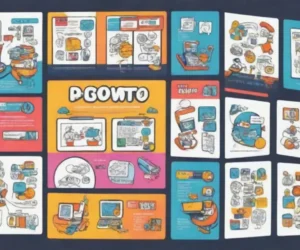
Real-World Applications of AI in Accelerating New Drug Approvals
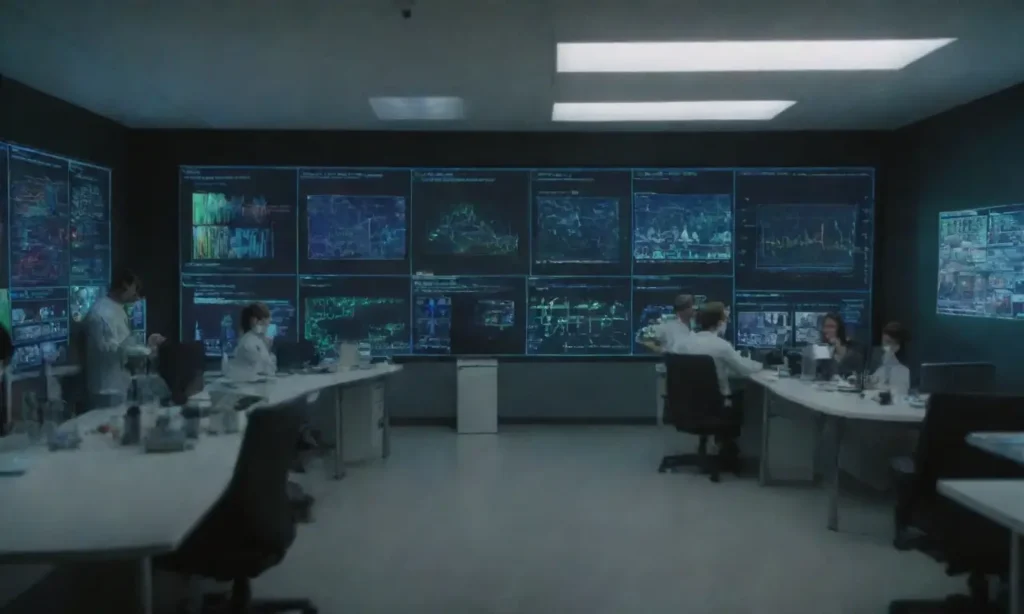
Introduction
The pharmaceutical industry has always been at the forefront of innovation, striving to develop new treatments and solutions for a myriad of health issues. However, the traditional process of drug development is often prolonged and costly, characterized by several phases of research, clinical trials, and regulatory hurdles. In recent years, the emergence of Artificial Intelligence (AI) has garnered significant attention for its potential to revolutionize how drugs are discovered and approved. By utilizing advanced algorithms and data analytics, AI technologies are streamlining processes, enhancing predictive modeling, and ultimately speeding up the time it takes to bring therapies from laboratory to market.
This article aims to explore the real-world applications of AI in the realm of drug approvals, shedding light on how organizations are harnessing these technologies to expedite the development timelines. By discussing the various methods and success stories, we hope to illustrate the transformative nature of AI in pharmaceuticals and underscore its role in addressing pressing healthcare needs.
The Role of AI in Drug Discovery and Development
AI's integration into drug discovery has fundamentally changed how researchers identify and validate new compounds. One primary application is in the analysis of vast datasets to discover potential drug candidates. Traditionally, this process required painstaking manual labor and was subject to human error. However, machine learning algorithms can sift through millions of data points, identifying trends and patterns that may not be immediately apparent to human analysts.
For instance, companies like Atomwise use AI to predict how different molecules will interact with specific proteins associated with various diseases. By leveraging deep learning techniques, Atomwise’s platform can rapidly assess numerous compounds and predict their effectiveness as potential therapeutics. Such AI-driven discoveries not only increase the likelihood of finding promising candidates but also reduce the overall time spent on hit identification in early drug development phases.
Using ML Algorithms for Predicting Side Effects in MedicationsMoreover, AI technologies can aid in optimizing the selection of clinical candidates. Through advanced computational methods, researchers can assess the toxicity, bioavailability, and pharmacokinetics of various compounds, allowing for a more informed decision on which candidates to advance. This not only minimizes the risk of failure during clinical trials but also conserves resources and time, expediting the overall drug approval timeline.
Enhancing Clinical Trials with AI
The traditional structure of clinical trials is often the rate-limiting step in drug approval processes. For studies to yield statistically significant conclusions, they must recruit a diverse and adequate sample size. AI can transform this aspect by enhancing participant recruitment strategies and streamlining trial design.
One significant AI application in clinical trials is in patient stratification. Machine learning models can analyze electronic health records (EHRs) to identify suitable candidates who meet specific inclusion criteria. Instead of relying solely on standard criteria, AI can unearth trends within patient data, allowing researchers to target participants more effectively. This technique not only accelerates patient recruitment but also increases the likelihood of better trial outcomes by ensuring a well-matched cohort.
Moreover, AI-powered tools can optimize clinical trial protocols. By analyzing prior trial data, AI can suggest modifications to dosing regimens or study endpoints that may enhance the likelihood of success. For instance, the FDA has recognized the potential of AI in adaptive trials, where modifications to the study are made while it is ongoing. This flexibility allows researchers to pivot based on interim results without delaying timelines or wasting resources.
Creating Robust Models for In Silico Drug Discovery ValidationThe ability to predict adverse events is another crucial role AI plays in clinical trials. Models trained on vast sets of historical trial data and patient outcomes can help forecast potential side effects of novel drugs. Such predictive capabilities enable researchers to implement risk mitigation strategies early in the process, providing a smoother path to regulatory approval.
AI in Post-Approval Monitoring and Pharmacovigilance

Once a drug is approved, the work does not end; ongoing monitoring of its safety and efficacy is essential to ensure public health. Pharmacovigilance practices are critical to identifying rare adverse drug reactions (ADRs) that may not have been evident during clinical trials. AI technologies offer significant advantages in this area, primarily through natural language processing (NLP).
AI-powered NLP algorithms can scan vast repositories of health data, including social media discussions, medical literature, and clinical reports, for mentions of drug-related incidents. By correlating this information with patient demographics and drug usage patterns, AI can facilitate the identification of signals that may warrant further investigation. This proactive approach enhances the detection of new safety concerns and informs regulatory actions, helping to protect patient safety.
The Role of Deep Learning in Predicting Drug-Target InteractionsFurthermore, AI can streamline the reporting of ADRs, improving the efficiency of adverse event reporting systems. Instead of relying on manual reports, which can be labor-intensive and slow, AI can automate data extraction and categorization. This capability not only allows for quicker responses to emerging safety issues but also ensures that regulatory bodies receive timely information about potential risks associated with medications already on the market.
AI's role in continuous monitoring also extends into predicting patient responses post-approval. By analyzing patient data post-therapy, AI models can anticipate which groups may experience issues based on genetic, demographic, or environmental factors. By identifying these populations, healthcare providers can tailor treatment plans and improve patient outcomes while reducing the impact of adverse events.
Case Studies: Success Stories of AI in Drug Approvals
Several pharmaceutical companies have successfully implemented AI technologies to accelerate drug approvals, providing compelling evidence of its impact. One notable example is DeepMind, a subsidiary of Alphabet Inc., which has made significant strides in solving complex biological problems using AI. Their research in protein folding, known as AlphaFold, demonstrated that AI could predict 3D structures of proteins with remarkable accuracy. This breakthrough can revolutionize the drug development landscape by enabling scientists to design drugs that precisely target these newly understood protein structures, leading to faster and more targeted therapeutics.
Similarly, GSK (GlaxoSmithKline) has partnered with AI companies to enhance their drug discovery processes. Their collaboration with Exscientia, an AI-driven drug discovery company, resulted in the rapid development of a drug candidate for mental health disorders. By employing predictive algorithms to analyze the biochemical properties and biological targets of compounds, they were able to advance a candidate into the clinic much faster than traditional methods would allow.
Pharmaceutical Data Mining: Using ML to Extract Valuable InsightsAnother successful application can be seen in Insilico Medicine. This biotechnology firm utilizes AI for various tasks, from drug discovery to personalized medicine solutions. Their AI platform designed a novel drug for fibrosis within just 46 days, showcasing an expedited timeline significantly shorter than conventional drug development timelines. This accomplishment emphasizes how effectively AI can transform processes that typically stretch over years or even decades.
Conclusion
The intersection of AI technology and pharmaceutical development holds tremendous potential to accelerate new drug approvals and enhance the overall efficacy of healthcare solutions. By optimizing each stage of the drug development process—from discovery to clinical trials and post-market monitoring—AI reduces timelines, lowers costs, and improves outcomes.
As the pharmaceutical industry continues to adapt to this rapidly changing environment, the embrace of AI-driven methodologies will undoubtedly lead to a future where effective treatments can reach patients faster. In addition to the tangible benefits of faster drug approvals, the incorporation of AI may also foster a culture of innovation, encouraging collaborations between tech startups, academic institutions, and large pharmaceutical companies.
Despite the challenges that lie ahead—such as regulatory considerations, ethical implications, and the need for robust data infrastructure—the impact of AI on drug development is already undeniable. As more organizations recognize the necessity of integrating AI into their workflows, we can anticipate a healthcare landscape that is not only more responsive to patient needs but also more capable of addressing the diseases and conditions that afflict populations worldwide.
Machine Learning for Biomarker Discovery in Cancer TherapeuticsIf you want to read more articles similar to Real-World Applications of AI in Accelerating New Drug Approvals, you can visit the Drug Discovery category.
You Must Read