
Building Better Customer Relationships Through ML Insights
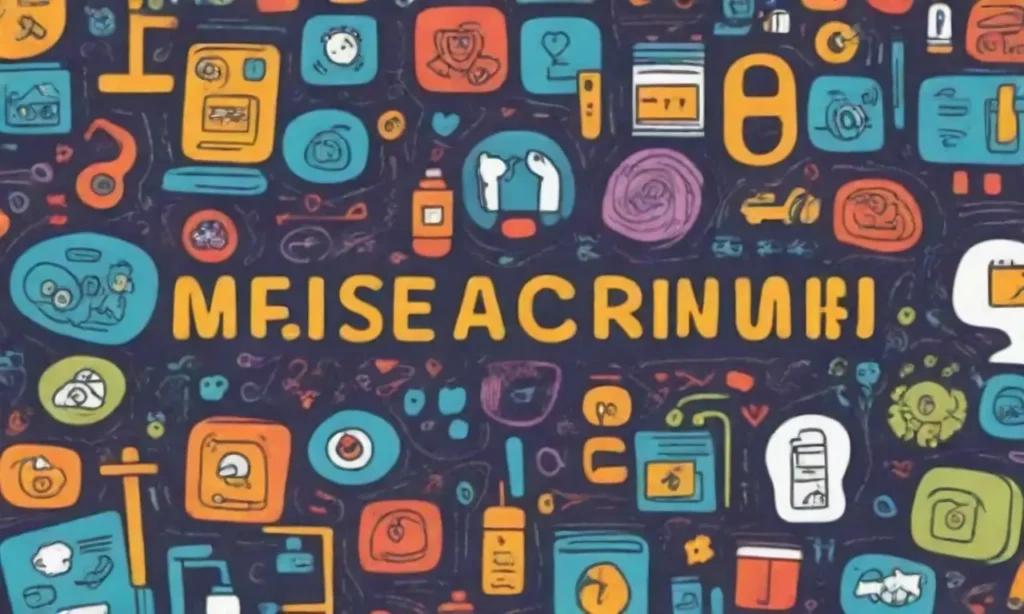
Introduction
In the contemporary business landscape, customer relationships have transitioned from traditional interactions to a more sophisticated engagement driven by technology. The advent of Machine Learning (ML) has brought about a paradigm shift in how businesses analyze customer behavior, preferences, and feedback. By leveraging these insights, companies can tailor their strategies to foster deeper connections with clients, increase loyalty, and ultimately drive profitability.
This article delves into the integration of Machine Learning in understanding and enhancing customer relationships. We will explore how businesses can utilize ML technologies to gather and interpret data, personalize engagements, optimize customer service, and predict future trends, thereby enriching the overall customer experience.
The Role of Machine Learning in Customer Insights
Machine Learning stands as a crucial technology in enabling businesses to sift through massive amounts of data, revealing customer insights that were previously inaccessible. By employing algorithms that learn from patterns in data, organizations can gain a comprehensive understanding of their customer base. This knowledge extends beyond mere demographics; it encompasses purchasing behaviors, feedback patterns, and preferences.
One of the primary functions of ML in analyzing customer behavior is through predictive analytics. By processing historical data, ML models can identify trends that indicate how a customer is likely to act in the future. For instance, businesses can determine which products a customer is likely to purchase based on their past buying habits, enabling companies to tailor marketing strategies to trigger those purchasing decisions. This proactive approach to customer engagement not only enhances the chances of conversion but also establishes a personalized relationship with the client.
Exploring the Intersection of ML and Human Touch in SupportFurther, ML can be instrumental in segmenting customers into distinct categories based on behavioral insights. By analyzing demographics, purchasing patterns, and feedback, businesses can classify customers into various segments, such as "loyal customers," "new customers," or "at-risk customers." Such segmentation allows for targeted marketing efforts that resonate with the specific needs and preferences of each group, fostering a more tailored customer experience and facilitating stronger relationships.
Enhancing Customer Engagement with Personalization
Personalization lies at the heart of building robust customer relationships, and Machine Learning plays a pivotal role in creating tailored experiences that resonate with individuals. With ML algorithms analyzing customer data, businesses can develop personalized marketing communications, product recommendations, and overall customer interactions. Personalized approaches instill a sense of value and recognition in customers, fostering loyalty and increasing satisfaction.
For instance, consider e-commerce platforms that utilize ML for product recommendations. These platforms analyze a customer's browsing history and previous purchases to suggest items that align with their interests. This technology not only increases the likelihood of additional sales but also enriches the shopping experience, showcasing the brand's understanding of the customer's preferences. When customers feel acknowledged and catered for, it paves the way for lasting relationships with the brand.
Moreover, businesses can leverage ML to deliver personalized content via email campaigns or digital platforms. By understanding customer behavior—what content they engage with, when they engage, and how they interact—companies can fine-tune their messaging to suit individual tastes. For instance, an email promoting a new product line can be personalized based on a customer's previous interactions, enhancing engagement rates and making customers feel valued. When customers feel like the offerings are a response to their unique preferences rather than generic mass marketing, it strengthens their connection with the brand.
Creating Effective ML Workflows for Customer Support AutomationOptimizing Customer Service with Machine Learning

In the realm of customer service, Machine Learning is a game-changer. By integrating ML into service operations, businesses can enhance their efficiency, responsiveness, and the quality of customer interactions. One of the most notable applications is the use of chatbots powered by ML algorithms. These chatbots can handle customer queries in real-time, providing instant responses and support round the clock.
The efficiency of ML-powered chatbots comes from their learning capabilities. They can analyze past interactions to improve responses continually, understanding common issues and typical customer queries. This ability not only frees up human customer service representatives to tackle more complex issues but also ensures that customers receive immediate support. In scenarios where speed is crucial, such as addressing complaints or urgent inquiries, ML chatbots play an invaluable role in maintaining customer satisfaction.
In addition to standard queries, Machine Learning can assist in sentiment analysis, which further enhances customer service quality. By analyzing customer feedback, reviews, and social media interactions, businesses can gauge the emotional tone behind customer communications. Sentiment analysis allows businesses to identify potential issues before they escalate and address them proactively. For example, if a significant number of customers express dissatisfaction with a particular product, ML can alert the company, allowing them to take corrective measures swiftly. Such responsiveness not only improves customer satisfaction but also builds trust in the brand.
Ethical Considerations in Customer Support Automation with AIPredicting Customer Behavior and Trends
Understanding customer behavior is instrumental in driving successful business outcomes, and Machine Learning provides powerful tools to achieve this. By harnessing historical and real-time data, businesses can anticipate changes in customer preferences and market trends, ensuring they stay ahead of the competition.
One significant application of ML is in forecasting customer demand. Using time-series analysis and regression models, businesses can predict when customers are likely to make purchases, what products they will prefer, and how external factors, such as seasonal trends or economic changes, will influence their behavior. This forecasting capability allows companies to optimize inventory levels, adjust marketing strategies, and refine overall business operations to align with anticipated customer needs.
Furthermore, Machine Learning can enhance customer retention strategies by identifying customers who are at risk of leaving. By analyzing usage patterns, purchase frequencies, and engagement levels, businesses can spot warning signs that indicate a dip in customer loyalty. Once identified, targeted retention strategies can be deployed—such as personalized offers or loyalty programs—to re-engage those customers. Preventing churn not only retains revenue but also strengthens the brand's relationship with existing customers.
Conclusion
In an era where customer expectations are continually evolving, building better customer relationships is paramount for businesses aiming to thrive. Machine Learning serves as a catalyst in this endeavor, enabling organizations to harness vast amounts of data, derive actionable insights, and implement personalized engagement strategies. From enhancing customer engagement through personalized recommendations to optimizing customer service with efficient chatbots and predictive analytics, ML empowers businesses to create meaningful connections with their clients.
Real-time Customer Support: ML Models that Respond InstantlyAs organizations continue to embrace the potential of Machine Learning, it becomes essential to foster a culture that values data-driven decision-making. Investing in the right technologies, training employees, and continuously monitoring customer sentiments will position businesses to not just meet but exceed customer expectations.
In conclusion, the journey of building better customer relationships through ML insights is ongoing. Businesses that prioritize understanding their customers through data-driven approaches will be more equipped to adapt in a competitive market. Ultimately, cultivating strong customer relationships leads to enhanced loyalty, increased lifetime value, and sustainable success in the long run. As ML continues to evolve, companies must remain agile, embracing new technologies and methodologies to stay ahead in their customer relationship strategies.
If you want to read more articles similar to Building Better Customer Relationships Through ML Insights, you can visit the Customer Support Automation category.
You Must Read