
Creating Omnichannel Marketing Strategies with ML Techniques
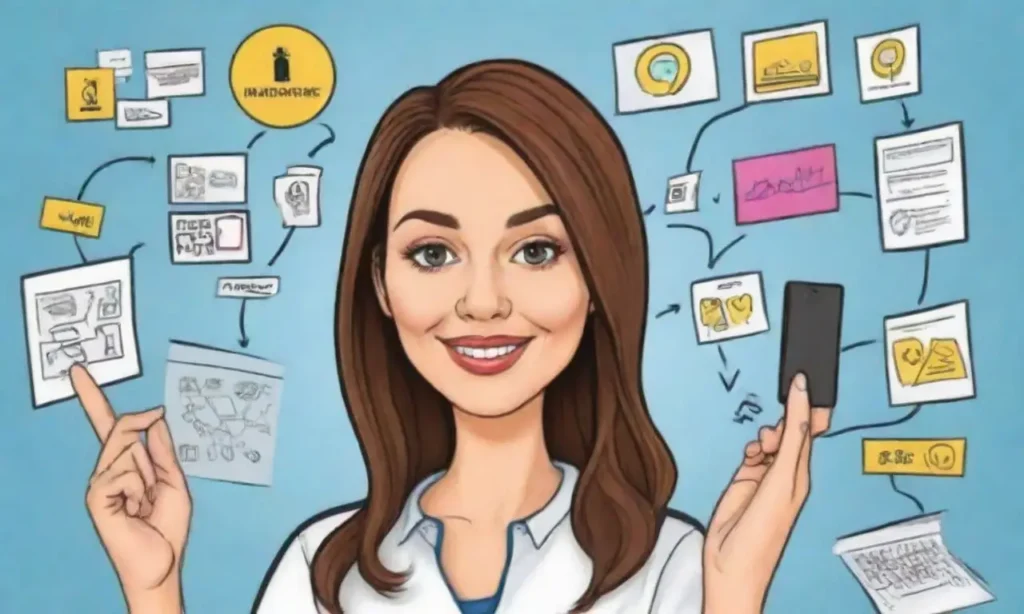
Introduction
In today's rapidly evolving digital landscape, the significance of omnichannel marketing cannot be overstated. As consumers move seamlessly across various platforms—be it social media, online retail stores, or physical locations— the traditional methods of marketing have become increasingly insufficient. Omnichannel marketing empowers businesses to deliver a cohesive, personalized experience that meets customers where they are, providing a seamless transition between all touchpoints.
This article delves into the intricacies of creating omnichannel marketing strategies enhanced by machine learning (ML) techniques. We will explore how ML can identify customer patterns, predict behaviors, and inform segmentation methodologies, ultimately driving a more effective omnichannel strategy. We aim to equip marketers with knowledge and practical insights that can be translated into actionable tactics to improve customer engagement and drive sales.
Understanding the Fundamentals of Omnichannel Marketing
Omnichannel marketing is a comprehensive approach that necessitates synchronization of customer experiences across diverse platforms — online and offline. Customers today expect consistency regardless of where they interact with a brand. This means if a customer receives an email promotion, sees a social media ad, and visits a physical store, those experiences should feel interconnected rather than disjointed.
The Customer Journey in an Omnichannel World
Understanding the customer journey is crucial for omnichannel marketing. The journey begins with awareness, progresses to consideration, and eventually leads to the purchase decision. Successfully navigating this journey requires brands to engage customers with relevant content at each stage, which is where ML techniques enter the equation.
By employing machine learning algorithms, marketers can analyze massive amounts of data to extract insights about customer behavior. This allows businesses to predict the next steps in the customer journey more accurately and personalize marketing messages accordingly. Essentially, ML serves as a guiding light through the complex maze of customer interactions, ensuring that every touchpoint enhances the customer experience.
The Role of Data in Omnichannel Marketing
Data is the cornerstone of effective omnichannel strategies. From transaction history to browsing behavior, customer data is the building block that informs segmentation, targeting, and personalization efforts. In the age of big data, organizations often find themselves inundated with information from various sources, making it challenging to derive actionable insights.
This is where machine learning shines. Leveraging advanced analytics, machine learning algorithms can sift through vast datasets to identify key patterns and trends. For instance, clustering algorithms can group customers based on similar preferences and behaviors, while regression models can predict future purchasing patterns. By automating this analysis, businesses can make real-time decisions and adapt their strategies to meet ever-changing consumer expectations.
Key Components of an Effective Omnichannel Strategy
Developing an effective omnichannel marketing strategy involves a blend of several critical components. Understanding these components will not only help businesses create a unified brand experience but also maximize the potential of ML techniques.
Customer Segmentation and Targeting
Effective customer segmentation allows organizations to tailor their marketing messages to specific audience groups. Traditional methods often rely on demographics, but machine learning brings a new dimension through predictive analytics.
Using algorithms like k-means clustering and decision trees, businesses can categorize customers according to a combination of factors such as purchasing behavior, online browsing patterns, and social media interactions. For instance, a fashion retail brand may discover distinct customer segments that exhibit unique shopping behaviors during specific seasons or in response to certain promotions.
By understanding these segments, brands can employ more targeted marketing efforts, sending personalized communications that resonate with individual preferences. Furthermore, ML models can continuously refine these segments in real-time, ensuring marketing efforts align with evolving consumer behaviors.
Personalization and Recommendations
Personalization is at the heart of successful omnichannel marketing. In a world where consumers expect tailored experiences, leveraging machine learning for personalized recommendations can significantly enhance customer satisfaction and loyalty.
By employing algorithms such as collaborative filtering and content-based filtering, marketers can make relevant product recommendations based on individual preferences and historical data. For example, an e-commerce site can use past purchase data to recommend complementary items, increasing the likelihood of an additional purchase.
Moreover, personalization extends beyond just product recommendations. Utilizing natural language processing (NLP), brands can analyze customer feedback and sentiment, ensuring that communications resonate based on tone, style, and context. This granular approach helps create an overall customized experience for customers across all channels.
Real-Time Analytics and Feedback Loops
With the pace of digital interactions accelerating, the ability to analyze data in real time is invaluable. Implementing feedback loops through machine learning allows businesses to measure campaign effectiveness instantly, adjust strategies, and respond to consumer needs promptly.
For example, through continuous tracking of customer interactions across all channels, marketers can utilize ML models to detect shifts in consumer sentiment or behavior almost immediately. If a marketing campaign underperforms, ML can help identify the underlying reasons, such as targeting the wrong audience or failing to resonate with customer needs.
This agile methodology creates a responsive and adaptable marketing strategy that aligns with the dynamic nature of customer preferences. The key to success lies in creating a loop of constant learning and adaptation through data-driven decision-making.
Implementing Machine Learning Techniques in Omnichannel Strategies

Integrating machine learning into omnichannel marketing strategies demands a clear understanding of organizational goals, data structures, and the technology stack. Below are crucial steps businesses can take to effectively implement ML techniques.
Building a Robust Data Infrastructure
Before implementing any machine learning techniques, it is essential to have a solid data infrastructure. This involves ensuring that the data collected from various sources—be it web analytics, CRM systems, or social media—is organized, clean, and ready for analysis.
Establishing a data warehouse or utilizing cloud-based solutions can facilitate the easy integration of data from multiple channels. Moreover, employing data governance tools ensures data quality and security, which is critical in the current regulatory environment surrounding data privacy.
Selecting the Right Algorithms
Once the data is organized, choosing the right algorithms becomes vital. The choice of ML algorithms will significantly impact the effectiveness of the omnichannel strategy. For instance, clustering algorithms are ideal for customer segmentation, while classification algorithms like random forests can be used to predict customer responses to marketing campaigns.
Additionally, employing ensemble methods can enhance model performance by combining multiple learning algorithms, thus improving predictive accuracy. it is also important to continuously evaluate and fine-tune the performance of these models to ensure they reflect current consumer trends.
Collaborative Learning and Team Alignment
Creating an omnichannel marketing strategy is not just the responsibility of marketing departments; it requires collaboration across various organizational teams, including sales, customer service, and IT. Engaging stakeholders from these areas will help develop a shared understanding of consumer insights driven by ML.
By aligning cross-functional teams on goals, objectives, and performance metrics, organizations can foster a collaborative environment that promotes innovation. Regular workshops or training sessions can ensure all teams understand how machine learning techniques can bolster their respective functions, leading to a unified brand experience for the customer.
Conclusion
Embracing omnichannel marketing strategies enhanced by machine learning techniques is no longer a luxury; it's become a necessity for businesses looking to thrive in today's competitive landscape. Organizations willing to invest in robust data infrastructure, leverage predictive analytics for customer segmentation, and foster collaboration across teams will undoubtedly stand out in delivering seamless and personalized customer experiences.
Moreover, the integration of machine learning not only drives efficiency in data handling and analysis but also empowers brands to anticipate consumer behaviors with remarkable accuracy. As customer expectations continue to evolve, marketers must remain agile, leveraging the power of ML to adapt their strategies.
In conclusion, creating effective omnichannel marketing strategies with machine learning techniques involves careful planning, collaboration, and execution. By adopting these practices, businesses can cultivate stronger customer relationships while significantly enhancing their market positioning. The future of marketing is omnichannel, and with the help of machine learning, brands are well-equipped to deliver experiences that truly resonate with their customers.
If you want to read more articles similar to Creating Omnichannel Marketing Strategies with ML Techniques, you can visit the Marketing Automation category.
You Must Read