
User Behavior Analysis for Effective Media Recommendation Systems
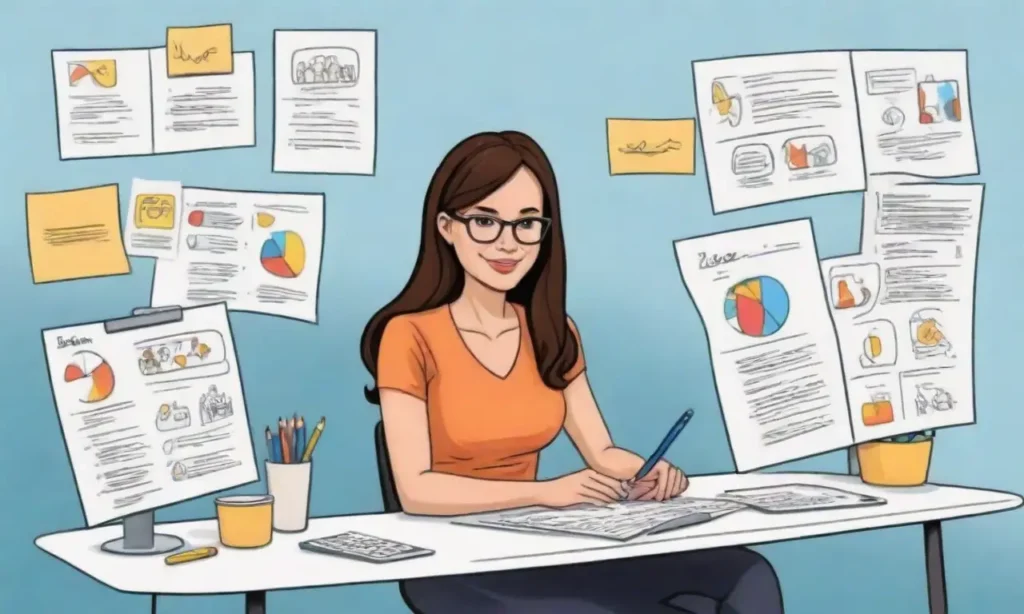
Introduction
In today's digital age, the sheer volume of media content available to consumers can be overwhelming. Whether it's streaming services, online news platforms, or social media, users are bombarded with options that can lead to decision fatigue. To make sense of this vast ocean of content, media recommendation systems have emerged as fundamental tools designed to tailor suggestions to each user's preferences and behaviors. By harnessing user behavior analysis, these systems can effectively predict what a user might enjoy next, thereby enhancing customer satisfaction and retention.
This article delves into the intricacies of user behavior analysis, focusing on its significance, methodologies, and impact on media recommendation systems. We will explore how understanding user actions—ranging from clicks to ratings—can illuminate the path towards developing more accurate, personalized, and engaging recommendations. By the end, readers will have a comprehensive understanding of how user behavior informs these systems and the best practices for leveraging this data effectively.
The Importance of User Behavior Analysis
Understanding user behavior is crucial for media recommendation systems because it provides insights into individual preferences and habits. When users interact with content, be it through watching videos, reading articles, or listening to music, a wealth of information is generated. Each click, like, share, or watch reflects user intent, preferences, and even evolving tastes over time. This data can be vital for crafting personalized experiences that resonate with users on a deeper level.
Moreover, user behavior analysis contributes to the overall business performance of media platforms. By providing tailored recommendations, platforms can manage to keep users engaged for longer periods, thereby increasing retention rates and reducing churn. When customers feel that a service understands them and caters to their specific interests, they are more likely to remain loyal and return frequently. Such loyalty not only boosts revenues but also enhances the reputation of the platform, leading to organic growth through word-of-mouth referrals.
The Role of Feedback Loops in Enhancing Recommendation SystemsUser behavior analysis also plays a pivotal role in the design and optimization of algorithms within recommendation systems. By analyzing user interactions, data scientists and engineers can tweak and improve their recommendation algorithms to ensure they are as effective and accurate as possible. Understanding the nuances of user behavior allows developers to create more nuanced models that can adapt to a variety of factors, such as changes in user behavior over time, market trends, and even seasonal shifts in content popularity.
Techniques for Analyzing User Behavior
Data Collection Methods
Analyzing user behavior requires systematic data collection methods that capture a broad spectrum of interactions. Modern media platforms typically use various techniques such as clickstream analysis, user ratings, and feedback loops to gather data. Clickstream data includes timestamps, page views, and the sequence of interactions a user has with the platform. This information can reveal patterns in browsing behaviors and consumption habits, providing a backbone for more in-depth analysis.
In addition to clickstream data, user ratings serve as another valuable source of information. When users rate content—be it movies, articles, or playlists—they are indirectly communicating their preferences. These ratings can help build user profiles that reflect tastes and desires, which can then be compared against other users to identify similar interests. For many media platforms, this collaborative filtering process is essential in creating a recommendation system that feels intuitive and personalized.
Another effective data collection method is the implementation of feedback loops. By actively soliciting user feedback through surveys or usability tests, platforms can gather qualitative data that adds context to quantitative metrics. Understanding why users like or dislike particular recommendations adds a qualitative dimension that can refine algorithms further. Thus, integrating feedback from users into the data collection process creates a more holistic view of user behavior that optimizes media recommendations.
How to Measure Success in Music Recommendation Systems EffectivelyBehavioral Analytics Tools
Once user behavior data is collected, behavioral analytics tools come into play to extract meaningful insights. These tools help identify trends, preferences, and anomalies in user behavior, which are crucial for refining recommendation algorithms. Various platforms and software solutions are available, ranging from simple analytics dashboards to sophisticated machine learning frameworks.
One popular tool for behavioral analytics is Google Analytics, which, though primarily used for websites, can provide valuable insights into user engagement and behavior on media platforms. By tracking various metrics such as time spent on page, bounce rates, and user demographics, media platforms can develop a clearer picture of how users interact with their content. Such insights often lead to better-targeted content delivery and improved overall user experiences.
Additionally, platforms may turn to machine learning algorithms for deeper analysis. These algorithms can sift through vast amounts of user data in real time and derive predictive insights based on observed patterns. Techniques like clustering algorithms can segment users into different categories based on similar behavior, while classification algorithms can predict the likelihood of a user enjoying specific content. Such advanced methodologies not only enhance the accuracy of recommendations but also allow media companies to respond agilely to changing trends and user preferences.
Evaluating Behavioral Insights
Collecting user behavior data and employing analytics tools is only one side of the coin; the other involves the evaluation of insights derived from this analysis. Metrics such as engagement rates, conversion rates, and user retention provide a quantitative measure of the effectiveness of recommendations generated by the system. A media recommendation system can only be deemed successful if it can demonstrate a positive correlation between personalized recommendations and user satisfaction.
Ethical Considerations in Music and Video Recommendation AlgorithmsAdditionally, A/B testing plays a pivotal role in evaluating behavioral insights. By presenting different user segments with varying recommendations or experiences, platforms can compare outcomes to determine which approach yields better results. This practice not only fine-tunes the recommendation process but also helps in identifying areas for improvement within the overall user experience.
Engaging users in an ongoing manner is also a crucial evaluative measure. Gathering real-time feedback through ratings and interactive surveys allows platforms to continuously refine their recommendation algorithms based on user satisfaction. For instance, if a significant number of users indicate a dislike for particular content, the system may need to recalibrate its understanding of that user's interests. An adaptive recommendation system that evolves with its user base is likely to enjoy sustained success.
Challenges in User Behavior Analysis

Data Privacy Concerns
As the demand for personalized media recommendations grows, so too do concerns surrounding data privacy. Users are becoming increasingly aware of how their data is collected, stored, and used. Consequently, compliance with regulations such as the General Data Protection Regulation (GDPR) has become a significant concern for media companies. Striking a balance between leveraging user data for personalized recommendations while respecting privacy rights is a complex challenge.
Understanding Contextual Bandits in Music Recommendation StrategiesCompanies must prioritize transparency in data collection and use. Providing users with clear information about data practices and giving them options to manage their privacy settings can build trust and improve user satisfaction. Implementing consent-based data collection processes allows users to feel more in control and engaged with the service, fostering a relationship built on trust.
Moreover, adopting privacy-first frameworks, such as anonymization and aggregation of user data, helps mitigate risks associated with data breaches and misuse. By providing significant value while ensuring users' privacy is respected, media platforms can develop effective recommendation systems without compromising ethical standards.
Data Overload and Noise
Another challenge in user behavior analysis stems from the data overload phenomenon. With the vast amounts of data generated by users, it can be easy for analysts to become overwhelmed or lose sight of the most pertinent information. Not all data points are equally useful; some may be irrelevant or misleading, also known as "noise." Identifying and filtering out unnecessary data points requires a strategic approach.
Employing effective filtering techniques and selecting meaningful metrics can aid in managing data overload. Analysts should focus on the key indicators that directly correlate with user engagement and satisfaction. Data visualization tools can also help distill complex datasets into manageable insights, making it easier for decision-makers to understand patterns and trends.
Artificial Intelligence in Music Recommendation: Myths and FactsFurthermore, using advanced techniques such as anomaly detection can help filter out noise. By identifying unexpected user behavior, companies can gain insights into potential issues or opportunities for improvement. Insights derived from noise can sometimes reveal hidden trends, allowing for nuanced recommendations that set media platforms apart from their competitors.
Adapting to Evolving User Preferences
User preferences are not static; they evolve over time in response to new trends, changing interests, and external influences. Adapting to this dynamic nature of user behavior presents its own set of challenges. Recommendation systems that rely solely on historical user behavior may quickly become obsolete if they do not incorporate the capacity to learn and adapt in real time.
To address this issue, implementing dynamic models is crucial. These models utilize real-time data to continually refine recommendations. Techniques such as real-time collaborative filtering, which updates user profiles based on the latest interactions, create a more agile system that can keep pace with rapid changes in user preferences.
Additionally, engaging in ongoing user research and keeping an eye on broader market trends can provide companies with the foresight needed to stay ahead of the curve. Exploring trends in user behavior on social media platforms, for example, can inform media platforms about potential shifts in interests, allowing them to adjust recommendations accordingly. Proactively adapting to evolving user preferences not only improves user satisfaction but can also foster innovation and growth within media recommendation systems.
Incorporating User Feedback Loops in Music Recommendation SystemsConclusion
User behavior analysis is an indispensable component of developing effective media recommendation systems. By deeply understanding users' interactions with content, businesses can glean valuable insights that foster personalized experiences. This approach leads to improved user engagement, satisfaction, and retention, all of which are pivotal in a competitive media landscape.
Despite the various challenges that come with analyzing user behavior—ranging from data privacy concerns to managing data overload and evolving preferences—media companies can navigate these hurdles through strategic data collection methods, employing robust analytics tools, and promoting transparency. Establishing a solid foundation of user trust through ethical practices is paramount in building sustainable relationships that allow for tailored recommendations.
Ultimately, the future of media recommendation systems lies in their ability to harness user behavior analysis while continuously adapting to evolving preferences and trends. Platforms that succeed in marrying effective data use with respect for user privacy will likely be at the forefront of enhanced user experiences, paving the way for innovation and a deeper connection between users and media content. Striking the right balance will not only ensure user satisfaction but can significantly impact a media platform's long-term success.
If you want to read more articles similar to User Behavior Analysis for Effective Media Recommendation Systems, you can visit the Music and Video Recommendation category.
You Must Read