
Cross-Industry Applications of Machine Learning in Cyber Defense
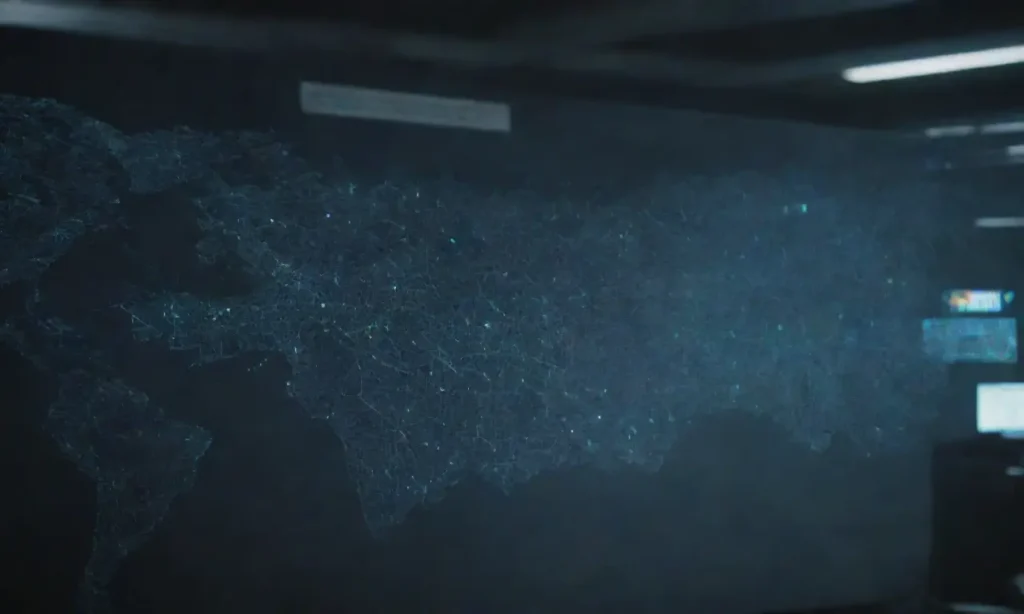
Content
Introduction
In today’s rapidly evolving technological landscape, cybersecurity has become a paramount concern for organizations across various industries. The increasing complexity and sophistication of cyber threats have necessitated the adoption of innovative solutions to safeguard sensitive data and systems. One of the most promising advancements in this arena is machine learning (ML), a subset of artificial intelligence that enables systems to learn from data and improve their performance over time. ML has emerged as a powerful tool in combating cyber threats by enhancing threat detection, automating response mechanisms, and improving overall cybersecurity strategies.
This article aims to provide a comprehensive exploration of the cross-industry applications of machine learning in cyber defense. We will delve into how different sectors, including finance, healthcare, manufacturing, and retail, are leveraging machine learning to bolster their cybersecurity frameworks. Additionally, we will outline the challenges these industries face, the solutions provided by machine learning, and the future potential of this technology in enhancing cyber defense.
Machine Learning Fundamentals and Its Relevance to Cybersecurity
To understand the applications of machine learning in cyber defense, it's crucial to grasp the fundamental concepts of machine learning. Essentially, machine learning involves algorithms and statistical models that enable computers to perform tasks without explicit instructions, relying instead on patterns and inference from data. This is particularly relevant in cybersecurity, where vast amounts of data are generated daily, making manual analysis impractical.
Machine learning can generally be classified into three categories: supervised learning, unsupervised learning, and reinforcement learning. In cybersecurity, supervised learning is often employed for tasks such as anomaly detection and malware classification. By training algorithms on historical data that includes labeled information about known threats, ML models can predict potential vulnerabilities in real-time systems.
Improving Cybersecurity Solutions with Advanced Machine Learning ToolsUnsupervised learning, on the other hand, is used to detect patterns in data where labels might not be available. This is crucial for identifying new or evolved threats that have not yet been categorized. Finally, reinforcement learning involves algorithms that improve their strategies over time based on feedback from their environment, a method that can be applied in adaptive security measures to counteract cyber attacks dynamically.
Applications in the Financial Sector
The financial sector is one of the most targeted industries for cyber attacks, often due to the sensitive nature of the data involved and the high potential for financial gain for attackers. Machine learning is being utilized in numerous ways to enhance cybersecurity in this domain. For instance, fraud detection systems leverage machine learning algorithms to analyze transaction patterns and flag potentially fraudulent activities. By continuously learning from new data, these systems can adapt to evolving fraud tactics and minimize false positives.
Moreover, machine learning models are increasingly employed in anti-money laundering (AML) efforts within banks. Traditional rule-based systems often struggle to identify complex laundering schemes; however, ML can uncover subtle patterns and relationships in transactional data that may indicate suspicious activities. By utilizing natural language processing (NLP) alongside machine learning, financial institutions can also analyze unstructured data from various sources, such as news articles and social media, to identify emerging threats and trends.
Furthermore, machine learning aids in enhancing identity verification processes. Biometric data, such as facial recognition and fingerprint scanning, can be analyzed using ML algorithms to determine the authenticity of users. As a result, ML significantly mitigates the risk of identity theft and unauthorized access to sensitive financial information.
Healthcare Sector Security Enhancements
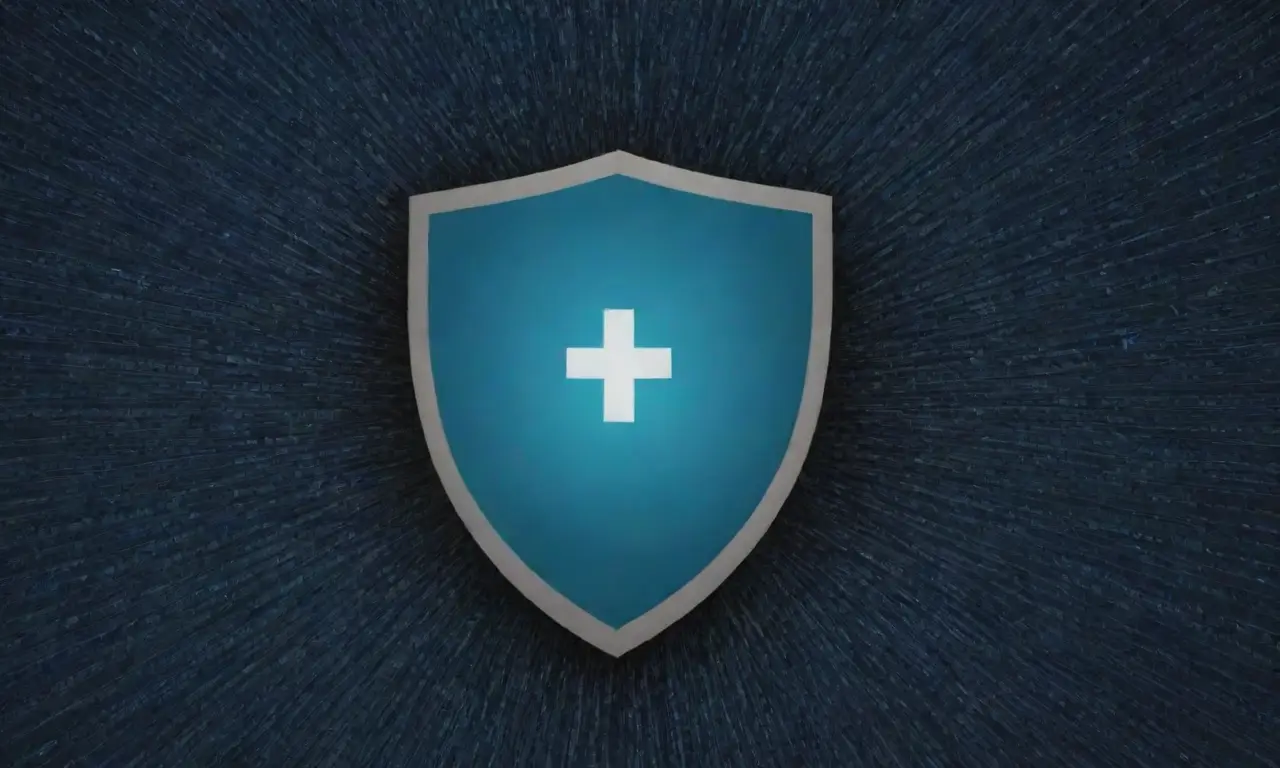
The healthcare sector is another critical area where machine learning is being strategically applied to enhance cybersecurity. The storage of large volumes of sensitive patient data makes healthcare organizations prime targets for cyber attacks. Machine learning can play a pivotal role in protecting this information by improving endpoint security.
For example, ML algorithms can analyze the behavior of devices connected to the network, identifying normal operating patterns and detecting anomalies that may signify a breach. This proactive approach allows security personnel to respond swiftly to potential intrusions before significant damage occurs.
Additionally, in the context of electronic health records (EHR), machine learning assists in safeguarding against data breaches by employing advanced encryption techniques and risk assessments. ML can continuously evaluate the security posture of EHR systems, alerting administrators to vulnerabilities and ensuring compliance with regulatory standards such as HIPAA.
The application of machine learning extends further to the monitoring of telemedicine platforms, which have surged in popularity due to the COVID-19 pandemic. Ensuring the security of remote interactions between healthcare providers and patients is critical. ML can enhance the security of these sessions by detecting unusual access patterns or unauthorized data sharing, helping maintain patient confidentiality and trust.
Cyber Defense in Manufacturing
The manufacturing industry is witnessing a significant transformation due to Industry 4.0, which integrates advanced technologies such as IoT (Internet of Things), AI, and machine learning. However, with this transition comes increased vulnerability to cyber threats. The integration of machine learning into Industrial Control Systems (ICS) has proved advantageous in enhancing security.
One of the key applications is in the realm of predictive maintenance. By employing machine learning algorithms to analyze data from machinery and equipment, manufacturers can identify patterns indicating potential malfunctions or security breaches. This enables timely interventions before issues escalate into serious problems or cyber incidents.
Moreover, ML models enable the detection of threats in real-time by continuously analyzing network traffic and user behavior. For instance, intrusion detection systems (IDS) backed by machine learning can identify unusual patterns indicative of a potential attack, such as unauthorized access to sensitive manufacturing data or disruption of critical operations.
The implementation of machine learning in the manufacturing sector also encompasses supply chain security. By analyzing data flows across the supply chain, organizations can identify potential points of failure or vulnerabilities that may be exploited by cybercriminals. This enables manufacturers to bolster their cyber defenses throughout the entire supply chain, from raw material procurement to final product delivery.
Retail and E-commerce Security Applications
The retail and e-commerce sectors are increasingly leveraging machine learning technologies to enhance cybersecurity measures against an array of cyber threats, including data breaches, payment fraud, and identity theft. With the increasing reliance on digital transactions, protecting consumer data has become an absolute priority for retailers.
One of the most essential applications of machine learning in retail is in fraud detection and prevention systems. Retailers utilize ML algorithms to analyze transaction data, flagging outliers that may suggest fraudulent activities. This proactive approach not only protects the business's financial interests but also enhances customer trust and loyalty.
Furthermore, machine learning can augment customer authentication processes. Retailers are implementing advanced authentication techniques, such as behavioral biometrics, which assess users’ online behaviors, passwords, and other identifiable factors to determine authenticity. Tying these factors together with machine learning can provide more robust security measures against credential stuffing attacks where criminals use stolen usernames and passwords to gain access to customer accounts.
Lastly, machine learning can also improve the security of point-of-sale (POS) systems. With cybercriminals increasingly targeting POS terminals to steal payment card information, ML can help detect and respond to atypical network traffic that may suggest a security breach. By monitoring system activity in real-time, machine learning tools can enable retailers to respond automatically to threats, shutting down systems or alerting personnel before significant damage occurs.
Conclusion
Machine learning is proving to be a game changer in the realm of cyber defense, offering diverse applications across various industries, including finance, healthcare, manufacturing, and retail. Its ability to analyze vast amounts of data, detect anomalies, and predict potential threats in real-time enhances an organization's overall cybersecurity posture. As cyber threats continue to evolve and become more sophisticated, the importance of integrating machine learning into cybersecurity strategies cannot be overstated.
However, organizations must remain vigilant and be aware of the challenges associated with machine learning, including data privacy concerns, the need for continuous training and updates of models, and the potential for bias in algorithms. Striking a balance between innovation and security will be crucial for the successful implementation of ML technologies in the fight against cyber threats.
Looking ahead, the potential for advancements in machine learning in cybersecurity is immense. As new technologies emerge and more data becomes available, the capability of machine learning to adapt and enhance security measures will continue to grow. Ultimately, by harnessing the power of machine learning, organizations can better protect themselves against the ever-evolving landscape of cyber threats, ensuring the safety and integrity of their systems and data for years to come.
If you want to read more articles similar to Cross-Industry Applications of Machine Learning in Cyber Defense, you can visit the Cybersecurity Measures category.
You Must Read