictive capability not only aids investors in making informed decisions but also allows real estate agents to provide better advice to their clients.
Deep Learning Applications for Accurate Real Estate ValuationsFurthermore, machine learning enhances the property valuation process by identifying properties that align with specific investment criteria. Investors can input various parameters such as location, property size, and features, and receive recommendations for properties that fit their criteria. By automating this process, machine learning not only saves time but also increases the likelihood of identifying lucrative investment opportunities.
Machine Learning Applications in Real Estate
Predictive Modeling for Property Prices
Predictive modeling serves as one of the most significant applications of machine learning in real estate. These models analyze immense datasets to forecast how property prices will change over time. By evaluating variables such as location, economic indicators, crime rates, school district ratings, and local amenities, machine learning algorithms create comprehensive models that predict future price changes.
For instance, companies like Zillow and Redfin employ machine learning algorithms to generate Zestimate home values. They continually update their predictive models with fresh data to ensure their valuations accurately reflect market conditions. By using regression analysis and other statistical techniques, these platforms provide users with valuable insights, allowing buyers and sellers to make data-driven decisions.
Investors can also utilize predictive modeling to evaluate investment potential in emerging markets. By analyzing trends in comparable markets, machine learning can help identify regions that are gaining traction before they become popular, thus enabling investors to capitalize on growth opportunities.
The Role of Data Science in Modern Real Estate Valuation MethodsMarket Trend Analysis
Another prominent application of machine learning in real estate is market trend analysis. Analysts can leverage machine learning tools to monitor market trends in real-time, considering various factors such as interest rates, housing supply, and consumer sentiment. By employing algorithms that can process unstructured data, such as social media posts or news articles, real estate professionals gain a holistic view of market dynamics.
Machine learning also helps in sentiment analysis, which interprets public perception regarding real estate markets. By analyzing social media activity, online reviews, or search engine trends, real estate professionals can determine how public sentiment is shifting concerning certain neighborhoods or regions. This information is invaluable for real estate developers and investors who need to understand how the market responds to economic changes or social trends.
Moreover, machine learning tools can help identify seasonal trends in real estate, allowing agents and landlords to adjust their rental strategies and marketing efforts accordingly. With insights into peak seasons for property sales or rentals, stakeholders can optimize their inventory management and leasing terms.
Risk Assessment and Management
Risk assessment is a crucial consideration in real estate investment, and machine learning significantly enhances this aspect. Investors can use risk assessment models to quantify the financial risks associated with various properties. By evaluating large datasets containing market performance, economic trends, and historical data on price fluctuations, machine learning can produce risk scores that help investors understand potential downsides.
Developing Real Estate Valuation Models Using AI and ML TechniquesAdditionally, machine learning algorithms can identify risk factors that might not be immediately apparent. For example, a property’s proximity to future public works projects or proposed zoning changes can affect its value over time. Machine learning can analyze such data, providing valuable insights that may influence investment decisions.
Real estate companies can also apply machine learning for default prediction on mortgage applications by analyzing credit history, income, employment status, and other relevant factors. By predicting the likelihood of loan defaults, lenders can minimize their risks and make data-driven lending decisions.
Challenges of Implementing Machine Learning in Real Estate

Data Quality and Availability
While the potential of machine learning in real estate is enormous, challenges remain regarding data quality and availability. One significant obstacle is the inconsistency and fragmentation of real estate data. Unlike stock markets, which benefit from standardized data reporting, real estate data is often unstructured and varies widely from one location to another. Inaccurate or incomplete data can lead to flawed predictions and undermine the efficacy of machine learning models.
To overcome this issue, stakeholders must invest in data curation and cleaning processes. By ensuring clean and high-quality data, machine learning systems can function more efficiently and produce reliable outcomes. Additionally, the collaboration between tech companies and real estate firms to establish industry-wide data standards could pave the way for integrating machine learning into broader applications.
Interpretability of Machine Learning Models
Another challenge facing the real estate sector is the interpretability of machine learning models. While these algorithms can provide complex analyses and predictions, explaining their decision-making processes can be intricate. Investors and real estate professionals may find it challenging to trust models that operate as "black boxes," especially when selecting properties or making critical investment decisions.
To address this concern, the development of explainable AI (XAI) is essential. XAI focuses on creating machines that can explain their reasoning in human terms, enhancing transparency and user trust. By understanding how a model reaches its conclusions, stakeholders can make informed decisions based on solid reasoning rather than blind faith in technology.
Integration with Existing Systems
Successfully integrating machine learning solutions into existing real estate management systems presents another hurdle. Real estate firms often have entrenched processes and legacy systems that may resist the adoption of new technologies. Change management and employee training are critical components in this process, as employees must be comfortable using machine learning tools to derive insights.
Organizational leaders should focus on fostering a culture that embraces data-driven decision-making and continuous learning. Providing educational resources on machine learning and its applications can empower teams to leverage these technologies effectively, resulting in a smoother transition to innovative practices.
Conclusion
Navigating the ever-evolving landscape of real estate markets is no small feat. However, the emergence of machine learning applications is rapidly reshaping how investors, developers, and agents approach decision-making in this sector. From predictive modeling that forecasts property prices to market trend analysis and risk assessment, machine learning serves as a transformative force that can optimize strategies and enhance investment outcomes.
Despite the numerous benefits, it is essential for stakeholders to acknowledge the challenges associated with implementing machine learning in the industry—from data quality concerns to the interpretability of models. By prioritizing efforts to ensure data integrity, embracing explainable AI, and facilitating seamless integration into existing systems, the real estate industry can fully harness the potential of machine learning.
As technology continues to advance, machine learning will likely take center stage in shaping the future of property investment. By remaining open to innovation, real estate professionals will not only navigate market volatility more effectively, but they will also unlock new opportunities that emerge in an increasingly data-driven world. The fusion of real estate and machine learning may pave the way for unprecedented growth and efficiency within this essential industry, transforming how we perceive and engage with real estate markets.
If you want to read more articles similar to Navigating Real Estate Markets with Machine Learning Applications, you can visit the Real Estate Valuation category.
You Must Read