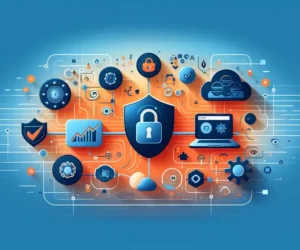
Examining the User Experience of Music Recommendation Algorithms
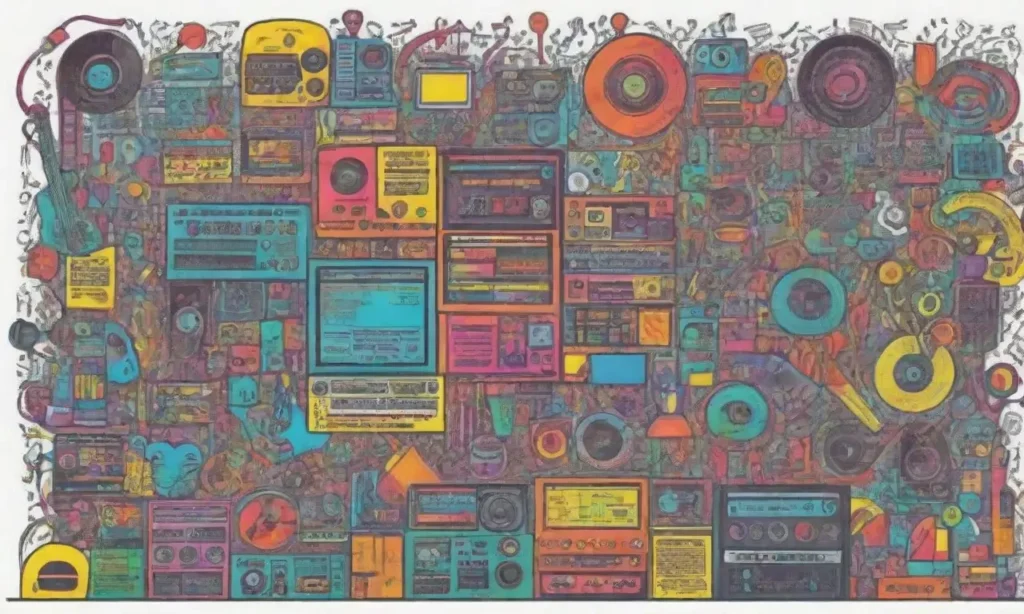
Introduction
The rise of streaming services has drastically transformed the way people consume music. Gone are the days when individuals would buy entire albums; they now have access to millions of tracks at the click of a button. With this unprecedented access, the need for sophisticated music recommendation algorithms has emerged. These algorithms are designed to curate personalized playlists and suggest new tracks based on users' tastes, which can enhance or detract from the overall user experience.
In this article, we will examine how music recommendation algorithms function, their impact on user experience, and the challenges they face. We will delve into the underlying technology, analyze user interactions, consider ethical implications, and suggest potential enhancements. By scrutinizing these aspects, we hope to gain a clearer understanding of how these systems can better serve users and create a more enriched musical journey.
Understanding Music Recommendation Algorithms
Music recommendation algorithms operate on complex systems that analyze user data to predict what songs a user is likely to enjoy. These algorithms can be broadly categorized into three main types: collaborative filtering, content-based filtering, and hybrid systems. Each of these methods has its own strengths and weaknesses, which we will delve into below.
Collaborative Filtering
Collaborative filtering is one of the most commonly used techniques in recommendation systems. It relies on the idea that users who have similar tastes in the past will continue to have similar tastes in the future. This method aggregates data from multiple users to make recommendations. For instance, if User A and User B have a high degree of overlap in the songs they have listened to and rated positively, the system can recommend other songs from User A's listening history to User B, and vice versa.
The Role of Feedback Loops in Enhancing Recommendation SystemsWhile collaborative filtering can effectively introduce users to new music, it faces some inherent challenges. These include the cold start problem, where new users do not have sufficient data for the system to make accurate recommendations, and the popularity bias, where the system favors more popular songs over niche or lesser-known artists. These limitations can lead to a homogenized listening experience, wherein users might miss out on unique tracks that fit their taste profile.
Content-Based Filtering
In contrast, content-based filtering uses information about the items themselves to make recommendations. This could involve analyzing metadata such as genre, tempo, key, and instrumentation of songs. For example, if a user frequently listens to upbeat pop songs, the recommendation algorithm will suggest similar tracks based on their musical attributes.
While content-based filtering allows for more precise recommendations according to users' explicit tastes, it can also create a filter bubble. A filter bubble occurs when users are presented with a narrow range of options that reinforce their existing preferences, potentially stunting musical diversity and exploration. This could lead to users missing out on a wealth of genres, artists, and cultural expressions that they might actually enjoy.
Hybrid Systems
To counter the limitations of both collaborative and content-based filtering, many streaming services have turned to hybrid systems. These systems combine elements of both methods to create a more balanced and comprehensive recommendation approach. By taking into account both user behavior and the inherent characteristics of music, hybrid systems can provide more nuanced and varied options.
How to Measure Success in Music Recommendation Systems EffectivelyFor instance, Spotify’s Discover Weekly playlist is a prime example of how hybrid algorithms work. The system analyzes a user’s past listening history alongside the preferences of similar users while also considering the characteristics of the songs themselves. The result is a playlist that is not only personalized but also refreshingly diverse. This way, users are exposed to new artists and genres, broadening their musical horizons and enriching their overall experience.
The Impact on User Experience
The implementation of music recommendation algorithms has a profound impact on user experience, shaping the way listeners interact with music and the streaming platforms themselves. By providing tailored playlists and suggestions, these algorithms serve to enhance user engagement and satisfaction in several pivotal ways.
Personalization and Discovery
One of the most compelling aspects of music recommendation algorithms is their ability to provide an unparalleled level of personalization. When users log onto their preferred streaming service, they are often greeted with playlists tailored specifically to their tastes. This instant curation eliminates the often time-consuming process of searching for songs and allows users to dive straight into listening.
Moreover, music recommendation algorithms excel in introducing users to new artists and genres they may not have encountered otherwise. This aspect of music discovery is crucial, especially for music enthusiasts looking to explore beyond their established playlists. By serving as cultural curators, these algorithms can create a richer experience that encourages users to continue exploring, all while feeling understood and catered to. The thrill of stumbling upon a hidden gem can often lead to lasting connections with new music and artists.
Ethical Considerations in Music and Video Recommendation AlgorithmsUser Control and Interactivity
User experience is further enhanced by incorporating elements of control and interactivity. Most streaming platforms allow users to provide feedback on recommended songs, whether through likes, dislikes, or skips. This feedback loop is essential for improving algorithm accuracy over time and fosters a sense of ownership over the listening experience. By actively participating in refining the recommendations, users feel more invested in their musical journeys.
However, it’s important to acknowledge that this interactivity can lead to a paradox of choice. When users are given overwhelming options or inundated with recommendations, the process of choosing what to listen to can become paralyzing. Striking a balance between providing choices and overwhelming users is essential for ensuring a positive user experience.
As music consumption has transitioned to an online environment, the role of social interactions cannot be overlooked. Many platforms have integrated social features, allowing users to share favorite songs and playlists with friends or see what their peers are currently enjoying. This social aspect not only enhances user engagement but also cultivates a sense of community among listeners.
For instance, Spotify’s “Blend” feature allows users to create a collaborative playlist based on the musical preferences of themselves and their friends, reinforcing connections through shared interests. The ability to connect over music can enhance the emotional experience of listening and provide opportunities for users to bond over their shared taste, enriching their enjoyment of the art form further.
Understanding Contextual Bandits in Music Recommendation StrategiesChallenges and Ethical Considerations

Though music recommendation algorithms improve the user experience, they are not without their challenges and ethical implications. As these algorithms become more integrated into users' daily lives, it is crucial to address potential pitfalls to ensure a fair and enjoyable experience for all.
Data Privacy Concerns
One of the foremost concerns associated with recommendation algorithms is data privacy. These systems rely heavily on user data, such as listening habits, demographic information, and even transactional data. Users may not always be aware of the extent to which their information is collected or how it is used, leading to potential discomfort and mistrust.
The necessity for transparency becomes evident. Companies must ensure that users understand what data is being collected, how it is being used, and provide an option for users to control their data preferences. Striking a balance between personalization and privacy is essential, as users are unlikely to engage fully with systems that they do not trust.
Unlocking the Secrets of Algorithmic Music RecommendationsAlgorithmic Bias and Limitations
Another challenge is algorithmic bias. These algorithms reflect the data they are trained on, which can perpetuate existing biases in music distribution and recognition. If the dataset is skewed towards popular genres or well-known artists, users might be less likely to discover music from underrepresented groups or independent artists. This bias can limit diversity within music consumption and result in a much narrower range of music being popularly accepted.
Mitigating this bias is imperative to ensure a more equitable music landscape. Companies can actively work towards diversifying their data sources and incorporating various genres and artists into their recommendation systems, thus encouraging listeners to engage with a broader spectrum of music.
The Future of Music Recommendations
Looking ahead, the landscape of music recommendations will continue to evolve, driven by advancements in technology and shifting user preferences. The integration of artificial intelligence and machine learning will enable even more nuanced understanding of user behavior, resulting in increasingly precise recommendations. Additionally, user-driven inputs and insights could guide the development of more adaptive systems that respond dynamically to taste changes over time.
Moreover, there is a growing trend towards mindful recommendations, where systems not only focus on what users like but also consider the emotional context of listening. This approach acknowledges that music can evoke different feelings and experiences depending on the listener's current mood or situation. By factoring in emotional cues and social contexts, recommendation algorithms could enhance the relevant emotional experience for each user.
Artificial Intelligence in Music Recommendation: Myths and FactsConclusion
In conclusion, music recommendation algorithms have become embedded in the fabric of modern music consumption, greatly influencing user experience and engagement. By employing collaborative and content-based filtering techniques, these systems provide personalized playlists while also introducing users to new and diverse musical offerings. However, there are notable challenges to address, including issues of data privacy and algorithmic bias.
The future of music recommendations lies in leveraging the power of AI and machine learning, fostering greater user interactivity and control while actively combating biases in data. Prioritizing user needs and preferences while maintaining transparency can create a more enjoyable and enriching experience for listeners.
As we further explore the intricacies of music recommendation, it is important for both companies and users to remain vigilant regarding their collective responsibilities. Encouraging ethical practices while celebrating musical diversity can help to create a fairer, more inclusive space for music enthusiasts to connect with the art form they cherish. Ultimately, the user experience of music recommendation algorithms can continue to improve, ensuring that the way we consume music remains not just personalized but profoundly enriching.
If you want to read more articles similar to Examining the User Experience of Music Recommendation Algorithms, you can visit the Music and Video Recommendation category.
You Must Read