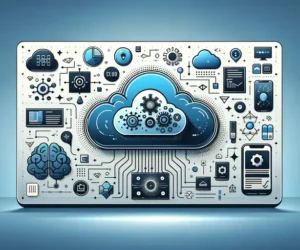
Ethical Considerations in Machine Learning for Financial Trading
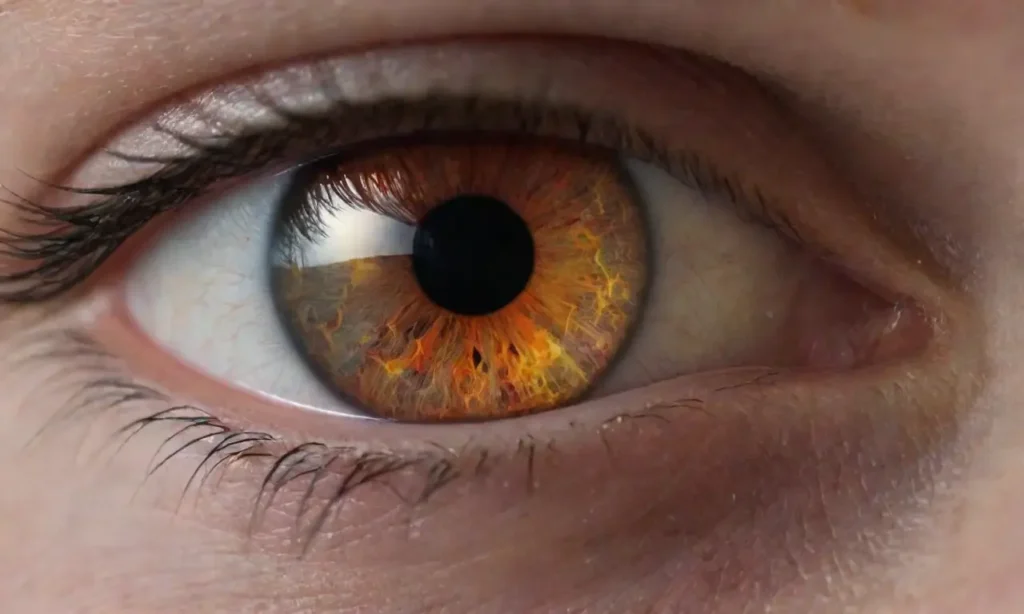
Introduction
In recent years, the rise of machine learning (ML) in the financial sector has sparked widespread excitement and concern. Financial trading, long dominated by human intuition and experience, is increasingly turning to advanced algorithms capable of analyzing vast datasets to inform buy and sell decisions. As these technologies advance, they not only promise to enhance efficiency and profitability but also raise important ethical considerations. The responsible deployment of machine learning in financial trading is crucial to avoid pitfalls that could undermine market integrity and societal trust.
This article will delve deep into the ethical considerations surrounding the use of machine learning in financial trading. We will explore several key themes, including algorithmic bias, transparency, accountability, and the potential for systemic risk. Through these discussions, we aim to shed light on the underlying challenges that professionals must address as they integrate advanced technologies into their trading strategies.
The Impact of Algorithmic Bias in Financial Trading
Algorithmic bias refers to the unintentional prejudices embedded within machine learning models, which can result from various factors, including skewed training data, flawed algorithms, or inadequate representation. In the context of financial trading, the stakes are particularly high. An algorithm trained on biased historical data may reinforce existing inequalities, leading to suboptimal investment strategies that favor certain assets or market segments over others.
Understanding the Roots of Algorithmic Bias
Generally speaking, algorithmic bias can emerge in different ways: it can be due to historical data that reflects past inequalities, or the algorithms themselves may favor certain types of information. For example, an ML model that predominantly uses past trading performance from a limited set of companies may consequently overlook the potential of emerging firms in diverse sectors or regions. This can exacerbate market inefficiencies and lead to a lack of innovation, as ongoing investment might overly favor established players at the expense of newer entrants.
Machine Learning-Driven Trading Platforms: Features and BenefitsMoreover, biases in algorithmic trading decisions can have broader societal consequences. If a particular demographic or group consistently receives inferior trading conditions, it can lead to broader economic disparities, eroding public trust in financial markets. This is especially significant considering that many retail investors are increasingly turning to algorithm-driven platforms for trading, hoping to level the playing field against institutional players.
Mitigating Algorithmic Bias
Addressing algorithmic bias is paramount in developing ML systems for financial trading. One way to mitigate bias is through diverse datasets that accurately represent the multiple facets of the trading environment. An inclusive dataset will allow models to make more equitable decisions and reduce the risk of market distortion. Techniques such as oversampling underrepresented data or employing adversarial training — where models learn from their errors — can also help create fairer algorithms.
Additionally, ongoing monitoring and evaluation of ML models in use is necessary. Detecting biases post-deployment is critical, and financial institutions should adopt best practices in model governance. This involves establishing frameworks for regular audits and updates to ensure that the algorithms adapt to market changes and remain fair and effective over time.
The Challenge of Transparency in Machine Learning Models
Transparency plays an essential role in building trust with stakeholders, and this becomes even more important when deploying machine learning algorithms in financial trading. Traditional algorithmic trading often operates under a veil of complexity, making it difficult for stakeholders to understand how decisions are made.
Predictive Analytics in Trading: Machine Learning Applications OverviewThe Need for Explainability
The lack of explainability in machine learning models poses a risk not only to individual investors but also to the broader market. When a trading algorithm makes rapid decisions based on data, but stakeholders cannot understand the reasoning behind those decisions, it creates an environment of distrust. Moreover, not understanding the inner workings can lead to regulatory challenges and compliance issues, as financial institutions may struggle to justify model outputs during audits or in legal contexts.
To address these challenges, the integration of interpretable algorithms that provide insights into how decisions are made is critical. Techniques such as SHAP values (SHapley Additive exPlanations) can help demystify the decision-making processes of more complex models, providing clearer rationale for investment choices and risk assessments. Transparency in machine learning not only aids in regulatory compliance but also fosters a healthier relationship between traders and clients, reinforcing confidence in the integrity of the financial markets.
Building a Culture of Transparency
Encouraging a culture of transparency within financial institutions is as vital as technological advances. Organizations should cultivate an environment where employees feel empowered to question and discuss algorithmic outputs, ensuring mistakes can be identified and corrected swiftly. Moreover, financial regulators are increasingly emphasizing the need for transparency around algorithmic trading practices. Aligning with these regulatory expectations can be a competitive advantage for firms willing to embrace transparency, as they will be better prepared for compliance and less susceptible to reputational risks in the event of controversies.
Accountability and Responsibility in ML Trading
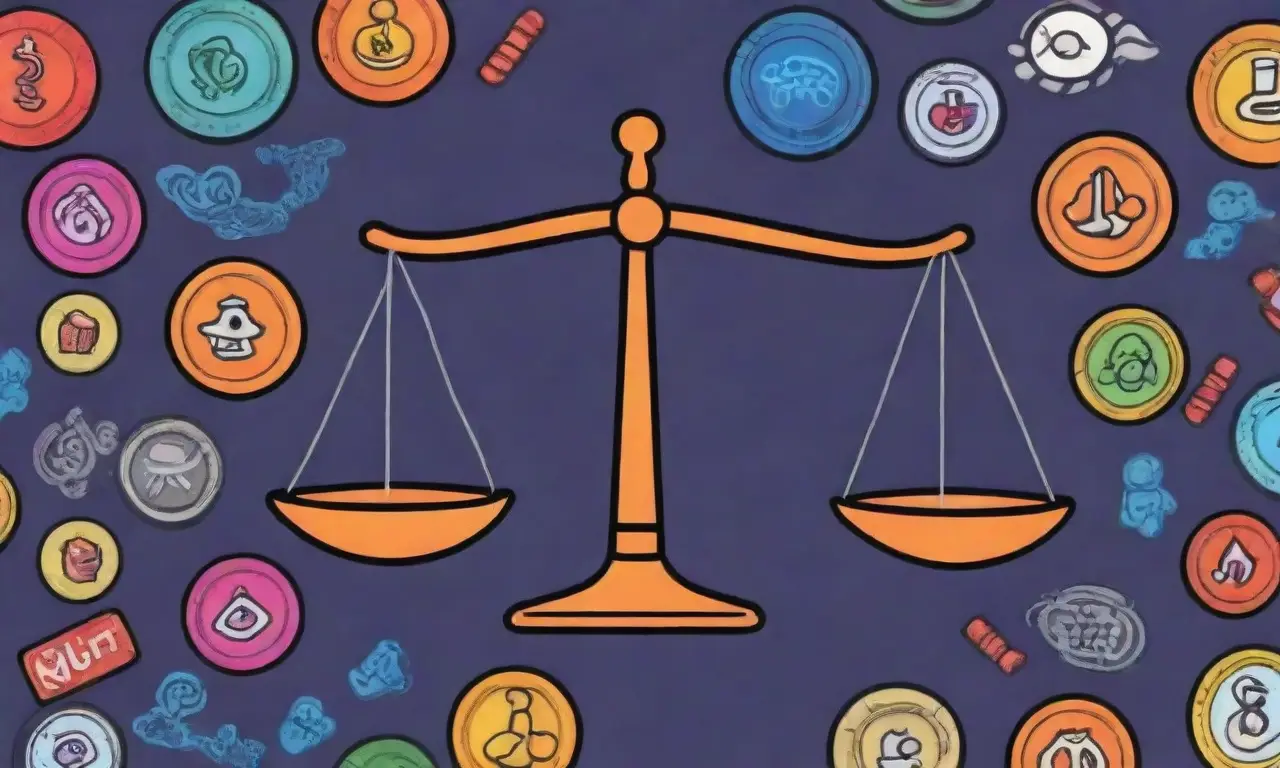
In traditional financial markets, accountability is relatively clear-cut. When a trader makes a poor decision, they are usually held responsible. However, the introduction of machine learning in trading complicates matters significantly. Who is held accountable when an algorithm behaves unexpectedly, leading to financial losses or market disruptions?
Defining Accountability
In the context of machine learning, defining accountability involves establishing clear lines of responsibility throughout the development and deployment of these algorithms. It may not be sufficient to place blame solely on technologists; instead, accountability should extend to executives who advocate for the use of machine learning, data scientists responsible for the algorithm design, and compliance teams who must ensure adherence to regulations.
Understanding the multifaceted nature of responsibility in complex systems is vital. Financial firms need to develop policies that clearly outline who is accountable for algorithmic decisions, along with procedures to investigate when algorithms produce unexpected results. Such a framework not only encourages responsible AI behavior but also prepares the institution for potential liability in the event of adverse outcomes.
Creating an Ethical Framework
Establishing an ethical framework for machine learning in financial trading can help guide decision-making processes. This framework should prioritize ethical considerations, such as fairness, accountability, and transparency, embedding these principles into the company culture. In addition, regular training on ethical practices for employees involved in machine learning can cultivate an environment where ethical implications are constantly contemplated and addressed.
Regulatory bodies also play a vital role in this realm. By introducing guidelines specific to machine learning practices in trading, they can hold firms accountable for their technology-driven decisions. Without such oversight, the potential for irresponsible trading behavior escalates, leading to consequences that can impact entire markets.
The Risk of Systemic Issues through Automated Trading
As financial markets increasingly rely on automated trading strategies powered by machine learning, the specter of systemic risk looms. The interconnected nature of global markets means that a malfunctioning algorithm or widespread bias can have devastating consequences, resulting in flash crashes or severe market instability.
Understanding Systemic Risks
Systemic risk refers to the possibility that an event at the company level could trigger severe instability or collapse the entire financial system. In automated trading, multiple firms often use similar algorithms and trading strategies simultaneously. If one firm's algorithm fails or reacts poorly to market volatility, it could lead to a cascading effect across multiple institutions, significantly impacting liquidity and market prices.
Flash crashes, for example, are exacerbated by the rapid response of algorithms to market events. If many algorithms initiate sell orders simultaneously based on predefined conditions, they can exacerbate price declines and trigger sell-offs across the board. In such scenarios, engineers and data scientists face the difficult challenge of designing algorithms that can effectively manage market fluctuations without overreacting.
Strategies to Mitigate Systemic Risk
To combat potential systemic risks associated with machine learning in trading, financial institutions and regulators could implement several strategies. One essential approach involves introducing circuit breakers that temporarily halt trading during periods of excessive volatility. This allows the market time to stabilize before resuming activity, helping to prevent catastrophic outcomes.
Moreover, increasing collaboration between firms and regulators can foster a shared understanding of potential systemic risks inherent in automated trading systems. Establishing industry standards for the ethical use of machine learning can help ensure that algorithms do not inadvertently create liabilities for the financial system. Furthermore, ongoing monitoring of market behavior can help detect troubling trends that may warrant intervention.
Conclusion
As machine learning continues to revolutionize the landscape of financial trading, addressing ethical considerations becomes essential for maintaining market integrity and public trust. The challenges posed by algorithmic bias, the need for transparency, accountability complexities, and the potential for systemic risk all require thoughtful approaches and collaborative solutions.
Implementing these strategies fosters a culture of ethics and responsibility, equipping financial institutions to navigate the complexities of machine learning. By prioritizing these values, firms can mitigate the risks associated with technological advancements while enhancing their trading capabilities, ensuring they remain competitive in a rapidly evolving market landscape.
In integrating ethics into the fabric of machine learning in financial trading, we point toward a future where technology complements human judgment, promoting a healthier and more resilient financial ecosystem. The journey of responsible AI will require vigilance, adaptability, and a commitment to the principles of fairness and accountability, ensuring that as we embrace innovation, we do so with integrity and foresight.
If you want to read more articles similar to Ethical Considerations in Machine Learning for Financial Trading, you can visit the Financial Trading Algorithms category.
You Must Read