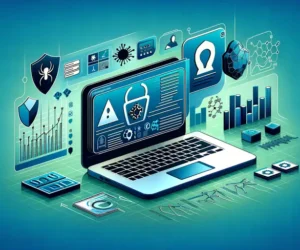
Adapting Face Recognition Systems for Diverse Environments and Lighting

Introduction
Face recognition technology has made remarkable advancements over the past few decades, revolutionizing the way we identify individuals in various contexts. From enhancing security measures to enabling personalized user experiences, the applications of face recognition systems have become increasingly prevalent across multiple domains. However, despite these technological strides, one of the significant challenges that persist is the system’s performance in diverse environments and varying lighting conditions. Variations in lighting, background clutter, and the emotional state of individuals can significantly affect the accuracy of these systems.
This article aims to explore the complexities of adapting face recognition systems to function effectively across diverse environments and lighting conditions. It will delve into the core challenges faced, the techniques employed in overcoming these challenges, and the ongoing research that strives to enhance system robustness. By the end of this article, readers will have a comprehensive understanding of the hurdles posed by environmental and lighting variations and the innovative solutions being implemented.
Challenges in Face Recognition Systems
Face recognition systems primarily rely on the unique features of a person's face to identify them accurately. However, several challenges arise when these systems encounter diverse environments. One of the most significant challenges is variations in lighting. Different lighting conditions can lead to dramatic changes in how facial features are perceived. For instance, harsh overhead lighting can cast strong shadows on the face, obscuring crucial features such as the eyes and nose, while extreme backlighting may wash out the facial details entirely. These fluctuations can introduce substantial discrepancies between the captured images and the reference images stored within the system, ultimately hindering accurate identification.
In addition to lighting, background clutter poses another challenge. Often, face recognition systems operate in environments with various distractions such as moving objects, busy backgrounds, and changing scenes. These distractions increase the likelihood of false positives or negatives, as the system may mistakenly interpret non-facial elements as key facial features or fail to identify the main subject amidst the chaos. Moreover, environmental conditions such as weather—be it fog, rain, or snow—can significantly obscure facial features, making it even more difficult for face recognition systems to function correctly.
A Beginner's Guide to Developing Face Recognition ApplicationsAnother core aspect to consider is the diversity of facial attributes. Individuals come from different ethnic backgrounds, age groups, and genders, each possessing unique facial characteristics. Traditional face recognition algorithms may struggle to provide accurate identification when individuals have attributes that deviate from the model they were trained on. This issue highlights the importance of ensuring that face recognition systems are trained using a robust, diverse dataset that encompasses a wide range of faces. Failure to consider diversity can lead to biased outcomes, exacerbating existing societal inequalities in technological deployment.
Techniques for Enhancing Face Recognition Systems
To tackle the challenges faced by face recognition systems in diverse environments and lighting conditions, researchers and practitioners have developed various techniques that aim to enhance the robustness of these systems. One prominent approach is the use of image preprocessing techniques. These methods focus on normalizing the input images to reduce the negative impact of adverse lighting conditions. Common preprocessing techniques include contrast enhancement, gray-scale conversion, and histogram equalization. Such processes help to enhance the contrast and visibility of facial features, allowing recognition algorithms to perform more effectively even in challenging lighting.
In recent years, the field has seen the emergence of deep learning techniques as a transformative solution for face recognition. Models based on Convolutional Neural Networks (CNNs) have gained popularity due to their capacity to learn complex hierarchical feature representations from large datasets. Such networks can be trained to recognize faces under various lighting conditions by incorporating a diverse array of training images. By exposing the system to multiple environments and lighting scenarios during training, it becomes more adept at distinguishing facial features even when conditions are less than ideal.
Moreover, the application of data augmentation has proven beneficial in enhancing the performance of face recognition systems. Techniques such as rotation, scaling, and color modification can artificially expand the training dataset, enabling the algorithms to learn specific variations that they might face in real-world applications. This not only improves the recognition accuracy but also reduces the risk of overfitting, which occurs when models become too specialized to the training data and fail to generalize to new situations.
Navigating the Technical Challenges of Face Recognition in AIMultiview Face Recognition
An additional technique that has gained traction in addressing face recognition challenges is multiview face recognition. This approach involves capturing facial images from various angles and orientations rather than solely frontal views. By using this technique, systems are better equipped to identify faces that may not be presented directly to the camera, which is particularly relevant in crowded or dynamic environments. Utilizing multiple views allows for a more comprehensive understanding of an individual’s facial features, thereby enhancing the system's ability to perform accurate recognition even when the person’s face is partially obscured or illuminated inconsistently.
Utilizing Robust Algorithms
Another promising development focuses on the use of robust algorithms that are designed to handle variances in lighting and other environmental factors. Researchers have been working on advanced algorithms, such as light-invariant face recognition, which enables systems to disregard the influence of lighting variations effectively. These algorithms are tailored to compute a robust representation of facial features that remain stable regardless of the illumination conditions. They leverage mathematical models to mimic how humans perceive faces, thus significantly improving the recognition rates across diverse environments.
Additionally, researchers are continually exploring fusion techniques, which involve combining data from multiple sources or modalities (such as thermal imaging alongside conventional visual data) to enhance recognition performance. This multidimensional approach helps in providing a more holistic understanding of the individual’s features, thereby allowing for accurate identification even in less-than-ideal conditions. For example, using thermal images can significantly improve recognition capabilities in low-light scenarios where traditional optical methods would struggle.
Future Directions in Face Recognition Research

As technology continues to evolve, the future of face recognition systems lies in the synergy between improved algorithms and hardware enhancements. Developing more sophisticated sampling techniques that can dynamically adapt to environmental variations will be crucial. This adaptability involves hardware that is capable of understanding context, allowing it to calibrate the image capturing process according to real-time environmental data such as lighting and obstacle detection.
Furthermore, employing ethics-aware approaches will be essential for the continued advancement of face recognition systems. With growing concerns about privacy and surveillance, it is crucial that future research pays close attention to bias mitigation and transparency in algorithm design. Ensuring that datasets used for training are diverse and representative of different demographics will help alleviate the ethical implications behind the technology's deployment.
Lastly, social acceptance will be a pivotal factor. As face recognition systems become more prevalent, understanding historical context and current societal trends will inform how these systems are designed to respect user privacy. Ongoing discussions surrounding regulations and policies will significantly shape the landscape of face recognition technology, guiding its ethical application in various sectors including law enforcement, education, and even personal devices.
Conclusion
In conclusion, adapting face recognition systems to function effectively in diverse environments and lighting conditions remains a complex yet critical challenge. The interplay between lighting variations, background clutter, and the diverse attributes of individuals introduces unique obstacles that require multifaceted solutions. However, technological advancements, including deep learning, data augmentation, and innovative algorithm design, have significantly enhanced the robustness and accuracy of these systems.
As research continues to evolve, it is imperative that the future innovations in face recognition prioritize ethical considerations, transparency, and social acceptance. Ensuring that these systems operate fairly, effectively, and respect user privacy will be crucial as they become increasingly integrated into our daily lives. Overall, the path forward is promising, with exciting advancements expected to further solidify the role of face recognition systems as reliable tools capable of navigating the complexities of varying environments and diverse lighting conditions.
If you want to read more articles similar to Adapting Face Recognition Systems for Diverse Environments and Lighting, you can visit the Face Recognition category.
You Must Read