
Navigating the Technical Challenges of Face Recognition in AI

Introduction
In the ever-evolving landscape of artificial intelligence (AI), one of the standout technologies that has captured both public interest and controversy is face recognition. This innovative application has found its way into various domains, ranging from security and surveillance to personalized marketing and user authentication. While the utility of face recognition is clear, it also brings forth a myriad of technical challenges that developers and researchers must navigate carefully to harness its full potential successfully.
This article aims to delve deep into the complexities surrounding face recognition technology, examining the myriad of technical challenges it faces today. From issues related to data privacy and bias in algorithms to the intricacies of achieving high accuracy and real-time processing, we will explore these challenges in detail, discussing their implications and potential solutions.
Understanding Face Recognition Technology
Face recognition technology has its roots in early computer vision studies and has evolved rapidly over the past few decades. At its core, face recognition works by identifying or verifying a person’s identity using their facial features. The process generally involves three main steps: face detection, where the system identifies faces in images; feature extraction, where specific characteristics of the detected face are analyzed; and matching, where the system compares the extracted features with those in a database.
The advances in deep learning and convolutional neural networks (CNNs) have significantly improved the performance of face recognition systems. By leveraging large datasets and powerful computing resources, these models can now achieve remarkable accuracy levels in identifying individuals. However, the technology is not without its flaws. Understanding the inherent challenges is crucial for anyone working in this fascinating field.
Adapting Face Recognition Systems for Diverse Environments and LightingTechnical Challenges of Face Detection
Face detection serves as the foundation for face recognition systems, but it is fraught with challenges. One primary challenge is variability in lighting conditions. Faces can look significantly different under varying light conditions—shadows, glare, or low-light situations can significantly affect detection accuracy. This variability necessitates robust algorithms capable of normalizing variations to ensure reliable detection in real-world scenarios.
Another challenge is pose variation. Faces can appear significantly different based on the angle from which they are viewed. For instance, a person could be facing left, right, or even turned at an angle—and these poses can confuse face detection algorithms trained primarily on frontal images. Addressing this requires extensive training on diverse datasets, including various poses, to enable systems to detect faces effectively from different angles.
Additionally, an essential aspect of face detection challenges includes occlusion caused by accessories like glasses, hats, or masks. Each of these obstructions can obscure critical facial features, leading to inaccurate detection or failure to recognize a face entirely. Algorithms must be designed with invariance to these occlusions to ensure reliable performance across varied scenarios.
Feature Extraction Accuracy
Once faces are detected, the next major challenge involves feature extraction. This is a critical step, as the algorithms must identify key attributes of the face that are unique and reliable for identification or verification purposes. A significant difficulty here lies in the algorithm's ability to generalize across a wide range of facial expressions. Different expressions can lead to alterations in the structure and proportions of facial features, which can confuse the recognition process.
A Beginner's Guide to Developing Face Recognition ApplicationsMoreover, the performance of feature extraction algorithms is heavily influenced by facial variability inherent in the population. Factors such as age, ethnicity, and gender can lead to unique characteristics in facial features that may not be adequately represented in the training dataset. This lack of diverse training data can result in biased algorithms that perform significantly better for certain demographic groups compared to others.
Furthermore, achieving high accuracy in feature extraction involves managing trade-offs between speed and precision. In many applications, especially in security and surveillance, systems must operate in real time. Striking the right balance between processing speed and accuracy becomes crucial, as delays can lead to missed identifications or heightened security risks.
Data Privacy and Ethical Considerations
As face recognition technology continues to proliferate, so do concerns regarding data privacy and ethical implications. Given that face recognition involves the collection and analysis of biometric data, there is a growing necessity for stringent regulations and policies to govern its use. The ethical dimensions of using this technology for surveillance and identification raise fundamental questions about personal privacy and consent.
One pressing concern is the potential for misuse of face recognition technology by state actors or private organizations. For instance, the deployment of facial recognition systems in public spaces can lead to unwarranted surveillance, infringing on individual rights. This scenario has prompted calls for transparent policies that ensure that individuals are informed about the use of such technologies and provide explicit consent for their data to be used.
Additionally, many jurisdictions are still grappling with the legal frameworks that govern biometric data. The lack of clear regulations can lead to erratic practices and inconsistencies in how face recognition is applied in various contexts. As developers and organizations continue to implement these systems, there is an urgent need to establish comprehensive legal guidelines that encompass data protection and the ethical use of AI technologies.
Addressing Algorithmic Bias
Another significant concern linked to face recognition technology is algorithmic bias. Studies have shown that many face recognition systems exhibit lower accuracy rates for individuals with darker skin tones, women, and other minority groups. This issue raises serious concerns about fairness and equity, suggesting that the technology must be calibrated to avoid exacerbating societal inequalities.
The roots of algorithmic bias often lie in the training datasets used to develop AI models. If these datasets lack diversity and representation, the resulting algorithms will inevitably reflect those deficiencies, leading to significant disparities in performance among different demographic groups. Tackling these issues requires concerted efforts to curate more inclusive datasets and employ techniques such as adversarial training, which seeks to enhance model robustness against biased interpretations.
Moreover, developers must implement ongoing evaluations and audits of their algorithms to identify and mitigate biases continually. By establishing standardized assessment protocols and benchmarks, the industry can promote fairness and accountability in face recognition technologies.
Conclusion
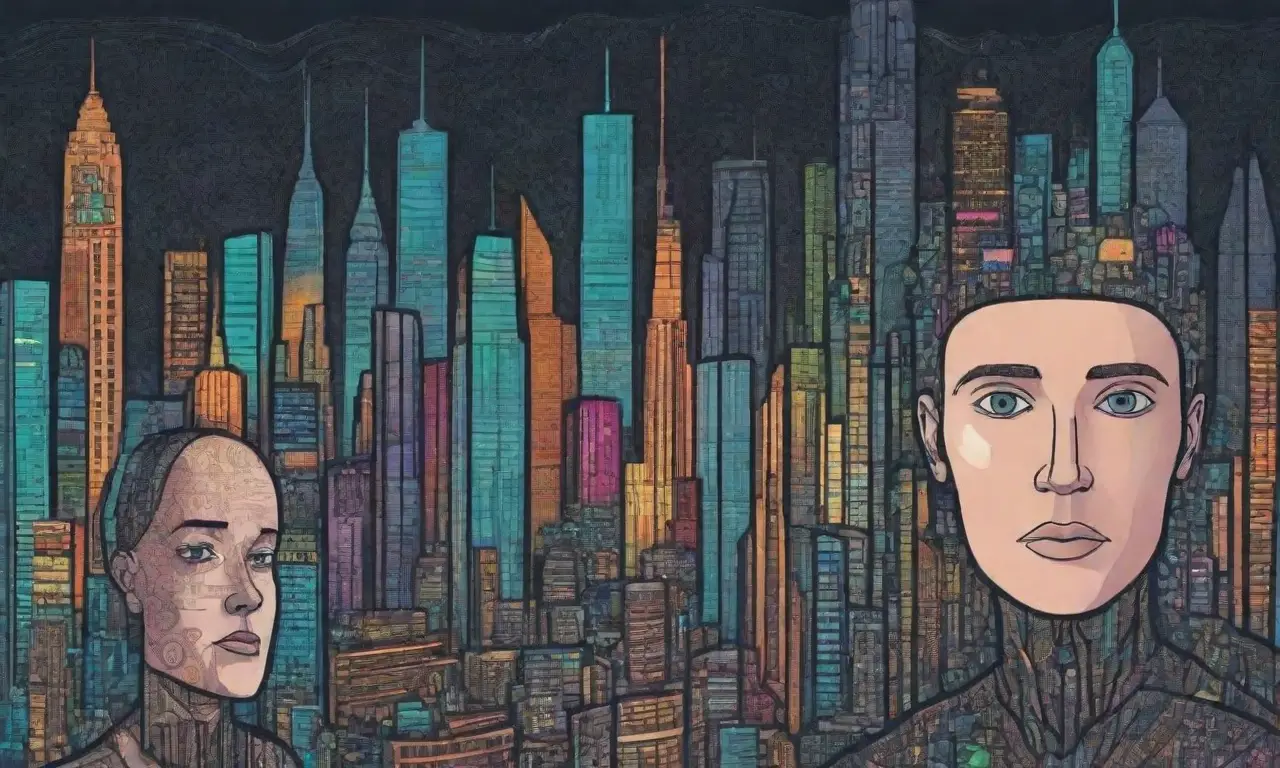
Navigating the technical challenges of face recognition in AI is a multi-faceted endeavor requiring substantial collaboration among computer scientists, ethicists, policymakers, and stakeholders from diverse backgrounds. As the technology matures, addressing issues related to face detection, feature extraction, data privacy, and algorithmic bias will be paramount.
Organizations must prioritize ethical implications and actively seek solutions to enhance fairness in face recognition systems. By adopting rigorous standards for data collection, ensuring diverse representation in training datasets, and fostering transparency throughout the development process, we can cultivate a more responsible approach to face recognition technology.
Looking forward, ongoing research, technological innovations, and comprehensive regulatory frameworks will be key to ensuring that face recognition technology serves humanity positively and equitably. Building a foundation of trust will not only benefit technological advancement but also society as a whole, balancing the promise of AI with profound respect for individual rights and personal privacy.
If you want to read more articles similar to Navigating the Technical Challenges of Face Recognition in AI, you can visit the Face Recognition category.
You Must Read