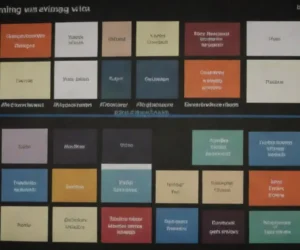
Customizing Marketing Strategies with ML-Driven Customer Segmentation
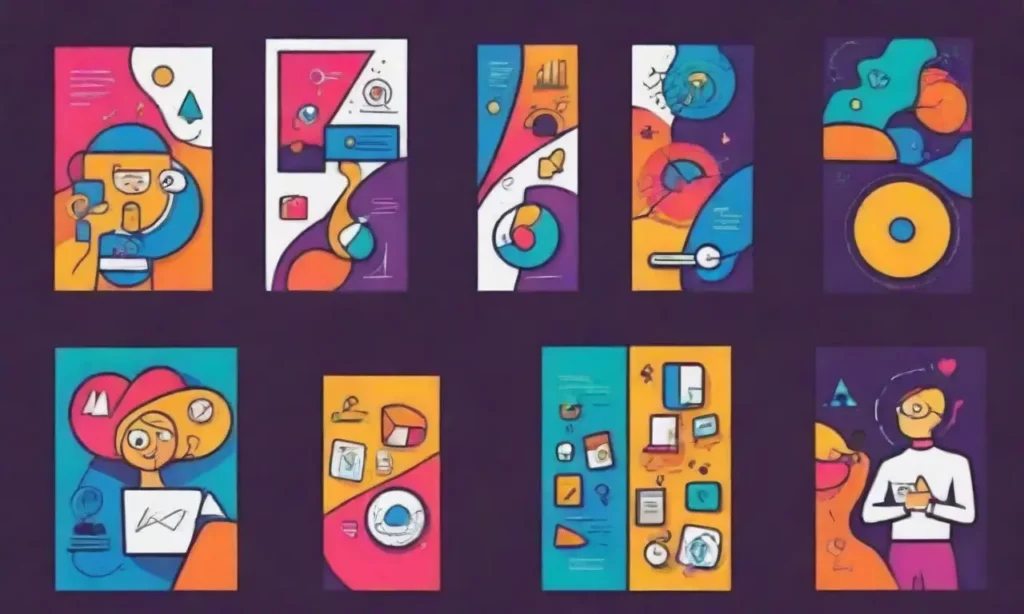
Introduction
The rise of machine learning (ML) technologies has revolutionized the landscape of marketing strategies. Companies today have access to vast amounts of data that lead to insights far beyond traditional marketing methods. Customer segmentation, the practice of dividing a customer base into distinct groups based on various criteria, is now enhanced significantly through machine learning. By leveraging ML algorithms, businesses can gain a deeper understanding of their customers, uncover hidden patterns, and tailor marketing efforts uniquely suited to each segment.
This article aims to delve into the importance and mechanics of using ML-driven customer segmentation to customize marketing strategies effectively. We will explore how machine learning models analyze vast datasets to identify characteristics and behaviors of different customer segments, refocusing marketing strategies based on these insights. We will discuss key methodologies, benefits, challenges, and future trends related to this advanced approach to customer-driven marketing.
Understanding Customer Segmentation
Customer segmentation is a critical marketing strategy that allows businesses to categorize customers based on specific criteria. Traditionally, companies segmented their audiences based on demographic data such as age, gender, location, and purchase history. However, this approach often fails to capture the nuanced behaviors and preferences of individual customers. Herein lies the role of machine learning, which offers unprecedented opportunities for refining and revolutionizing how businesses approach segmentation.
Types of Customer Segmentation
There are multiple types of customer segmentation, and adopting a machine learning framework enables businesses to tackle complex segmentation more effectively. The commonly recognized categories include:
Adaptations of Customer Segmentation in E-Commerce through MLDemographic Segmentation: This is the most basic form and involves segmenting customers based on easily identifiable metrics such as age, gender, income level, education, and occupation. While these metrics are essential, they often do not reveal the underlying motivations driving customer behavior.
Geographic Segmentation: This focuses on segmenting customers based on their geographical location. Understanding regional preferences allows marketers to customize product offerings and messaging to resonate specifically with local clientele. However, geographic segmentation may overlook other pertinent factors like interests and behaviors.
Behavioral Segmentation: Perhaps one of the most critical segments, behavioral segmentation categorizes customers based on their interactions and behaviors with the brand—such as purchase history, product usage, and brand loyalty. This type can help identify high-value customers and tailor experiences based on their behavioral patterns. By employing ML algorithms, businesses can analyze this data at scale, using advanced analytics to understand behaviors dynamically.
Psychographic Segmentation: This dives deeper into the personalities, values, interests, and lifestyles of customers. Although it may be less common, psychographic segmentation plays a vital role, particularly in creative marketing campaigns that resonate emotionally with customers. Combining psycho-graphic data with ML can uncover consumer motivations that traditional methods might miss.
Exploring Cross-Selling Opportunities through Customer Segmentation
The Role of Machine Learning in Customer Segmentation
Machine learning introduces advanced analytical techniques that remove the limitations of traditional segmentation approaches. ML algorithms, particularly clustering algorithms (e.g., K-Means, hierarchical clustering), can identify customer segments based on multiple attributes and behaviors concurrently. By processing vast datasets, these algorithms can discern intricate patterns and relationships that are otherwise undetectable.
A prime example of a machine learning model's application in customer segmentation is the RFM (Recency, Frequency, Monetary) approach. By analyzing how recently customers made a purchase, how frequently they buy, and the amount they spend, businesses can cluster their audience into different segments with tailored strategies. Moreover, as new data comes in, machine learning allows for continual refinement of segments without manual oversight, ensuring that marketing strategies remain relevant and effective.
Benefits of ML-Driven Customer Segmentation
The application of ML-driven customer segmentation offers numerous advantages over traditional methods. Enhanced customer understanding is at the forefront, enabling businesses to offer personalized marketing experiences. With precise segments in place, companies can create targeted marketing campaigns that resonate more profoundly with different groups.
Enhanced Targeting and Personalization
In a marketplace saturated with homogenized marketing messages, standing out is imperative. Utilizing ML-powered segmentation achieves extensive precision in targeting. Marketing campaigns tailored to specific customer characteristics tend to yield higher engagement rates. For instance, an online retail store can identify which segments respond best to discounts for various products and adjust their offerings accordingly, ensuring they reach the right audience at the right time.
Analyzing Sentiment Data for Enhanced Customer SegmentationPersonalization extends beyond mere discounts; it encompasses delivering customized content, suggestions, and recommendations based on individual preferences. By examining user behavior patterns, machine learning algorithms can suggest relevant products, which not only drives sales but also enhances customer satisfaction and loyalty.
Increased Efficiency in Marketing Strategies
Adopting a machine-learning approach to customer segmentation significantly increases marketing efficiency. In traditional segmentation, marketers often relied on a trial-and-error methodology to identify and reach their target audience. However, through the automatic, data-backed identification of customer segments, businesses can reduce wasted ad spend and allocate resources more effectively.
Moreover, ML-driven segmentation enables ongoing optimization. As customer preferences evolve or market trends shift, machine learning models can process new data and revise segments accordingly, allowing businesses to adapt swiftly without undergoing tedious manual analysis. This agility ensures brands remain competitive and engaged with customers in a rapidly changing marketplace.
Improved Customer Retention and Loyalty
Organizations that effectively employ ML-driven customer segmentation can expect to see notable improvements in customer retention and loyalty. By developing a solid understanding of their customers’ needs and tailoring marketing messages accordingly, brands foster deep emotional connections with their audience. Personalization makes customers feel valued—leading to higher purchase frequency and loyalty.
Real-Time Data Processing for Dynamic Customer SegmentationAdditionally, ML algorithms can help identify customers at risk of churning by analyzing behavioral signals indicating dissatisfaction or disengagement. Here, proactive engagement strategies can be implemented, such as personalized outreach, special promotions, or opportunities for feedback, ultimately preventing customer loss.
Challenges in ML-Driven Customer Segmentation

While the potential of ML-driven customer segmentation is immense, there are challenges and hurdles that organizations must navigate. Notably, complications can arise in data collection, model training, and the ethical implications surrounding customer data privacy.
Data Quality and Accessibility
One of the foremost challenges is ensuring data quality and accessibility. Machine learning relies heavily on quality data, as inaccuracies or missing data points can skew insights. Organizations must invest in solid data governance practices, ensuring datasets are cleaned, normalized, and updated consistently. Furthermore, obtaining comprehensive data from disparate sources can be cumbersome, particularly for smaller businesses with limited resources.
Custom Metric Development in Customer Segmentation via MLEnsuring data privacy and compliance with regulations such as GDPR is crucial, as companies need to balance effective data collection strategies with customer trust. When machine learning practices infringe on privacy, consumer backlash can occur, resulting in damaged brand reputation.
Expertise and Model Complexity
Implementing ML-driven segmentation also requires a level of expertise that not all companies possess. Developing effective machine learning models demands specialized skills in data science and analytics, along with familiarity with machine learning concepts and algorithms. This can create a barrier for some organizations, particularly small to medium-sized enterprises, lacking the technical expertise or budget to invest in such capabilities.
Moreover, the complexity of certain machine learning algorithms might lead to challenges in interpretability. Organizations need to understand the fundamentals of how models derive conclusions to take appropriate actionable steps. Creating transparency in these processes can build trust both internally and with consumers.
Integrating with Existing Systems
Finally, integrating machine learning models into existing customer relationship management (CRM) or marketing systems may prove difficult. Legacy systems may lack the infrastructure necessary to support advanced analytics, necessitating potential upgrades or replacements.
Role of Market Basket Analysis in Customer Segmentation ModelsSeamless integration of data sources and aligning marketing strategies with insights gleaned from ML algorithms require careful planning and implementation. Companies must also train their personnel on how to leverage these technologies effectively to witness maximum returns on investment.
Conclusion
Customizing marketing strategies through ML-driven customer segmentation stands as one of the most transformative approaches businesses can adopt in today's competitive landscape. Through a nuanced understanding of customer behaviors, preferences, and needs, machine learning empowers organizations to create tailored marketing campaigns that resonate meaningfully with their target audiences. This not only enhances customer engagement but also drives sales, retention, and brand loyalty.
However, as we explored, the journey to effective segmentation is not without its challenges. Ensuring data quality, navigating technical barriers, and addressing privacy and integration concerns are all essential facets that organizations must consider. By approaching these obstacles thoughtfully and strategically, companies can fully harness the potential of ML-driven customer segmentation, positioning themselves for success in an increasingly data-centric marketing world.
The future of marketing lies in the adoption of advanced technologies, and machine learning will be at the forefront of this revolution. Businesses that embrace these innovations will not only improve their marketing efforts but also create meaningful connections with customers, setting the stage for unmatched growth and success in the years to come.
If you want to read more articles similar to Customizing Marketing Strategies with ML-Driven Customer Segmentation, you can visit the Customer Segmentation category.
You Must Read