
Best Practices for Feature Engineering in Recommender Systems
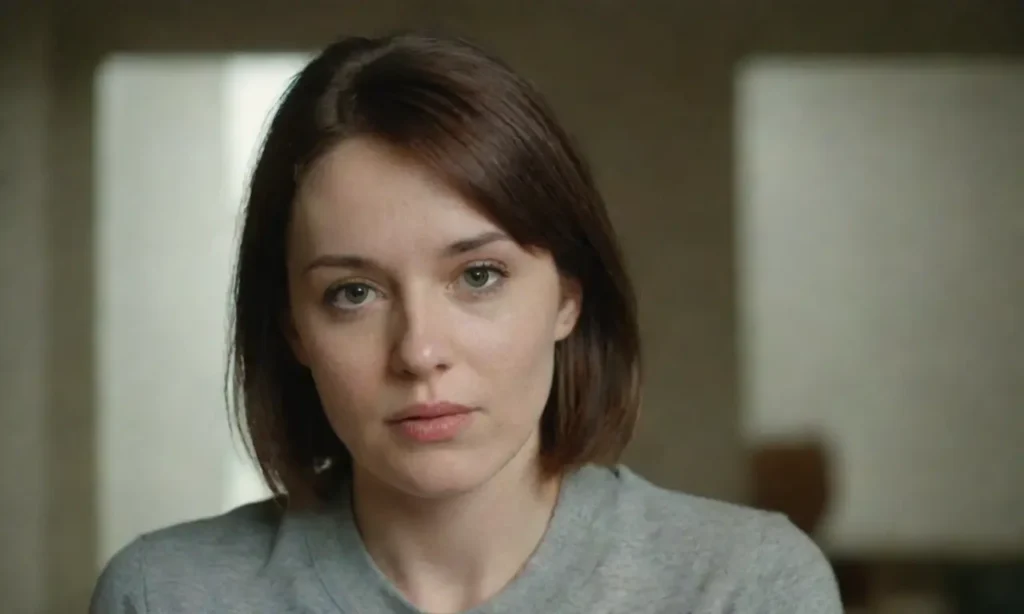
Introduction
Feature engineering is a critical step in the development of recommender systems, as it directly impacts their accuracy, efficiency, and ability to deliver personalized content. This process involves the selection, modification, and creation of features from raw data to improve the performance of machine learning models. With the rapidly growing volume of data and the diverse nature of user preferences, effective feature engineering has become indispensable for harnessing the full potential of recommendation algorithms.
In this article, we will delve into the best practices for feature engineering specifically designed for recommender systems. We will discuss the key types of features, techniques for extracting and transforming these features, and common pitfalls to avoid. By the end of this article, you will have a comprehensive understanding of how to effectively engineer features in order to create robust and user-friendly recommender systems.
Understanding the Types of Features in Recommender Systems
One of the first steps in feature engineering is identifying the various types of features that can be leveraged in a recommender system. Broadly speaking, features can be categorized into three types: user-based, item-based, and contextual features.
User-Based Features
User-based features focus on the characteristics and behaviors of the end-users. These can include demographics such as age, gender, and location, as well as behavioral aspects such as past interactions, purchase history, and preferences. For example, if you are building a movie recommendation system, knowing whether a user has a preference for action movies could help in customizing suggestions.
A Comprehensive Guide to Building a Book Recommendation SystemMoreover, user engagement metrics such as time spent on the platform, ratings given, and frequency of interactions can provide valuable insights into user preferences. Employing techniques like one-hot encoding for categorical variables and scaling continuous variables may enhance the model's learning capabilities. By accurately capturing user characteristics, you can create a more personalized experience that resonates with their individual tastes.
Item-Based Features
Item-based features categorize and describe the attributes of the items being recommended. In a book recommendation system, item features might include genre, author, publication year, and even user-generated content like reviews and ratings. Understanding the unique attributes of each item helps the system to make connections and recommendations effectively.
Another approach is to utilize content-based filtering, which focuses on the features and properties of the items themselves. For instance, if a user has shown interest in books by a particular author, the system can recommend additional books by the same author or in the same genre. The creation of item embeddings through techniques like natural language processing (NLP) can enhance recommendations by capturing semantic similarities among items.
Contextual Features
Contextual features relate to the circumstances surrounding the user and the items being recommended at a particular moment. Understanding the context can drastically improve recommendation accuracy. Contextual features can include time of day, current location, device type, and even seasonality.
How to Optimize Recommendations Using Reinforcement LearningFor example, a streaming service might want to recommend different content to users during the evening compared to daytime. By analyzing user behavior across different times or scenarios, you can fine-tune recommendations. Integrating context-aware features using techniques like contextual bandits or multi-armed bandit algorithms can enhance adaptability and improve the relevance of recommendations in real-time.
Techniques for Effective Feature Extraction
Once you identify relevant features, the next step is their extraction and transformation to optimize model performance. Several techniques can enhance your feature engineering process in recommender systems.
Data Preprocessing
Data preprocessing is essential for ensuring that the raw data is clean and ready for feature extraction. This typically involves handling missing values, removing noise and outliers, and transforming features into appropriate formats. For instance, if a user has not rated any items, this missing information must be accounted for rather than discarded to prevent skewing the results.
Normalization techniques such as Min-Max scaling or Z-score standardization can be employed to adjust the scales of numerical features so that they contribute equally to the model. Addressing these preprocessing steps ensures that the data quality is top-notch, laying a foundation for accurate feature extraction.
Implementing User-Based Collaborative Filtering in PythonFeature Combination and Interaction
Feature combination involves the synthesis of two or more features to create new, more predictive features. This can often improve model performance significantly. For example, combining user demographics with their interaction history can yield a new feature that provides insights into user behavior patterns.
Furthermore, considering feature interactions is crucial in recommender systems. Interaction terms can capture relationships between features that are not evident when analyzed individually. For instance, the effect of age on preferences may change when paired with the type of device a user utilizes. Modeling these interaction terms can be computationally intensive, but techniques like decision trees or neural networks can effectively uncover these hidden relationships.
Use of Automated Feature Engineering Tools
Another best practice is to leverage automated feature engineering tools to streamline the process. Libraries like Featuretools or Keras Tuner can help identify and generate potentially useful features without extensive manual effort. Automated tools can save time and ensure that no salient feature is overlooked.
These tools can also facilitate cross-validation and feature selection to identify the most effective features for your specific recommender system, thereby enhancing both efficiency and accuracy.
How Collaborative Filtering Enhances User Recommendations in AppsCommon Pitfalls to Avoid in Feature Engineering
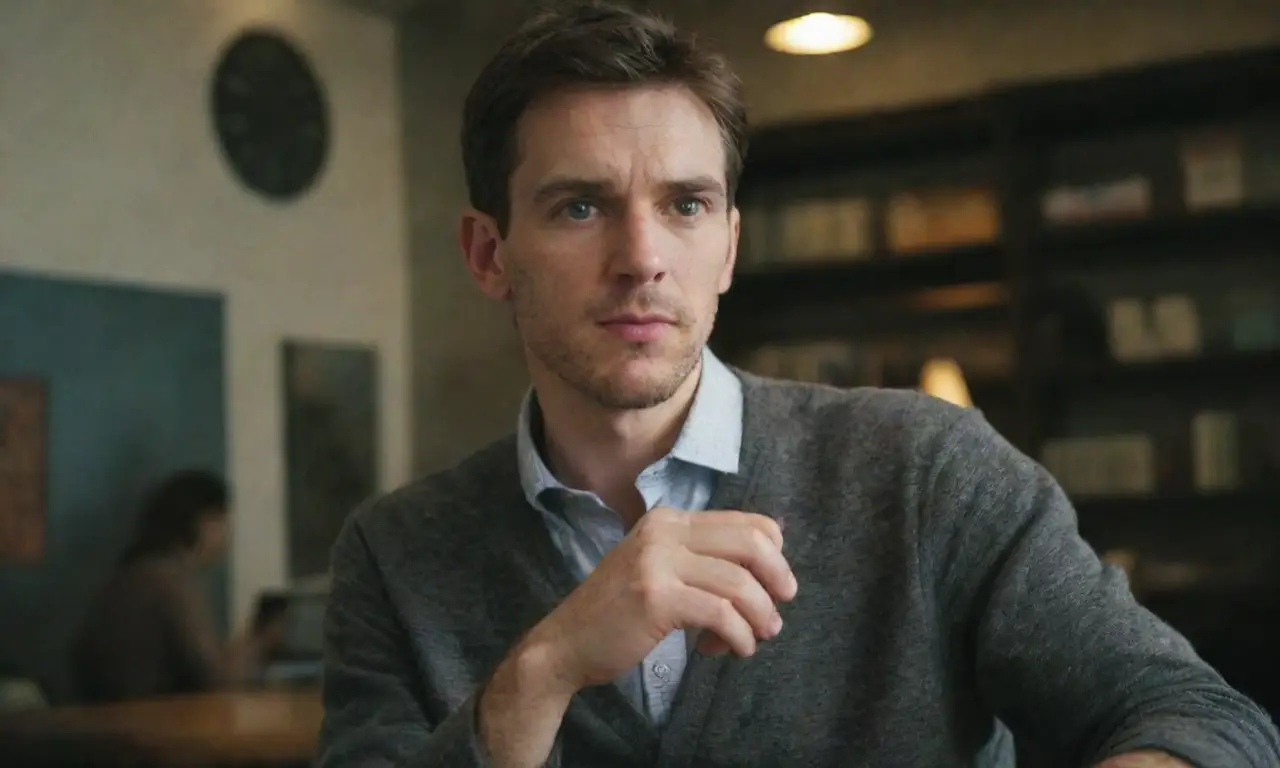
While feature engineering has great potential to enhance recommender systems, it is essential to be aware of common pitfalls that can hinder performance.
Overfitting Features
One of the most prevalent issues in feature engineering is overfitting. Overfitting occurs when a model learns noise from the training data instead of the underlying patterns. This typically happens when too many features are used, particularly when many of them are not informative.
To counteract overfitting, it is advisable to apply techniques such as L1 (Lasso) or L2 (Ridge) regularization, which impose penalties on the complexity of the model. Moreover, rigorous feature selection processes help identify and retain only those features that provide substantial predictive power without contributing to noise.
Cross-Domain Recommendation Systems: Sharing Knowledge Across DomainsIgnoring Domain Knowledge
Domain understanding plays a crucial role in effective feature engineering. Often, engineers fall into the trap of relying solely on automated tools and models without integrating their knowledge of the relevant domain.
For instance, certain user-item interactions may not be well-represented through traditional numerical features. Incorporating domain-specific knowledge can provide unique insights that strictly data-driven features may miss. Collaborating with domain experts can lead to the discovery of innovative feature combinations that better reflect real-world behaviors.
Failing to Incorporate Feedback Loops
In a dynamic environment like a recommender system, user preferences can evolve over time. Failing to create a feedback loop to monitor and adapt features based on user interactions will limit the system’s ability to remain relevant.
Incorporating user feedback into the recommendation algorithms, whether through explicit ratings or implicit signals like click-through rates, is essential. Implementing techniques such as reinforcement learning can help in continuously adapting the feature set to align with evolving user preferences, ensuring the recommender system remains dynamic and responsive.
Building User-Item Interactions: Techniques for Enhanced RecommendationsConclusion
In summary, feature engineering is a fundamental aspect of enhancing the performance of recommender systems. By understanding the types of features, utilizing effective extraction techniques, and avoiding common pitfalls, developers can significantly improve the quality and relevance of their recommendations.
The process of feature engineering is not static; it requires a continuous commitment to learning, adaptation, and refinement to keep pace with changing data and user preferences. As technologies evolve and new methodologies emerge, staying abreast of industry trends and research can provide the edge needed to optimize recommender systems.
Ultimately, an effective recommender system should not only aim for high accuracy but also prioritize creating a delightful user experience. Thoughtfully engineered features that resonate with users' needs will lead to enhanced satisfaction, engagement, and loyalty. By following best practices in feature engineering, developers can build systems that truly connect with users, driving their ongoing success in the competitive landscape of personalized recommendations.
If you want to read more articles similar to Best Practices for Feature Engineering in Recommender Systems, you can visit the Recommendation Systems category.
You Must Read