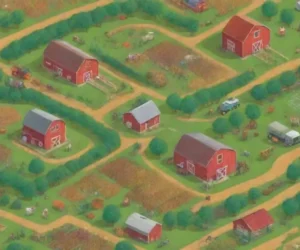
Clinical Applications of Machine Learning in Cardiovascular Processes

Introduction
The advent of machine learning (ML) has revolutionized various fields, and healthcare, particularly cardiology, is no exception. As professionals seek innovative solutions to improve patient outcomes, preventing cardiovascular diseases (CVDs) has emerged as a primary objective. Machine learning offers vast potential in analyzing complex datasets, identifying patterns, and making predictions that enhance the diagnosis, treatment, and management of cardiovascular diseases. This article delves into various clinical applications of machine learning in cardiovascular processes, illustrating its transformative effects on how we approach heart health.
As we navigate through this article, we will uncover how machine learning is applied across multiple facets of cardiovascular healthcare, from risk assessment and image processing to treatment recommendations and patient monitoring. By examining real-world applications, benefits, challenges, and future directions, we aim to provide an expansive overview of how machine learning is shaping the landscape of cardiovascular health.
Machine Learning in Risk Assessment
One of the most important applications of machine learning in the cardiovascular domain is in risk assessment. Traditionally, risk factors such as family history, high blood pressure, obesity, and lifestyle choices have been used to estimate an individual's risk for developing cardiovascular diseases. However, machine learning enhances this process through the analysis of vast amounts of patient data. Using algorithms, ML can predict which patients are at a higher risk of experiencing conditions like a heart attack or stroke.
Machine learning models can utilize structured data, such as demographic information, laboratory results, and medical history, along with unstructured data, including clinical notes and other forms of free text. For instance, algorithms such as Logistic Regression, Random Forest, and Support Vector Machines have demonstrated effectiveness in identifying high-risk patients by processing aggregated features from electronic health records (EHRs). These approaches not only improve prediction accuracy but can also uncover previously unknown risk factors that may contribute to cardiovascular diseases.
Moreover, machine learning can facilitate the calculation of risk scores tailored to the individual patient, enabling doctors to prioritize those in greatest need of intervention. For example, the Framingham Risk Score has been historically used for this assessment. By integrating machine learning, healthcare professionals can enhance the risk stratification process, ensuring that preventative measures can be implemented timely, thereby reducing the overall burden of cardiovascular diseases on healthcare systems.
Machine Learning in Cardiovascular Imaging
The application of machine learning in cardiovascular imaging marks another significant advancement in the field. Cardiologists widely use imaging modalities such as echocardiography, MRI, and CT scans to evaluate heart health. However, analyzing these images can be labor-intensive and requires considerable expertise. Machine learning algorithms, particularly deep learning, are now at the forefront of automating and enhancing imaging analysis.
Deep learning models such as convolutional neural networks (CNNs) can analyze images to detect anomalies, such as stenosis, cardiomyopathy, or atherosclerosis, with remarkable precision. These algorithms can be trained to differentiate between normal and abnormal structures in the heart using vast datasets of annotated images. As a result, these ML applications are not only improving diagnostic accuracy but also enabling faster processing times, which is crucial in acute settings where timely intervention can be lifesaving.
Additionally, the integration of machine learning in imaging has led to new possibilities, such as the prediction of patient outcomes based on imaging features. For example, certain algorithms can analyze the left ventricular ejection fraction from echocardiograms to gauge the prognosis of heart failure patients. The ability to automatically extract and interpret image-based biomarkers provides cardiologists with invaluable insights into individual patient conditions, allowing for a more personalized approach to treatment.
Treatment Recommendations and Decision Support
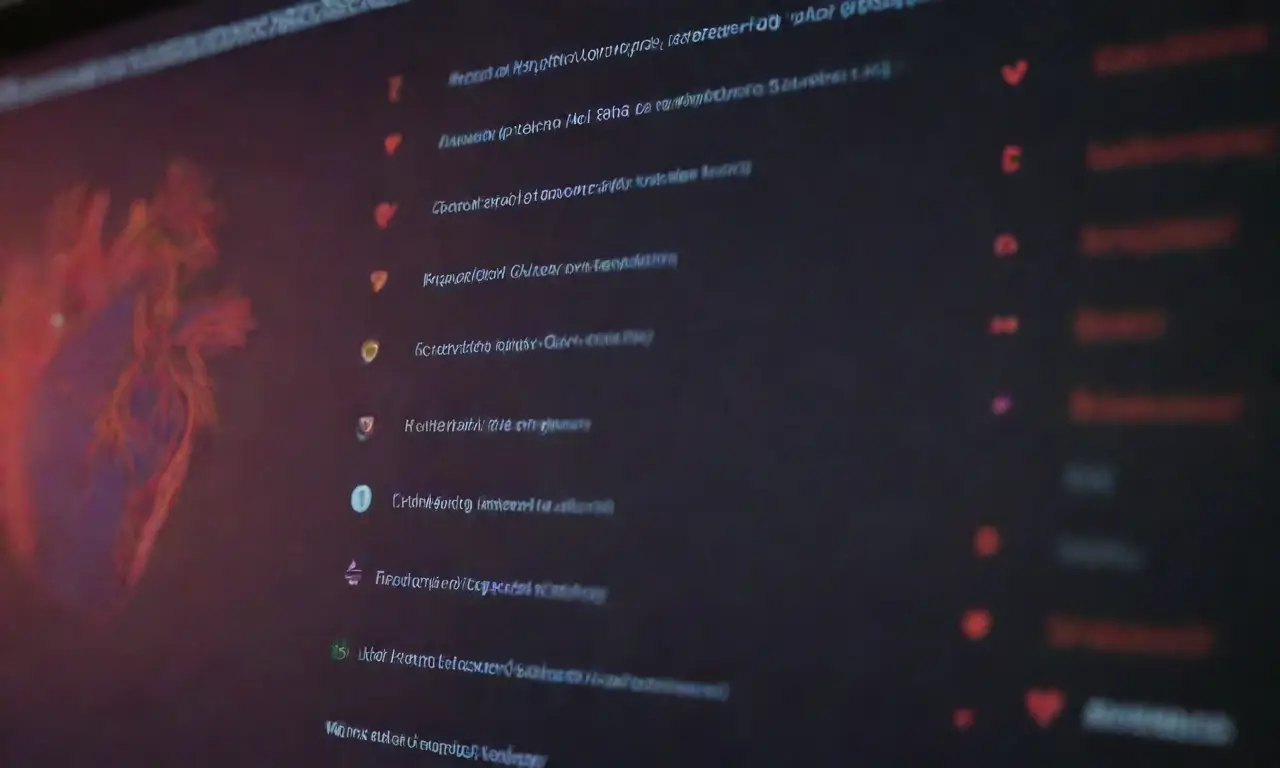
Machine learning extends beyond diagnosis to significantly impacting treatment recommendations and clinical decision-making. Decision support systems powered by machine learning can analyze a wealth of patient data to inform healthcare providers about the most effective treatment options tailored to specific patients. This involves assimilating data from clinical trials, real-world evidence, and treatment outcomes, facilitating a more comprehensive understanding of patient care strategies.
For instance, ML can be used to evaluate the effectiveness of various pharmacologic interventions based on a patient's unique profile. Algorithms can consider factors such as age, sex, genetic predispositions, and previous treatment responses to recommend personalized medication regimens. This level of precision medicine can optimize treatment efficacy while minimizing potential side effects, significantly enhancing patient satisfaction and adherence.
Furthermore, machine learning is also valuable in predictive analytics, which can forecast patient responses to certain treatments. For instance, researchers may apply reinforcement learning algorithms to derive optimal treatment paths based on patterns observed in treatment datasets, correlating specific interventions with positive patient outcomes. This adaptability means treatment protocols can evolve until they effectively address the needs of individual patients.
Remote Monitoring and Telehealth Applications
The rise of telehealth has gained tremendous momentum in recent years, especially following the global pandemic. Machine learning plays a crucial role in enhancing how healthcare systems can monitor patients remotely and optimize clinical workflows. Continuous remote monitoring of patients with cardiovascular diseases is now feasible through wearables and mobile health applications that utilize machine learning to analyze real-time data.
For example, wearable devices equipped with sensors can track vital signs, such as heart rate, blood pressure, and activity levels. Machine learning algorithms can process these data streams to detect irregularities, which may signal acute issues like arrhythmias or heart failure exacerbations. Such early warnings provide healthcare providers with real-time insights, allowing for timely interventions that can prevent hospitalization and other complications.
Moreover, machine learning enhances the efficiency of virtual consultations by providing healthcare providers with systematic insights drawn from patient history and real-time health data. This information can inform clinical discussions, making them increasingly relevant and personalized. Additionally, these applications can prioritize patients based on urgent health needs, ultimately ensuring that healthcare resources are allocated effectively.
Challenges and Limitations in Machine Learning in Cardiology
Despite its numerous advantages, the integration of machine learning into cardiovascular processes also faces several challenges and limitations. For instance, one of the primary obstacles is the quality of data. Machine learning models require substantial and high-quality datasets to perform effectively. However, the presence of missing values, inconsistent formatting, and unstandardized data across different healthcare systems can lead to biased or erroneous predictions.
Another significant challenge lies in the interpretability of machine learning models. While ML can provide accurate results, understanding how these models arrive at decisions remains difficult. This lack of transparency can be a barrier to widespread adoption, as healthcare professionals need to trust these systems in clinical settings. Ensuring that algorithms are explainable and present decision support in an intuitive manner is crucial for enhancing clinician acceptance.
Lastly, surgical concerns must be considered when implementing machine learning in clinical practice. Issues related to patient privacy, data security, and adherence to regulatory standards create a complex landscape. As data collection grows, ensuring that patient information remains confidential and secure must be prioritized, as concerns regarding data misuse can hinder progress in adopting ML technologies in healthcare.
Conclusion
The intersection of machine learning and cardiovascular healthcare presents immense opportunities for innovation, efficiency, and improved patient outcomes. From enhancing risk assessment and automating imaging analysis to optimizing treatment recommendations and facilitating remote monitoring, machine learning's influence is transforming traditional approaches to heart health. However, as we embrace these advanced methodologies, it is also essential to address the accompanying challenges, such as data quality, interpretability, and ethical considerations.
The future of machine learning in cardiovascular processes appears promising, with ongoing research and technological advancements expected to pave the way for broader applications and acceptance. As algorithms become increasingly sophisticated and integrated within clinical settings, there will undoubtedly be new opportunities to not only better predict and treat cardiovascular diseases but also to foster a more personalized approach to patient care.
Integrating machine learning in healthcare is ultimately about enhancing patient outcomes, reducing healthcare costs, and promoting a proactive approach to heart disease management. As stakeholders collaborate to refine data practices, enhance explainability, and strengthen regulatory frameworks, we can look forward to a healthier future where cardiovascular diseases are managed with unprecedented precision and effectiveness.
If you want to read more articles similar to Clinical Applications of Machine Learning in Cardiovascular Processes, you can visit the Applications in Healthcare category.
You Must Read