
The Role of Data Science in Modern Real Estate Valuation Methods
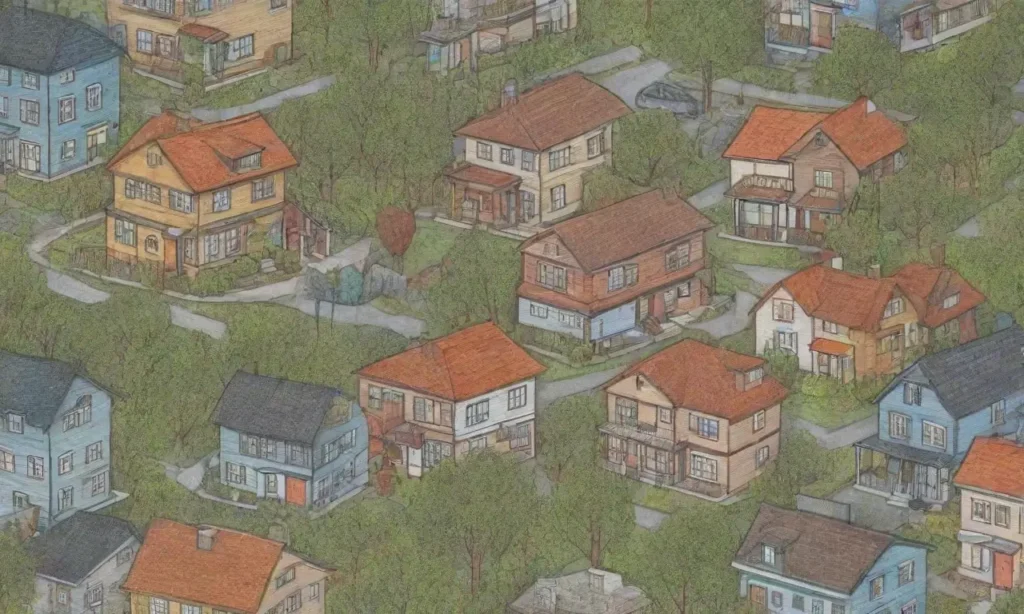
Content
Introduction
In today's rapidly evolving digital landscape, data science has emerged as a transformative force across various industries, and real estate is no exception. As the demand for accurate property valuations continues to soar, leveraging vast amounts of data has become not just beneficial but essential for investors, homeowners, and real estate professionals. The integration of advanced algorithms and analytical techniques has paved the way for more informed decision-making processes and enhanced valuation methodologies, ultimately changing how we perceive, invest in, and manage properties.
This article aims to explore the intricate relationship between data science and real estate valuation methods. We will dive into the various techniques employed in modern appraisal practices, highlight specific data science applications that provide deeper insights into market trends, and discuss the advantages and challenges associated with these methods. By the end of this read, you will have a comprehensive understanding of how data analytics is revolutionizing property valuation and its potential future implications.
Understanding Real Estate Valuation
Real estate valuation is the process of determining the economic value of a property, which serves as a critical component in buying, selling, or renting real estate. Valuation techniques can be grouped into several categories, including Sales Comparison Approach, Cost Approach, and the Income Approach. Each method has its unique focus, strengths, and limitations.
Sales Comparison Approach
The Sales Comparison Approach (SCA) is one of the most widely used methods for residential property valuation. In this technique, the appraiser looks at the recent sales of comparable properties in a specific area to provide a basis for gauging the value of the subject property. The appraiser considers factors such as property size, location, age, condition, and market trends when conducting these comparisons.
Navigating Real Estate Markets with Machine Learning ApplicationsHowever, the effectiveness of SCA can be compromised by fluctuating market conditions, leading to outdated or skewed valuations. This is where data science steps in. By employing big data analytics and machine learning algorithms, valuators can process immense datasets far beyond typical human capabilities. These include real-time property sales data, demographic shifts, economic indicators, and neighborhood trends. Utilizing such a comprehensive dataset helps yield more accurate valuation insights, adjusting for aspects that traditional methods might overlook.
Cost Approach
The Cost Approach involves estimating the cost to replace or reproduce the improvements on a property, minus any depreciation. This method is particularly useful for unique or special-use properties where comparable sales data may be limited. The three main components of this valuation are land value, construction costs, and depreciation.
Incorporating data science into the Cost Approach enables property appraisers to more effectively estimate construction costs by utilizing historical data analytics, regional cost indices, and supply chain metrics. Advanced predictive modeling offers insights into future construction costs, helping to accurately gauge depreciation trends. Additionally, data-driven insights can provide context and meaning to the $/sq. ft. cost changes influenced by regional variables, ultimately improving the accuracy of the valuation.
Income Approach
The Income Approach is predominantly used for commercial real estate, focusing on the property's ability to generate income over time. This method evaluates factors such as gross rental income potential, operating expenses, and financing costs to derive the Net Operating Income (NOI) and subsequently the cap rate, which then informs the property’s value.
Deep Learning Applications for Accurate Real Estate ValuationsThe challenge with the Income Approach lies in predicting future rental income, especially in diverse markets with fluctuating demands. Here, data science techniques, including predictive analytics and machine learning algorithms, can forecast rental prices by analyzing historical rental data, economic indicators, and other relevant data points. By building models that account for various scenarios, appraisers can obtain a more reliable understanding of income-producing properties, thereby arriving at more accurate valuations.
The Impact of Data Science on Valuation Techniques
As the world becomes increasingly data-driven, the intersection of real estate and data analytics has burgeoned, greatly influencing valuation techniques.
Enhanced Market Analysis
Data science has facilitated enhanced market analysis through the collection and processing of big data. The advent of online property listing services and platforms has resulted in the mass generation of data about properties, neighborhoods, and broader market trends. Data scientists can now utilize complex algorithms that sift through massive datasets to extract valuable insights relevant to property valuation.
Machine learning models allow for proficient pattern recognition, meaning that trends, price fluctuations, and market dynamics can be identified more quickly and accurately. This capability empowers real estate professionals to make more informed decisions and enhances their understanding of where to invest or how to approach property sales.
Predictive Modeling
The future of property valuation is heavily intertwined with predictive modeling powered by data science. By forecasting future real estate trends, investors and appraisers are better equipped to anticipate shifts in property values based on a multitude of variables, from changing zoning regulations to evolving demographic characteristics.
Predictive modeling takes historical data as inputs, allowing for the creation of regression models and decision tree analyses that project future prices. Such data-driven approaches not only anticipate trends but also help validate and refine current valuations based on reliable historical analogs. With powerful machine learning techniques, error rates can be significantly reduced, leading to more trustworthy assessments.
Automated Valuation Models (AVMs)
Automated Valuation Models (AVMs) are sophisticated tools increasingly utilized in real estate. These computer-based models employ statistical and algorithmic approaches to compute property values utilizing data sourced from public records, tax assessments, and demand-supply parameters. This innovation is especially appealing in the age where quick, reliable information is paramount.
AVMs can rapidly provide comprehensive valuations using data that reflects an area's specific dynamics, removing the inherent biases associated with human judgment. They can serve both institutional investors seeking rapid valuation assessments and individual homeowners wanting quick insights regarding the market value of their properties. Data science enhances AVMs by ensuring they consider a wide array of econometric and statistical data, cultivating assessments that are both innovative and accurate.
Challenges in Integrating Data Science within Real Estate Valuation

Despite the undeniable advantages of data science in real estate valuation, significant challenges must also be navigated.
Data Quality and Availability
The reliability of any data-driven approach hinges on the quality and availability of the data. In real estate, discrepancies exist in the availability of data across regions, with some lacking comprehensive datasets that are critical for accurate valuations. For businesses reliant on precise property valuations, this unpredictability can have tangible financial implications.
Moreover, data quality often fluctuates; properties that haven’t been updated within formal records or have inconsistencies hold the potential to skew analytical models. As such, significant investments in data curation, validation, and management practices are essential to ensure high-quality data remains at the backbone of valuation methods.
Skill Gap
The successful integration of data science within real estate valuation also hinges on the skills available within these organizations. While the demand for data scientists is surging across industries, real estate has been somewhat sluggish in embracing this transformation. There exists a noticeable skill gap, with many evaluating professionals lacking the analytical expertise required to use complex data effectively.
Organizations must invest in training and development programs to equip appraisers and real estate professionals with the essential data literacy skills. Navigating data science tools requires a new mindset and an understanding that traditional valuation techniques alone are insufficient in today’s data-driven environments.
Regulatory and Ethical Concerns
As data and technology continuously advance, so too do regulatory and ethical concerns surrounding data privacy and usage. Issues arise when using consumer data for valuations, raising potential privacy violations and escalation of biases in assessments. Algorithms that learn from historical data may inadvertently perpetuate biases present within that data, leading to unethical outcomes in property valuations.
For real estate firms utilizing data analytics wisely, collaboration with legal and compliance teams becomes essential to navigate complexities effectively. Balancing innovation with responsibility is crucial for sustainable growth in this data-centric landscape.
Conclusion
The integration of data science into modern real estate valuation methods significantly enhances the accuracy, reliability, and efficiency of appraisals. By employing sophisticated techniques such as machine learning, predictive modeling, and AVMs, investors and professionals alike can glean insights that were once beyond reach. The art of evaluating properties is evolving into a science, reshaped by data-driven methodologies that account for complex market dynamics.
Nonetheless, embracing technology comes with responsibilities, particularly regarding data quality, skill development, and ethical practices. As the industry moves forward, establishing a solid foundation of reliable data and skilled professionals will be imperative to fully leverage the potential of data science in real estate valuation. Thus, companies willing to invest in people, technology, and data governance will likely lead in this ever-evolving and competitive landscape—ensuring sustainable success in the intricate world of real estate.
If you want to read more articles similar to The Role of Data Science in Modern Real Estate Valuation Methods, you can visit the Real Estate Valuation category.
You Must Read