
Creating Robust Models for In Silico Drug Discovery Validation
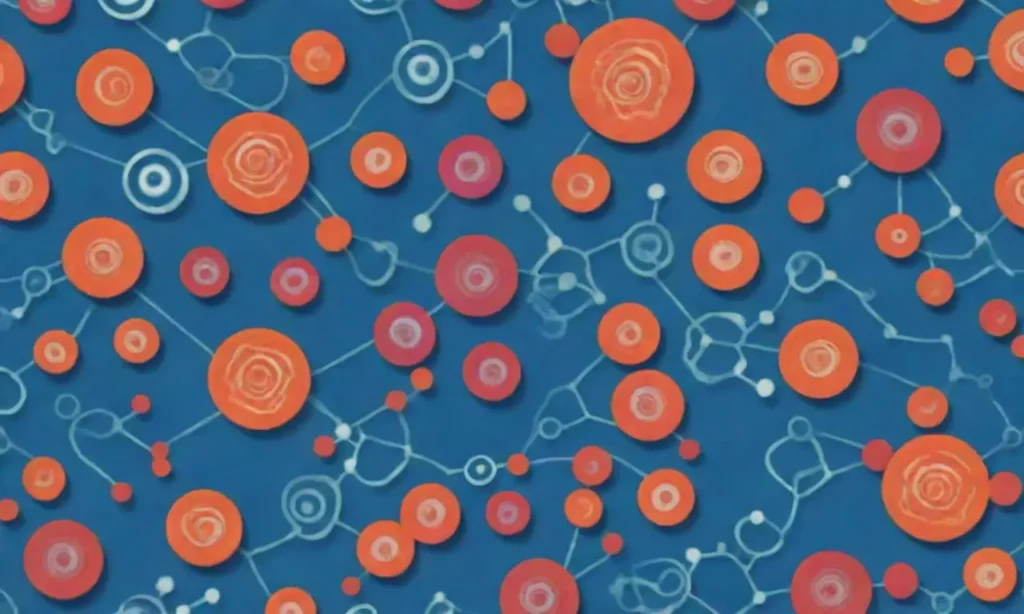
Introduction
In recent years, the pharmaceutical industry has been significantly transformed by the advancement of in silico drug discovery methods. These computational techniques allow researchers to virtually simulate the interactions between drugs and biological systems, effectively accelerating the drug development process. With the ability to predict the efficacy and safety of new compounds early in the research phase, in silico methods are becoming an essential component of the drug discovery pipeline.
This article delves into the intricacies of creating robust models for validating in silico drug discovery. We will explore the various methodologies, the importance of data quality, the types of models employed, and their practical applications in the pharmaceutical industry. By understanding how to create and validate these models, researchers can ensure greater accuracy and reliability in their predictions, ultimately leading to more effective drug candidates.
The Importance of Robust Models in Drug Discovery
The significance of robust models in drug discovery cannot be overstated. In silico methods serve as a bridge between theoretical research and practical application, offering several advantages that traditional laboratory experiments cannot provide. For instance, these models allow researchers to assess a vast number of potential drug compounds quickly and cost-effectively, facilitating the rapid identification of lead candidates.
Moreover, robust models are crucial for minimizing the risks associated with clinical trials. By incorporating predictive algorithms and machine learning approaches, researchers can better forecast how a drug will behave in living organisms based on its chemical properties and biological targets. This not only enhances the success rate of drug candidates but also reduces the time and financial resources expended on less promising compounds.
The Role of Deep Learning in Predicting Drug-Target InteractionsAdditionally, robust models help to standardize the drug discovery process. With the increasing complexity of biological data, inconsistencies can lead to conflicting results and hinder the overall progress of research. By establishing well-defined frameworks for model creation and validation, researchers can ensure that their findings are reproducible and credible. This is pivotal for gaining regulatory approval and fostering trust among stakeholders in the pharmaceutical industry.
Key Components of Building Robust In Silico Models
Creating a dependable in silico model involves several critical components, including data collection, feature selection, algorithm determination, and rigorous validation.
Data Collection and Quality
The foundation of any robust model is high-quality data. Researchers must begin by gathering extensive datasets that accurately represent the biological systems and the molecules of interest. This data can come from various sources, including experimental results, publicly available databases such as PubChem and ChEMBL, or proprietary company data.
Once the data is collected, it is essential to ensure its quality. Data curation processes such as cleaning, normalization, and standardization are necessary to eliminate inconsistencies and biases that could compromise the model's performance. Furthermore, employing methods like cross-validation and data augmentation helps in bolstering the robustness of the data set. This ongoing commitment to data quality will ultimately enhance the model's predictive accuracy and reliability.
Machine Learning for Biomarker Discovery in Cancer TherapeuticsFeature Selection and Engineering
Following data collection, the next step in building a robust model is selecting relevant features that will inform the model’s predictions. Feature selection involves identifying the most informative variables that contribute to the model's target outcome, such as drug efficacy or toxicity. This process can involve statistical methods, machine-learning techniques, or even domain knowledge from medicinal chemistry.
Feature engineering, on the other hand, refers to creating new input variables derived from the existing data. By generating additional meaningful features, researchers can significantly improve the predictive power of their models. It’s essential to strike a balance between complexity and interpretability in feature selection and engineering since overly complex models can lead to overfitting, where the model performs well on training data but fails to generalize to new, unseen data.
Algorithm Selection and Model Construction
The type of algorithms chosen for building in silico models can drastically impact their success. Several machine learning techniques and statistical tools can be employed, including linear regression, support vector machines, random forests, and neural networks. The choice of algorithm depends on various factors, such as the nature of the data, the specific goals of the study, and the required level of interpretability.
Moreover, employing an ensemble approach, where multiple models are used in conjunction to make predictions, can enhance the robustness of the results. This method combines the strengths of different algorithms to produce more accurate outcomes. However, it also increases the model’s complexity, necessitating careful validation to ensure reliability.
Challenges and Solutions in Implementing AI for Drug DiscoveryValidation Techniques for In Silico Models

Validation is a critical step in establishing the credibility of any predictive model. It involves testing the robustness of the model against independent datasets to assess its predictive power and generalizability.
Cross-Validation
Cross-validation is one of the most common validation techniques used in model development. This method involves dividing the dataset into subsets, training the model on some subsets, and validating it on others. A popular variant is k-fold cross-validation, where the dataset is split into k equally-sized segments. The model is trained k times, with each segment serving as the validation set once. This technique helps in ensuring that the model retains its predictive power across various data subsets, thus minimizing the likelihood of overfitting.
External Validation
Beyond cross-validation, external validation is crucial for ascertaining a model’s applicability in real-world scenarios. This process requires testing the model on entirely independent datasets that were not involved in the training phase. By validating the model in various contexts and against different datasets, researchers can establish confidence in its predictive capabilities and enhance its credibility when presented to regulatory bodies.
Using ML Algorithms for Predicting Side Effects in MedicationsPerformance Metrics
To evaluate model performance comprehensively, researchers must employ various performance metrics. Common metrics include accuracy, precision, recall, F1-score, and area under the receiver operating characteristic (ROC) curve (AUC-ROC). Each of these metrics provides insight into specific aspects of model performance, allowing researchers to find a balance between sensitivity and specificity based on their project’s goals.
Impacts of Robust In Silico Models on Drug Discovery
The implementation of robust in silico models impacts several aspects of drug discovery, from preclinical research to regulatory approval.
Cost Efficiency and Time Savings
One of the most immediate benefits of using robust in silico models is the substantial cost and time savings they facilitate. Traditional drug development can take over a decade and billions of dollars to complete. By leveraging computational techniques at the early stages of discovery, researchers can prioritize the most promising candidates and eliminate compounds that are unlikely to succeed long before escalating resource expenditure.
Enhanced Predictive Power
Robust in silico models can significantly improve the predictive power of drug candidates, enabling researchers to forecast efficacy, safety, and potential side effects more accurately. This proactive approach allows for the rapid optimization of drug compounds by identifying structural features that enhance activity while minimizing toxicity.
Regulatory Acceptance and Compliance
Given the increasing reliance on computational methods in drug development, regulatory bodies have begun to recognize and accept robust in silico models as viable tools in the submission process. By adhering to best practices in model creation and validation, researchers can ensure their in silico predictions stand up to scrutiny, thereby facilitating smoother application processes and timely approvals.
Conclusion
The burgeoning field of in silico drug discovery is poised to revolutionize the pharmaceutical industry through the development and implementation of robust predictive models. Emphasizing the significance of data quality, feature engineering, algorithm selection, and rigorous validation can enhance the reliability and applicability of these models, ultimately benefiting researchers and patients alike.
By incorporating machine learning and artificial intelligence into the drug discovery pipeline, we unlock unprecedented opportunities for innovation. However, the success of these approaches hinges on our ability to establish scientifically sound frameworks for model formulation and validation. As we continue to advance in this domain, the fortitude of our models will play a pivotal role in shaping the future of medicine and improving global health outcomes.
In summary, embracing a holistic view of model creation and validation will empower researchers to navigate the ever-evolving landscape of drug discovery with confidence, paving the way for the development of groundbreaking pharmaceuticals that meet the needs of modern medicine.
If you want to read more articles similar to Creating Robust Models for In Silico Drug Discovery Validation, you can visit the Drug Discovery category.
You Must Read