Real-Time Data Processing for Dynamic Customer Segmentation

Introduction
In an increasingly digital world, businesses are inundated with vast streams of data generated by customer interactions across various platforms. Real-time data processing has emerged as a critical capability, allowing businesses to interpret and act upon this information almost instantaneously. This capacity is particularly significant when it comes to dynamic customer segmentation—the practice of categorizing customers into groups based on real-time data to fine-tune marketing strategies, enhance customer experiences, and optimize business operations.
This article will delve deep into the world of real-time data processing and its implications for dynamic customer segmentation. We will explore the fundamental concepts surrounding these technologies, the tools and platforms that facilitate them, how businesses implement these strategies, and ultimately, the key benefits derived from such approaches.
Understanding Real-Time Data Processing
Real-time data processing refers to the capability to input, analyze, and output data almost instantly, providing organizations with the ability to react immediately to changing conditions. Unlike traditional data processing methods, which involve batch-processing data at scheduled intervals, real-time processing offers systems the ability to handle continuous streams of data. This involves utilizing technologies such as stream processing, in-memory computing, and event-driven architecture.
The Architecture of Real-Time Data Processing Systems
To comprehend real-time data processing, it’s crucial to understand its architecture, which typically includes various essential components:
Data Sources: This could be anything from social media feeds, website clicks, customer activity on apps, or even sensor data from IoT devices. These sources generate massive amounts of data requiring immediate attention.
Data Ingestion: A robust mechanism is necessary to pull data from various sources, commonly performed through Apache Kafka, Amazon Kinesis, or Apache Pulsar. These tools facilitate the continuous flow of data, handling high volumes and providing buffering mechanisms to ensure no data loss.
Real-Time Processing Engine: This is where the heavy lifting occurs, analyzing and processing the data as it flows into the system. Apache Flink, Apache Storm, and Spark Streaming are some tools that allow for complex event processing and immediate computation of incoming data.
Storage: Once data is processed, it is often necessary to store the data for long-term analysis. Solutions like NoSQL databases, such as MongoDB or Cassandra, are popular in this space due to their speed and flexibility in handling unstructured data.
Visualization Tools: Dashboards and real-time visualization tools (e.g., Grafana, Tableau, or Power BI) help stakeholders make sense of the incoming data by presenting it in a visually accessible format.
Importance of Real-Time Insights
Having real-time insights enables organizations to be agile and responsive. For instance, if a sudden spike in product interest is detected via social media monitoring, companies can respond by ramping up their advertising efforts or bolstering stock supplies. The real-time nature of data processing ensures businesses are making data-driven decisions based on the latest information rather than historical trends, which may no longer be relevant.
Swift insights can also lead to enhanced customer satisfaction. A telecommunications company might analyze customer call data in real-time, identifying patterns that indicate higher-than-usual call volumes. By proactively adjusting their customer support resources, they can decrease wait times and improve the overall customer experience.
Dynamic Customer Segmentation: A Game Changer
Dynamic customer segmentation is a process wherein businesses are able to categorize customers into distinct groups based on their behaviors, preferences, and purchasing activities as they happen. This contrasts with traditional segmentation methods, which often rely on periodic analyses of historical data, leading to potentially outdated profiles.
Methodologies for Dynamic Segmentation
Dynamic segmentation can draw from a variety of methodologies. Behavioral segmentation, for example, classifies users based on their interactions with a product or service. This could involve tracking website visits, clicks, and purchasing patterns, and segmenting customers into categories such as frequent buyers, one-time visitors, or bargain hunters.
Demographic analysis complements behavioral insights. For instance, age, gender, location, and spending patterns can be merged with behavioral data to form richer, more complex customer personas. This multidimensional approach potentially allows businesses to devise more tailored marketing efforts—ultimately enhancing customer satisfaction and brand loyalty.
Another effective segmentation methodology is predictive analytics, where past behaviors are analyzed alongside real-time data to forecast future customer actions. This anticipatory approach empowers organizations to craft tailored marketing messages, product suggestions, and promotions aligned with expected behaviors.
Tools and Technologies Supporting Dynamic Segmentation
Many tools and technologies enable dynamic customer segmentation, fostering real-time insights and actions. Customer Relationship Management (CRM) tools like Salesforce and HubSpot allow for the integration of real-time data analysis directly into existing customer platforms. These tools often come equipped with AI-driven algorithms that segment customers based on collected data.
Social media analytics platforms, such as Hootsuite and Sprout Social, also play a crucial role by allowing businesses to retrieve real-time data from social network interactions, providing insights for segmentation and targeted campaigns. More advanced offerings incorporate machine learning, enabling these platforms to refine segmentation continuously as they learn from ongoing interactions.
Additionally, companies are increasingly leveraging data lakes to store vast amounts of structured and unstructured data that is valuable for segmentation. This approach allows them to analyze data in real time without being confined to traditional data modeling or ETL (extract, transform, load) approaches.
Implementing Real-Time Dynamic Segmentation
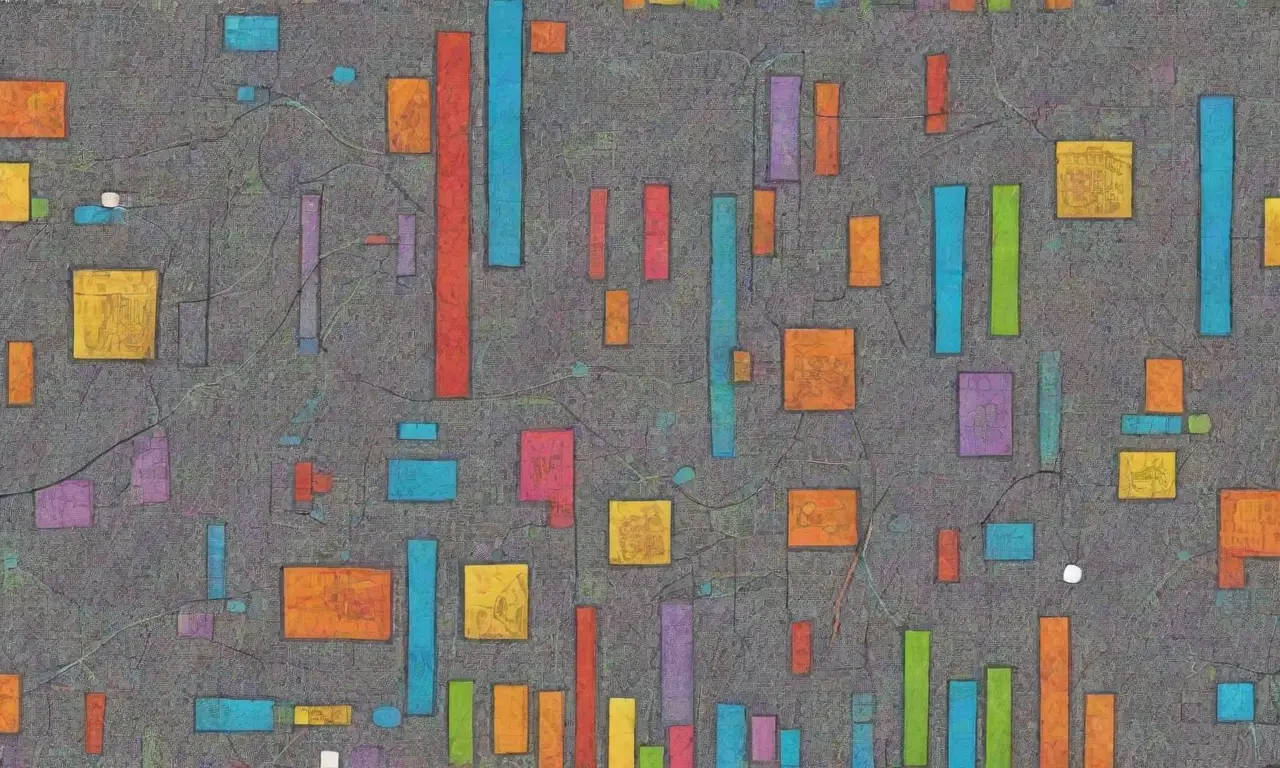
Implementing a real-time dynamic segmentation strategy requires careful planning and execution. Companies need to recognize their data sources first, identifying what information is available and relevant for segmentation. They must then develop the necessary infrastructure for effective real-time data processing, which includes integrating various software tools, storage solutions, and visualization platforms.
Data Collection Strategies
A robust data collection strategy is essential for successful implementation. Businesses should implement tracking mechanisms across all customer touchpoints, from websites to social media to offline interactions. Understanding customer interactions in a comprehensive manner may lead to better segmentation information. Using tools like Google Analytics, Mixpanel, or custom tracking solutions can summon crucial insights and enable ongoing data flow.
The Role of Cross-Channel Integration
For real-time segmentation to be truly effective, it is imperative that organizations adopt a cross-channel integration strategy. This not only allows for the collection of data across multiple platforms, but it also ensures that insights derived are consistent and comprehensive. Key systems like CRM and Marketing Automation platforms need to be effectively interconnected, so every segment of customer data correlates.
Continuous Monitoring and Adjustment
True to the name 'dynamic,' segmentation is not a one-time task but an ongoing process. Companies must continually monitor the performance of their segmentation strategies, checking the validity of their categories as customer behaviors evolve. By incorporating feedback loops, organizations can adapt their strategies based on the most recent data, ensuring they remain in sync with customer preferences.
Conclusion
In today’s fast-paced business landscape, the need for agile and effective marketing strategies is paramount. Real-time data processing enhances businesses' ability to respond swiftly to market changes, and when coupled with dynamic customer segmentation, it becomes a game-changing force. By utilizing real-time insights, organizations can not only target their marketing efforts more accurately but also develop a deeper understanding of their customer base.
The advantages of implementing real-time dynamic segmentation are multifaceted. Companies can enhance customer satisfaction through personalized experiences and targeted promotions, drive customer loyalty, and ultimately achieve higher conversion rates. Moreover, an effective implementation model broadens opportunities for long-term growth by fostering a culture that values data-driven decision-making.
As technology continues to advance, firms willing to invest in real-time data capabilities and dynamic segmentation methodologies will position themselves at the forefront of their industries. The future will undoubtedly favor those who can move faster, adapt more swiftly, and understand their customers more intimately. Therefore, organizations should not only keep pace with the evolving data landscape but strive to harness its power for sustained competitive advantage.
If you want to read more articles similar to Real-Time Data Processing for Dynamic Customer Segmentation, you can visit the Customer Segmentation category.