
The Science Behind Emotion Recognition in AI-Generated Imagery
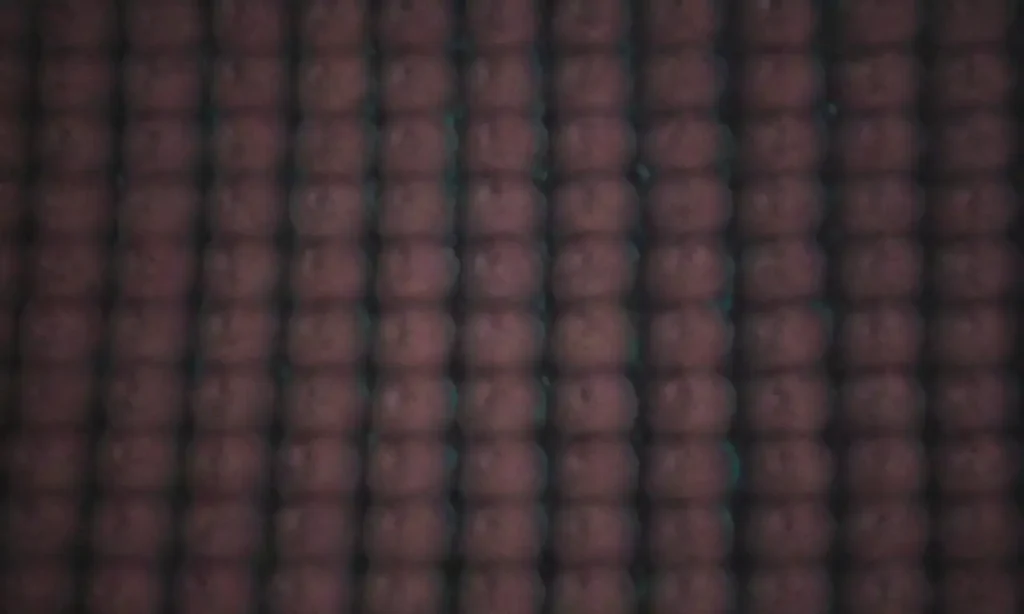
Introduction
In our fast-evolving digital world, artificial intelligence (AI) plays a pivotal role in numerous fields, including healthcare, social media, and entertainment. Among its many applications, one of the most fascinating is the ability of AI systems to recognize and interpret human emotions through various forms of imagery, whether it be photographs, videos, or even AI-generated art. This intersection of technology and psychology raises several questions about how machines perceive emotions and the implications of such abilities in our daily lives.
This article delves deep into the underpinnings of emotion recognition in AI-generated imagery, examining the technological frameworks, psychological theories, and ethical considerations that coexist with this incredible advancement. We will explore how AI algorithms detect emotional cues, the accuracy of such systems, and their potential applications and challenges in real-world scenarios.
Understanding Emotion Recognition
Emotion recognition refers to the ability of software systems to identify and interpret human emotions based on various inputs, predominantly visual stimuli. This process involves analyzing facial expressions, body language, and even physiological responses such as heart rate and skin conductance. The data science behind emotion recognition intertwines computer vision, machine learning, and psychological studies, creating a rich tapestry that reveals insights into human emotions.
At the core of emotion recognition systems lies computer vision—a field focused on enabling machines to interpret and make decisions based on visual data. Advanced algorithms are trained on vast datasets that contain images paired with labeled human emotions. By exposing the algorithm to countless instances of joy, surprise, anger, or sadness, the system learns to recognize and classify emotions through specific facial expressions and body postures.
The Impact of AI on the Future of Handmade Art and CraftsMachine learning models, particularly deep learning architectures such as convolutional neural networks (CNNs), are the backbone of these recognition systems. These networks excel in processing pixel data, enabling them to extract complex features from images. By fine-tuning these models with diverse datasets, researchers can enhance their accuracy, allowing machines to understand subtle emotions that even human observers might find challenging.
The Role of Psychological Theories
The Basic Emotions Theory
One of the most influential frameworks in understanding how emotions are expressed and interpreted is the Basic Emotions Theory proposed by psychologists such as Paul Ekman. According to this theory, certain emotions—such as happiness, sadness, anger, surprise, and disgust—are universal and can be recognized across different cultures. AI systems often use this conceptual framework to categorize emotions.
The Basic Emotions Theory posits that these emotions are linked to recognizable facial expressions, making them ideal for AI analysis. For example, a smiling face is often associated with happiness and joy, while a furrowed brow may indicate sadness or concern. AI algorithms rely on these universal cues to identify emotions in imagery effectively.
Moreover, understanding the cultural context of facial expressions is crucial for improving the accuracy of emotion recognition systems. Although many emotional expressions are universal, cultural nuances do exist; therefore, the inclusion of diverse datasets in training algorithms is essential to ensure that they work effectively across varying demographics and cultural backgrounds.
The Role of Latent Space in Generating Diverse Image OutcomesThe Dimensional Model of Emotion
Another prominent framework is the Dimensional Model of Emotion, which suggests that emotions can be understood on two axes: valence (the intrinsic attractiveness or averseness of an emotion) and arousal (the intensity of the emotion). This model enables a more nuanced analysis of emotional states beyond basic categories.
AI systems can utilize this dimensional approach to classify emotions along a spectrum rather than fitting them into discrete categories. This allows for greater flexibility in interpreting complex emotional states, which are more in line with human emotional experiences. For instance, someone might feel a mix of joy and anxiety during a happy event, such as a wedding.
By employing these psychological frameworks, AI can better generalize its understanding of emotions, ensuring that its analyses reflect the complexities of human emotional experiences rather than oversimplifying them into singular categories.
Techniques in Emotion Recognition
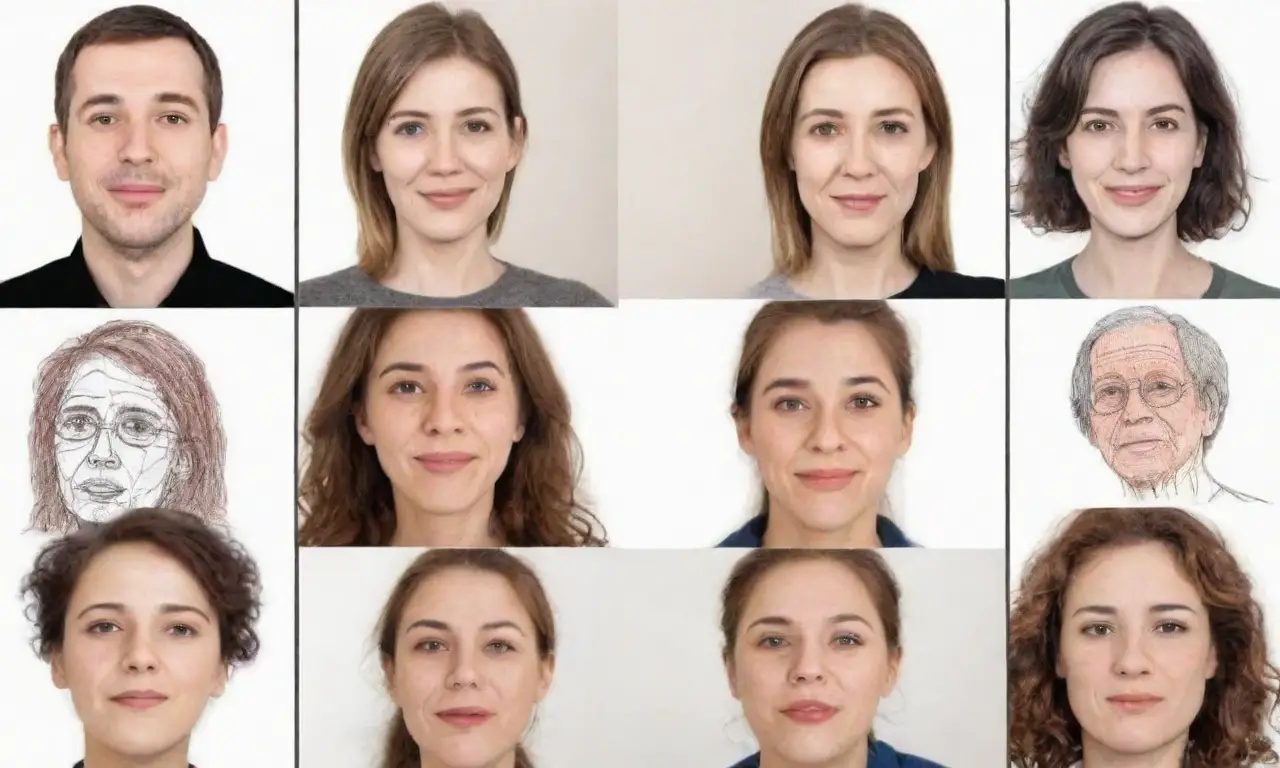
Facial Expression Analysis
One of the most common methods of emotion recognition in AI is through facial expression analysis. By using techniques from computer vision, AI algorithms can detect facial landmarks that correspond to different emotions. For example, the curvature of the mouth, the position of the eyebrows, and the openness of the eyes can provide valuable cues about a person's emotional state.
The analysis begins with the preprocessing of images to ensure consistency, involving tasks like resizing and normalization. Subsequently, the algorithm identifies key facial features and matches them to known expressions using pre-trained models. This is often followed by applying machine learning techniques to classify the detected expressions into corresponding emotional categories.
Body Language and Posture Analysis
Beyond the face, body language plays a significant role in conveying emotion. AI systems are increasingly incorporating pose estimation technologies to assess human emotion through posture and gestures. For example, crossed arms may indicate defensiveness or discomfort, while an open posture may signal confidence and openness.
By integrating data analytics techniques, AI can analyze videos frame by frame to understand movements and postures, contributing to a more holistic understanding of emotional states. This multidisciplinary approach, combining computer vision and behavioral analysis, allows for the development of systems that can gauge emotions with greater precision.
How Ethical Considerations Shape Image Generation Model DeploymentMultimodal Emotion Recognition
The latest advancements in emotion recognition focus on multimodal techniques, which utilize multiple sources of data to enhance accuracy. For instance, these systems may combine facial expression analysis, body language observation, and even auditory cues such as tone of voice or speech patterns.
By drawing upon various data modalities, these systems can create a more comprehensive understanding of emotion. For example, a person might express happiness verbally while simultaneously exhibiting a nervous body posture. A sophisticated AI could integrate these cues, yielding a more nuanced interpretation of the individual’s emotional state.
Applications of Emotion Recognition in AI
Healthcare
The applications of emotion recognition technologies are vast, particularly in the realm of healthcare. Emotion recognition AI can assist healthcare professionals in identifying mental health conditions, such as depression or anxiety, by analyzing patients’ emotional expressions during consultations. Early detection enables timely intervention, which can significantly improve outcomes for patients.
Moreover, AI systems can be utilized in therapeutic settings, where they can provide real-time feedback to patients undergoing emotional therapy. By recognizing emotions through virtual interactions, these systems can help individuals gain insight into their emotional states and develop coping strategies effectively.
Image Generation in Fashion: How AI Is Changing the IndustryMarketing and Customer Engagement
In the realm of marketing, companies are leveraging emotion recognition technologies to enhance customer engagement. By analyzing customers' emotions while interacting with a product or brand, businesses can tailor their marketing strategies more effectively. For instance, if an advertisement triggers positive emotions, marketers may focus on that specific aspect to draw in more customers.
Additionally, companies can use AI systems to gauge audience reactions during live events or ad campaigns, allowing them to make real-time adjustments to enhance engagement. This data-driven approach to marketing creates a more personalized experience for consumers, fostering brand loyalty.
Education
Emotion recognition AI is also finding its place in education, where it can help educators understand the emotional states of students, particularly in online learning environments. By detecting feelings of confusion or frustration, educators can adapt their teaching methods to meet students' needs more effectively.
Furthermore, AI can provide students with feedback on their emotional responses during learning activities, encouraging them to develop emotional intelligence and resilience. The integration of emotion recognition technology into educational settings can lead to a more supportive and effective learning experience, ultimately enhancing student outcomes.
A Comprehensive Guide to Style Transfer in Image Generation ModelsEthical Considerations and Future Directions
Privacy Concerns
As with any technological advancement, the deployment of emotion recognition systems raises significant ethical concerns, particularly regarding privacy. The ability of AI to interpret emotions from facial expressions and body language means that individuals may be subjected to emotional surveillance without their consent. This can lead to a variety of ethical dilemmas, including unwanted profiling or discrimination based on emotion.
To address these concerns, it is essential for stakeholders, including developers, policymakers, and society at large, to engage in open discussions about ethical guidelines and privacy regulations. Establishing robust frameworks will help safeguard individuals’ rights while enabling the responsible use of emotion recognition technologies.
Accuracy and Reliability
Another critical aspect is the accuracy and reliability of emotion recognition systems. While advancements in AI have led to impressive abilities in emotion detection, there is still a risk of misclassification. Misinterpretation of emotions can have severe ramifications, particularly in high-stakes situations like healthcare or law enforcement.
To ensure that emotion recognition systems are effective, ongoing research and refinement of algorithms are necessary. Furthermore, incorporating diverse datasets during the training phase is vital in reducing bias and increasing overall accuracy.
Conclusion
The science behind emotion recognition in AI-generated imagery is a rapidly developing field that combines the depths of computer science, psychology, and ethics. As AI continues to evolve, its ability to recognize and interpret human emotions presents opportunities and challenges that merit thoughtful exploration.
By understanding facial expressions, body language, and employing multimodal techniques, AI systems can achieve remarkable feats in interpreting human emotions. The potential applications are vast, from advancing healthcare and enhancing marketing strategies to cultivating effective educational environments.
However, the road ahead is not without challenges. Ethical considerations around privacy and accuracy must be prioritized to ensure that these technologies benefit society without infringing on individual rights. The continued collaboration between technologists, psychologists, and ethicists is essential for fostering a future where emotion recognition technology serves humanity positively and responsibly.
As we stand on the cusp of increasingly sophisticated AI advancements, it’s crucial to navigate this territory with open communication and responsible practices, paving the way for a more emotionally intelligent and connected world.
If you want to read more articles similar to The Science Behind Emotion Recognition in AI-Generated Imagery, you can visit the Image Generation category.
You Must Read