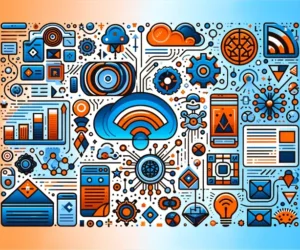
Conducting Sensitivity Analysis in Supply Chain with ML Techniques
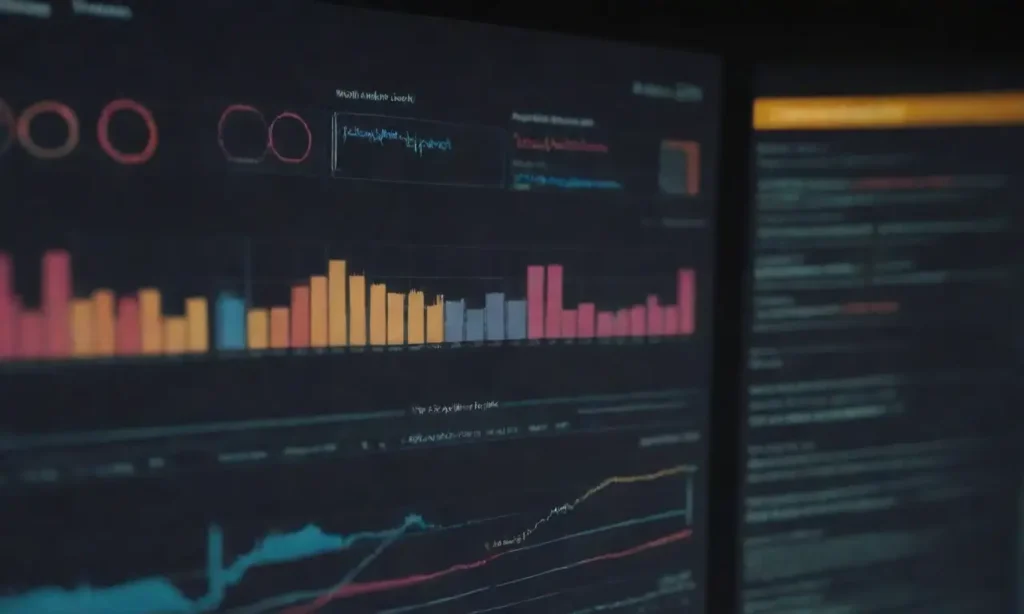
Introduction
In the dynamic landscape of modern supply chains, organizations are tasked with navigating uncertainties while optimizing performance. Sensitivity analysis, a crucial component of decision-making, aids supply chain professionals in understanding how changes in input variables can impact outcomes. With the advent of machine learning (ML) techniques, executing sensitivity analysis has become more sophisticated and insightful, enabling analysts to derive actionable insights from complex data sets. This article delves into the concepts of sensitivity analysis and machine learning, examining how these elements synergistically enhance supply chain management.
In the following sections, we will explore the core principles of sensitivity analysis, its significance in supply chains, and how machine learning techniques can revolutionize this process. We will discuss various ML models, the methodologies for implementing sensitivity analysis, and the benefits that arise from integrating these elements. By the end of this article, readers will have a comprehensive understanding of how to apply ML techniques to sensitivity analysis and the pivotal role it plays in optimizing supply chain strategies.
Understanding Sensitivity Analysis
Sensitivity analysis is a potent analytical tool used to evaluate how variations in input parameters affect a model's outcomes. In supply chain management, this means analyzing how changes in factors such as demand, supply lead times, and production costs influence critical performance indicators, such as profitability or service levels. This analysis helps leaders in making informed decisions about inventory management, supplier relationships, logistics, and overall operational efficiency.
The foundational principle of sensitivity analysis lies in understanding the relationships between different variables in a system. By systematically varying these inputs and observing the corresponding changes in outputs, analysts can identify which variables have the most significant impact on the model's results. This understanding drives both strategic and tactical decisions, facilitating effective responses to market changes or disruptions. For instance, during unforeseen events like natural disasters or political unrest, quick access to sensitivity analysis can help businesses pivot their strategies effectively to maintain supply chain continuity.
Skills and Knowledge Required for ML in Supply Chain OptimizationIn traditional sensitivity analysis, linear models and simple statistical techniques were often employed. However, these methods can be limited in their ability to capture complex non-linear relationships typical in supply chains. As a result, the integration of machine learning techniques has emerged as an essential enhancement, allowing organizations to delve deeper into data patterns and correlations that were previously difficult to discern.
The Role of Machine Learning in Supply Chain Sensitivity Analysis
The infusion of machine learning into sensitivity analysis provides robust tools for uncovering complex data relationships. ML algorithms can handle vast amounts of input data, identify patterns, and predict outcomes based on historical relationships, making it ideal for modeling supply chain dynamics. Through these insights, organizations can optimize various supply chain parameters and improve their responsiveness to changes.
One key aspect of implementing machine learning for sensitivity analysis is the selection of appropriate models. Several algorithms can be leveraged, including decision trees, support vector machines (SVM), and neural networks. For example, decision trees can simplify complex decision-making processes—allowing analysts to visualize how changes in specific variables affect overall supply chain performance. Additionally, neural networks can be particularly effective in identifying intricate non-linear relationships within large datasets, producing more accurate predictions compared to traditional statistical methods.
Moreover, machine learning techniques provide the advantage of automation and scalability. Once trained, these models can rapidly simulate multiple scenarios by altering input variables, enabling faster and more comprehensive sensitivity analysis. This speed is particularly beneficial during disruptions when timely decision-making is critical. For instance, a retail company could use machine learning models to simulate various promotional strategies by adjusting parameters like pricing, advertising spend, and inventory levels, identifying the optimal approach to maximize sales while minimizing costs.
How Collaborations Can Enhance ML Applications in Supply ChainsMethodologies for Conducting Sensitivity Analysis with ML
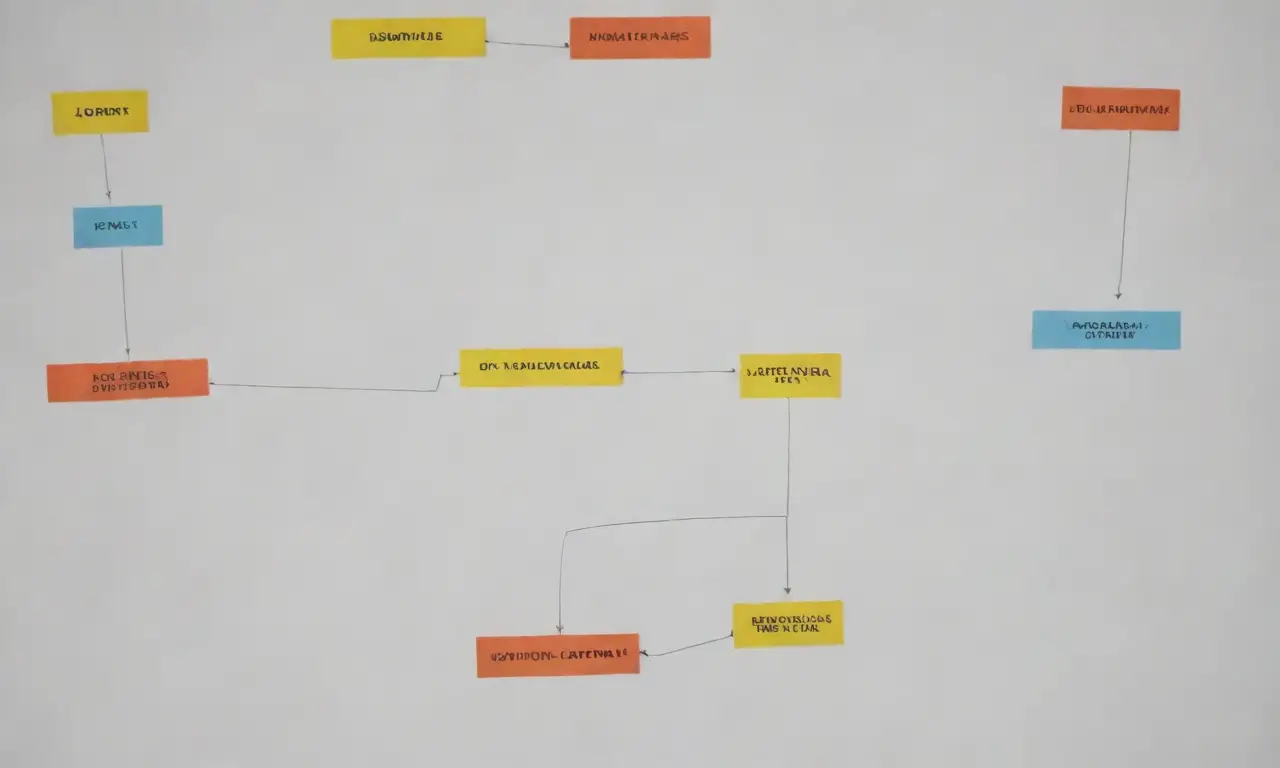
When integrating machine learning techniques into sensitivity analysis, a systematic approach is essential to maximize understanding and insights. This process typically consists of several key steps, starting with data preparation, followed by model selection, training, scenario testing, and finally, interpreting results.
Data Preparation
The first step in any sensitivity analysis is data collection and preparation, which is critical for ensuring the accuracy and reliability of the analysis. Collected data must come from various sources throughout the supply chain, including sales data, inventory levels, supplier lead times, and external factors such as market trends. To ensure the completeness and validity of the dataset, analysts may need to clean and preprocess the data, removing outliers and filling in missing values.
Next, it is essential to identify the input variables that will be varied during the analysis. These could include price changes, variations in demand forecasts, modifications in lead times from suppliers, or fluctuations in production costs. By carefully selecting which variables to analyze, the researchers can focus on the aspects of the supply chain that are most prone to risk or uncertainty, enabling targeted optimization efforts.
Utilizing Neural Networks for Enhanced Demand ForecastingModel Selection and Training
After preparing the data, analysts must choose the appropriate machine learning models for their specific context and goals. Different models can yield varying insights, and the choice often depends on the nature of the data and the complexity of relationships being analyzed. Once the models are selected, the next phase involves training them on historical data, which allows the models to learn patterns and correlations.
During the training phase, it is imperative to split the dataset into training and testing subsets to validate the performance of the models. By assessing the model's predictive accuracy against the testing data, analysts can refine their approaches, tune parameters, and ultimately select the best-performing model for use in sensitivity analysis.
Scenario Testing and Interpretation
With the models finalized, analysts can now conduct sensitivity analysis by simulating various scenarios. This might entail systematically changing input parameters in the model—such as increasing supplier lead times or decreasing product prices—and observing the corresponding changes in outcomes. Through this process, analysts can identify which variables most profoundly impact performance goals, allowing businesses to prioritize focus areas for improvement.
Once the simulations are completed, interpreting the results is crucial in deriving actionable insights. Analysts should visualize the outcomes through graphs or charts to elucidate trends and relationships clearly. Sensitivity graphs can show the sensitivity of outcomes concerning different input variables, helping stakeholders understand the potential ramifications of changes in the supply chain effectively.
Exploring the Ethical Implications of AI in Supply ChainsBenefits of Machine Learning-Driven Sensitivity Analysis
The advantages of implementing machine learning techniques in sensitivity analysis are numerous and can significantly improve supply chain performance. One of the foremost benefits is enhanced predictive accuracy. By utilizing advanced algorithms, organizations can uncover hidden patterns and relationships not easily identifiable through classical methods. This increased precision enhances strategic decision-making and prepares organizations for various scenarios.
Another critical advantage of ML-driven sensitivity analysis is the speed at which insights can be generated. Traditional analysis can be time-consuming, particularly when handling large datasets. In contrast, machine learning algorithms can process and analyze extensive data rapidly, enabling organizations to react swiftly to emerging opportunities or risks. This capability is especially vital in industries with high volatility, where timely decision-making can lead to competitive advantages.
Furthermore, using machine learning in sensitivity analysis allows for a greater degree of scalability. As data continues to grow in volume and complexity with technological advancements, traditional methods may struggle to keep pace. Machine learning techniques, however, can seamlessly scale to accommodate larger, more diverse datasets—making them indispensable in the evolving landscape of supply chain management.
Conclusion
In conclusion, sensitivity analysis paired with machine learning techniques represents a transformative approach for optimizing supply chain performance. The insights garnered from combining these methodologies empower organizations to understand critical variables and their impacts better, enabling them to adjust strategies swiftly in response to internal and external changes. By formalizing the analytical process through systematic methods—from data preparation to model selection and scenario testing—analysts can derive powerful insights that directly inform decision-making.
Automating Supply Chain Processes with Machine Learning TechnologiesAs supply chains face increasing complexities and uncertainties, the integration of machine learning into sensitivity analysis becomes a vital tool in a company's arsenal. Businesses that embrace this innovative blend will not only enhance their operational efficiency but also secure a competitive edge in an ever-evolving market. Moving forward, it will be essential for organizations to continually explore novel approaches and advancements in machine learning to further refine their sensitivity analysis processes and maximize their overall supply chain effectiveness.
If you want to read more articles similar to Conducting Sensitivity Analysis in Supply Chain with ML Techniques, you can visit the Supply Chain Optimization category.
You Must Read