
Actionable Steps for Deploying ML in Customer Service Teams
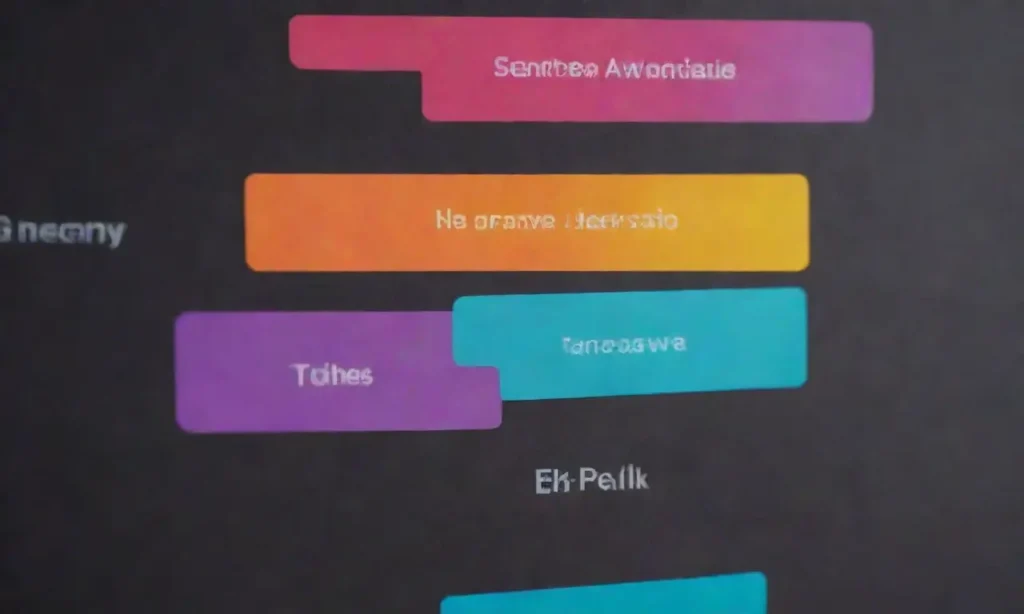
Content
Introduction
In today's fast-paced digital world, customer service plays a critical role in maintaining and enhancing customer relationships. As businesses strive for excellence in their service departments, Machine Learning (ML) emerges as a powerful tool to transform customer interactions. ML not only automates processes but also enables organizations to analyze vast amounts of data to deliver personalized experiences. This article explores actionable steps for deploying ML within customer service teams, ensuring they leverage the technology effectively to streamline operations and enhance customer satisfaction.
Deploying ML in customer service isn’t merely about implementing sophisticated algorithms. It requires meticulous planning, understanding customer needs, and aligning technology with business objectives. This article will guide you through several comprehensive steps to successfully integrate ML into your customer service framework, focusing on practical insights and real-world applications. By the end, you should have a clear roadmap to harnessing ML for improved service delivery.
Understanding Customer Needs Through Data Analysis
One of the foundational steps to deploy ML in customer service is to understand customer needs through thorough data analysis. Effective ML applications stem from a detailed comprehension of customer behavior and preferences, which can significantly enhance the relevance of the insights generated.
1. Collect Customer Interaction Data
To begin, gather data from various customer interactions across multiple channels—be it emails, chats, social media, or phone calls. Each touchpoint generates valuable insights. Utilize tools like Customer Relationship Management (CRM) systems to centralize this data, ensuring it can be seamlessly accessed and analyzed. The more data you collect, the richer the input for your ML models. However, it’s essential to ensure this data is clean and consistent to avoid skewing results.
Boosting Customer Engagement through ML-Powered Chatbots2. Analyze Feedback and Behavior Patterns
Next, analyze the collected data to identify feedback and behavior patterns that indicate customer preferences, common pain points, and service trends. Natural Language Processing (NLP) techniques can be employed here to process text data from customer feedback and categorize sentiments, helping you to gauge the general mood of your customer base toward your products and services. In addition, look for frequent topics or issues customers discuss—this provides valuable insight into what your team can improve upon or automate.
3. Prioritize Key Areas for ML Implementation
Once you understand the common themes within your data, prioritize key areas to apply ML solutions. Focus on problems that cause significant customer dissatisfaction or consume substantial staff resources. Common areas include automating responses to frequently asked questions, identifying potential churn through predictive analysis, and personalizing recommendations based on past purchases. By focusing on high-impact areas first, you can generate significant improvements relatively quickly.
Setting Clear Goals and Objectives
Before deploying ML solutions, it’s crucial to set clear goals and objectives. Having a well-defined framework allows your team to measure the effectiveness of implemented changes consistently.
1. Determine Specific Use Cases
Begin by determining specific use cases for your ML initiatives. For example, consider automating responses through chatbots, improving ticket resolution times through predictive analytics, or enabling personalized customer service based on previous interactions. Clearly articulating these use cases helps your team understand the overarching purpose behind the ML deployment, guiding development and integration efforts.
Integrating Machine Learning Tools with Existing Support Systems2. Define Success Metrics
Once use cases have been established, define success metrics that will help you evaluate the effectiveness of the efforts. Metrics might include customer satisfaction scores (CSAT), Net Promoter Scores (NPS), response times, and resolution rates. An explicit set of KPIs not only clarifies your objectives but also helps in tracking progress over time. Regular assessments and refinements based on these metrics will enable your team to pivot and adapt strategies when necessary.
3. Create a Timeline for Implementation
Establishing a timeline for implementation is another crucial component of setting clear goals. Break your deployment strategy into manageable phases with defined milestones. Each phase may include initial research, prototype development, testing, and full-scale deployment. Timelines create accountability within the team and ensure that the project remains on track. Regular check-ins at each milestone promote the necessary adjustments required to align with overall objectives.
Building a Cross-Functional Team
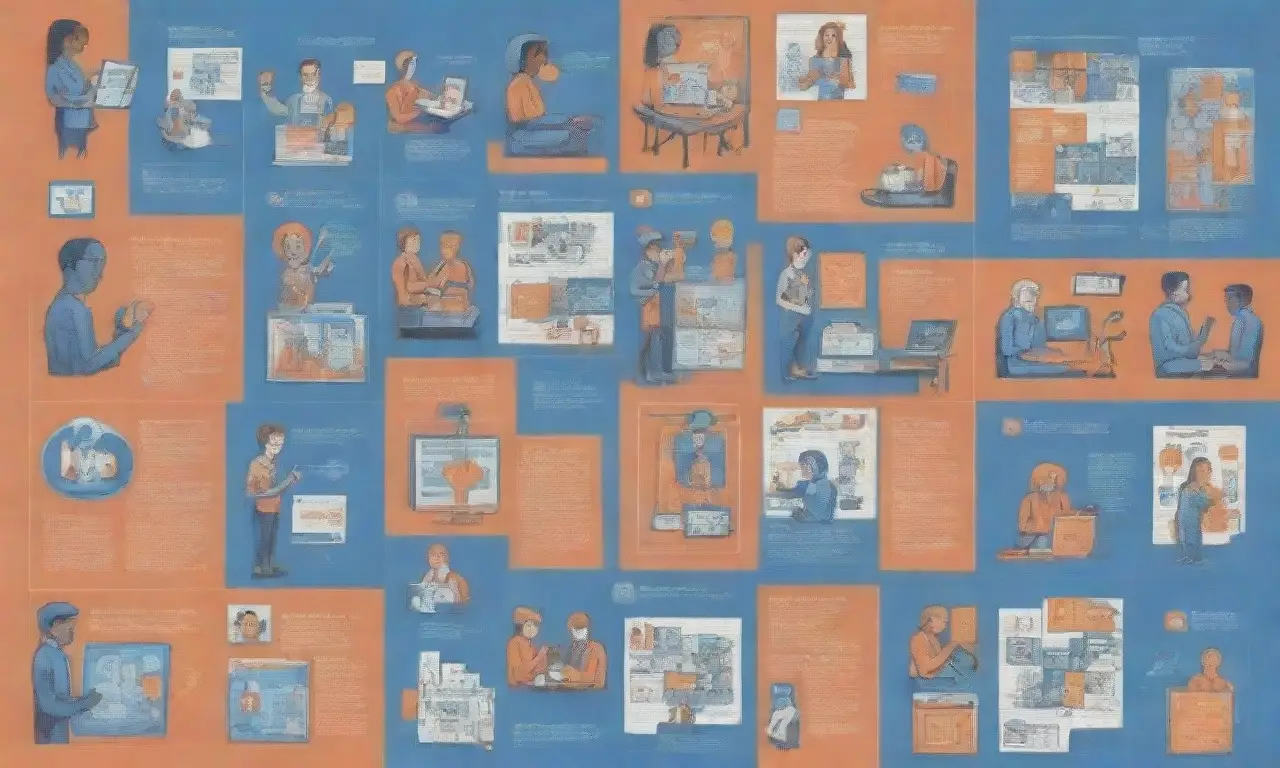
Deploying ML successfully in customer service activities requires a cross-functional team approach. Collaboration between various departments ensures that all necessary perspectives and expertise contribute to the project.
Exploring the Intersection of ML and Human Touch in Support1. Engage Stakeholders Across Departments
Engaging stakeholders from multiple departments—including customer service, IT, data science, and management—creates a more holistic perspective. Each department brings unique insights and expertise, allowing for better identification of potential challenges and solutions. Conducting workshops or brainstorming sessions can foster collaboration and help identify departmental needs that ML can address.
2. Foster Continuous Learning and Development
With technology rapidly evolving, investing in the continuous learning and development of your team is essential. Encourage team members to upskill through training sessions and workshops specifically focused on ML and data science concepts. This knowledge will not only empower your team but also foster a culture of innovation. Ensure your team stays updated on the latest trends and best practices in customer service and ML applications.
3. Establish Clear Roles and Responsibilities
To ensure efficiency, it’s vital to establish clear roles and responsibilities within the team. While diverse expertise is valuable, individuals should have defined areas of accountability to prevent overlap and confusion. For instance, designate a project manager to oversee the progress, a data analyst to interpret insights, and customer service representatives to provide essential feedback on customer interactions. This clarity in roles promotes focus and accountability, driving the project toward a successful outcome.
Implementing and Testing ML Solutions
After laying the groundwork, it’s time to implement and test your ML solutions. This phase is crucial for validating hypotheses and assessing operational effectiveness in real-world scenarios.
1. Develop Prototypes and Conduct Pilot Testing
Start with developing prototypes of your chosen ML models. For example, if you intend to deploy a chatbot, create an initial version and conduct pilot testing to evaluate its effectiveness. Pilot tests provide invaluable insights into user interactions and system usability. During this phase, gather real-time feedback from both customers and internal teams on functionality, accuracy, and overall quality.
2. Iterate Based on Feedback
One of the vital aspects of ML deployment is the ability to iterate based on feedback. Analyze the data gathered during testing, identifying areas for improvement or unexpected performance issues. This period of refinement increases the chances of successful implementation. Continually engage with customer service representatives who interact with the systems daily, as they can often provide the most relevant feedback from actual customer experiences.
3. Deploy Full-Scale after Validation
Once you are satisfied with the performance of the pilot tests and have made the necessary adjustments, prepare for full-scale deployment of your ML solutions. Ensure that adequate support and training materials are available to your customer service teams, allowing them to leverage the new tools effectively from day one. Communication with team members is key during this transition to foster an atmosphere of enthusiasm and acceptance for the new technology.
Ensuring Continuous Improvement
After deployment, the journey of ML integration doesn’t end; in fact, it is just the beginning. ML applications require ongoing evaluation and continuous improvement to adapt to evolving customer needs and technological advancements.
1. Monitor Performance and Gather Data
Establish a constant performance monitoring system to continuously evaluate the impact of your ML initiatives. Utilize analytical tools to visualize KPIs established in the earlier phases and assess system performance regarding customer satisfaction, operational efficiency, and overall effectiveness. Regular monitoring ensures that if potential issues arise, they can be addressed proactively before they escalate.
2. Stay Current with Technological Advancements
The field of ML is rapidly evolving, presenting new opportunities and technologies frequently. Encourage your team to remain current with technological advancements. This might include participating in industry webinars, subscribing to relevant publications, or networking with other professionals in the field. Understanding the latest trends allows your organization to adapt its customer service strategies continually, maintaining a competitive edge.
3. Foster a Feedback Culture
Creating a culture that encourages feedback at all levels is essential for continuous improvement. Soliciting feedback from both customers and employees can reveal valuable insights into the effectiveness of your ML solutions. Employ surveys, interviews, or focus groups to gather information regularly. Feedback mechanisms contribute not only to the enhancement of ML applications but also to broader service improvement initiatives.
Conclusion
Implementing Machine Learning in customer service teams is a transformative process that can significantly enhance operational efficiency and customer satisfaction. By taking actionable steps—understanding customer needs, setting clear goals, building a cross-functional team, implementing ML solutions, and ensuring continuous improvement—organizations can successfully leverage ML into their customer service strategies.
Ultimately, deploying ML is about creating a user-centric environment through data-driven insights. By prioritizing customer interactions and ensuring that technology aligns with business objectives, organizations can deliver more personalized, efficient, and responsive service experiences. Remember, the key to successful ML deployment lies in the interplay between technology, human expertise, and a commitment to continual evolution. It's an ongoing journey that requires investment, attention, and adaptability to achieve meaningful, long-lasting results.
If you want to read more articles similar to Actionable Steps for Deploying ML in Customer Service Teams, you can visit the Customer Support Automation category.
You Must Read