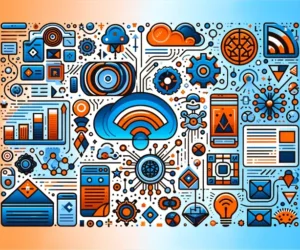
Building and Refining Data Sets for Music Generation Projects

Introduction
In the realm of artificial intelligence and machine learning, the concept of music generation has increasingly sparked interest among researchers, musicians, and tech enthusiasts alike. With the ability to create new and original compositions, machine-generated music offers a potent blend of creativity and technology, opening avenues for artistic expression that were previously unimaginable. However, the cornerstone of any successful music generation project lies in the quality of the data sets used to train these machine learning models.
This article aims to delve deeply into the complex and multifaceted process of building and refining data sets for music generation projects. We will discuss the importance of data collections, methodologies for acquiring quality data, techniques for effective data annotation, and intricate aspects of refining data sets to enhance model performance. By the end of the article, you will be equipped with a comprehensive understanding of how to create robust data sets that facilitate the development of advanced music generation systems.
Understanding Data Sets in Music Generation
The significance of data sets in music generation cannot be overstated; they serve as the bedrock upon which machine learning algorithms are built. Essentially, a data set consists of a collection of musical pieces used to train models, enabling them to learn patterns, styles, and structures inherent in music. Various types of data sets can be harnessed for different music generation goals, ranging from simple melodies to complex orchestral compositions.
One vital aspect of data sets is their diversity. To create a well-rounded model, it's essential to include a variety of genres, instruments, and cultural influences. By doing this, the generated music can resonate with a broader audience, appealing to listeners across different backgrounds and musical tastes. The richness in diversity allows the model to learn the nuances of different musical styles, blending characteristics effectively to generate unique compositions. Moreover, a diverse data set helps the model to avoid overfitting to a single genre, encouraging creativity in the generated outputs.
The Evolution of Algorithmic Music Generation Over the Last DecadeCollecting the right kind of data set is often a meticulous process. Various sources can be utilized, such as music databases, public domain resources, and even user-generated content platforms. It is crucial to ensure that the data collected is of high quality and relevant to the intended music generation objectives. For example, if you aim to create a model that generates classical music, gathering data exclusively based on pop music would be counterproductive. As such, identifying and aligning the data to your project goals is an instrumental step in this journey.
Data Collection Methods
The methods for collecting data sets in music generation projects are varied and can significantly impact the outcomes of the model. One of the widely recognized sources of music is music composition databases, such as IMSLP or Musopen, which provide a wealth of public domain compositions. These sources can yield a treasure trove of material for classical compositions that can be digitized for analysis. When collecting data from these sources, it is essential to ensure proper formatting and adherence to copyright guidelines, especially if you intend to share your work publicly.
Another prevalent method involves using data scraping techniques to gather music data from online platforms, streaming services, or digital archives. This process may involve extracting MIDI files, sheet music, or audio files from various sources. However, data scraping comes with ethical considerations; it requires adherence to the respective platforms' terms of service. This method, though efficient, necessitates careful consideration of the legality and ethics involved in using such scraped data.
Additionally, crowdsourcing is an emerging method that has proven useful in building data sets. Platforms like SoundCloud allow users to upload their work, making it available for others to utilize. By reaching out to musicians or online communities, you can request submissions under certain terms, making the data collection process collaborative. This not only enriches the diversity of the data set but also fosters a sense of community around the project, where artists contribute to the evolution of music generation technology.
Analyzing the Quality of AI-Generated Music: Research InsightsData Annotation Techniques
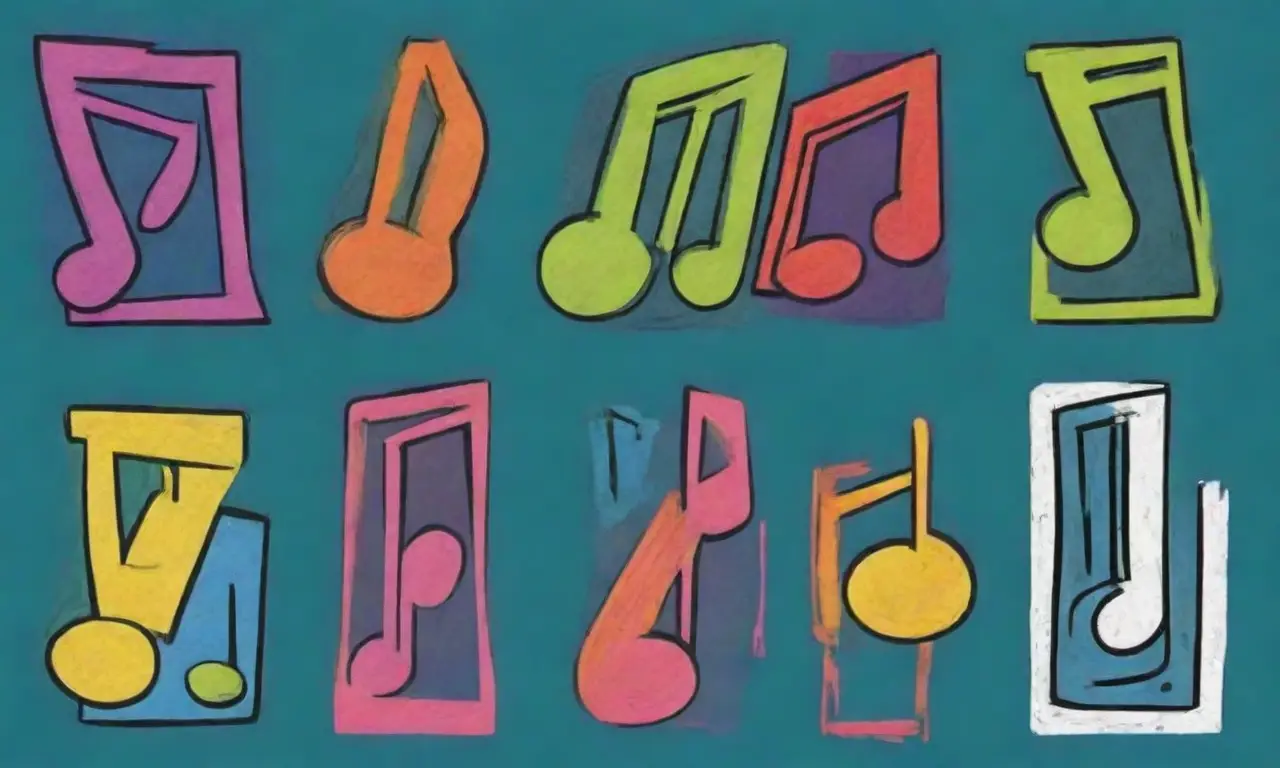
Once you have amassed your data set, the next crucial step is data annotation. This involves labeling the music files to provide context, structure, and additional information that facilitates machine learning. Data annotation is a pivotal aspect that can significantly enhance the model's training and output. There are several techniques to undertake effective data annotation in music generation projects.
One of the fundamental approaches involves creating metadata for each piece of music in the data set. Metadata can include various attributes such as the genre, speed (BPM), key signature, instrumental arrangement, and even emotional context (e.g., happy, sad). This detailed information allows machine learning algorithms to better understand the music styles and replicate them effectively in generated compositions. Without adequate metadata, the model may struggle to learn specific characteristics that define different musical pieces.
Another powerful technique is to annotate the data with musical features. These features can be extracted using specific algorithms and tools to represent aspects like melody, harmony, rhythm, and dynamics. Tools such as Melody Extraction or Tempo Analysis can help in analyzing audio files and converting them into structured data. By incorporating such features, models can learn complex musical structures more intuitively, resulting in higher-quality compositions that reflect realistic music elements.
Exploring Neural Networks for Autonomous Music Composition TechniquesMoreover, manual annotation by music experts can add a layer of quality to the data set. Trained musicians can evaluate the dataset, assessing the quality of melodies, harmonies, and arrangements. They may provide insights that offer an additional layer of quality assurance often not captured by automated tools. This human-in-the-loop approach can be particularly useful for refining the dataset in preparation for machine learning, ensuring that it meets the desired quality standards.
Refining Your Data Set
As your data set takes shape, it is essential to engage in refinement to enhance its quality and relevance continuously. The refinement process involves cleaning the data, removing duplicates, and smoothing out inconsistencies. The goal is to arrive at a data set that is not just comprehensive but also streamlined for better learning.
One critical component of data refinement is data cleaning, which involves identifying and rectifying errors in the data set. This can involve removing corrupted files, resolving inconsistent labeling, or correcting attribute errors. For example, if one part of the dataset labels a composition as "jazz" while another section marks it as "blues," such discrepancies need to be resolved to maintain clarity. Effective data cleaning ensures that the machine learning model learns from accurate and consistent information.
Additionally, there's a need for continuous evaluation and testing of the dataset. Periodically testing the model against the data can reveal unanticipated biases or shortcomings in the data set. This can lead to adjustments to the selection or weighting of parts of the dataset that are not performing as expected. Moreover, gathering feedback on music output from potential users can offer further insights into the model's effectiveness, laying the groundwork for further refinement.
Building Your First AI Music Generator with Open Source LibrariesFinally, employing techniques like data augmentation can breathe new life into your data set, especially if it is limited in size. By manipulating original music files—through methods such as tempo alterations, pitch changes, or adding reverb effects—you can create variations of the data, thereby expanding the volume of training examples available to the model. This strategy enables the system to generalize better while learning from a diversified pool of examples.
Conclusion
Building and refining data sets for music generation projects is a multifaceted endeavor that requires careful planning, execution, and continuous refinement. As we explored, the process begins with understanding the significance of data and collecting diverse and relevant data sources. The importance of data annotation, specifically metadata and musical features, provides essential context for machine learning algorithms to learn effectively. Refinement through data cleaning, evaluation, and augmentation significantly enhances the quality of the data set, resulting in improved model outcomes.
By recognizing the intricacies involved in building and refining music data sets, aspiring music technologists and researchers can create robust models that generate high-quality musical content. As technology evolves, and music generation becomes increasingly integrated with AI, the significance of well-structured data sets will continue to rise, underpinning the successful marriage of creativity and technology. Ultimately, as you embark on your own music generation project, remember that the quality of your data set is paramount in unveiling the full potential of machine-generated music, paving the way for new musical innovations in the years to come.
Using Constraint Satisfaction in Algorithms for Music GenerationIf you want to read more articles similar to Building and Refining Data Sets for Music Generation Projects, you can visit the Music Generation category.
You Must Read