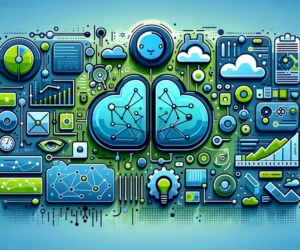
Exploring the Potential of Generative Models in Industrial Design

Introduction
The landscape of industrial design has been dramatically reshaped by technological advancements over the last few decades. Among these innovations, generative models — often powered by artificial intelligence (AI) and machine learning (ML) — have emerged as a revolutionary force. These models enable designers to explore a myriad of possibilities, enhancing creativity while streamlining the design process. By learning from vast datasets, generative models can produce unique designs that meet specific constraints, ultimately pushing the boundaries of innovation and usability.
In this article, we will delve into the growing role of generative models within the framework of industrial design. We will explore their capabilities, the advantages they bring to the design process, as well as the challenges and limitations they may present. By the end, readers will gain a deeper understanding of how generative modeling can reshape industrial design practices and help them envision a future where technology complements human creativity.
Understanding Generative Models
Generative models refer to a class of statistical models that can generate new data that resembles a given dataset. In the context of industrial design, this means using algorithms and computational techniques to engage with data in such a way that new design concepts can be automatically produced. Generative design tools utilize various approaches, including Genetic Algorithms (GA), Neural Networks, and Variational Autoencoders (VAEs), to evolve solutions that are not only innovative but also highly efficient.
The Science Behind Generative Models
At their core, generative models are built on the principles of machine learning. They learn from datasets that contain examples of good design practices, enabling them to understand and replicate the patterns they observe. For instance, a generative network might analyze thousands of chair designs to identify recurring features such as material usage, ergonomic considerations, and aesthetic choices. Once trained, the model can generate new variations that combine these learned distinctions, leading to unique design outputs.
Improving Production Efficiency with AI-Powered Automation ToolsImportantly, generative models can also integrate constraints and specifications provided by the designer. This allows for the creation of solutions tailored to meet specific requirements, such as weight limits, cost constraints, or user ergonomics. By combining creative exploration with computational rigor, generative design offers a promising avenue for tackling complex design problems effectively.
Types of Generative Models
There are several types of generative models widely used in industrial design, each with its own approach and applications. Among these, Generative Adversarial Networks (GANs) have gained particular prominence. GANs consist of two neural networks — a generator and a discriminator — that work against each other to improve the quality of generated data. The generator produces new designs, while the discriminator evaluates them against real-world examples, thereby refining the outputs iteratively.
Another important technique is the Variational Autoencoder (VAE), which continuously encodes data into a lower-dimensional space and decodes it back to its original format. This capability allows designers to explore design components in an intuitive manner. Lastly, systems based on Genetic Algorithms mimic natural evolutionary principles, generating a "population" of design solutions that can be tested and refined over time.
Through these techniques, generative models facilitate a new paradigm in industrial design where creativity is augmented by data-driven insights, potentially leading to solutions that were previously unimaginable.
Advantages of Generative Models in Industrial Design
The integration of generative models into industrial design offers a multitude of advantages that significantly impact the way designers approach their work. One of the most notable benefits is the increase in design efficiency. By automating the exploration of design alternatives and automating repetitive tasks, these tools allow designers to focus more on strategic decision-making and creative thinking. This shift not only speeds up the overall timeline of projects but also enables designers to explore a broader range of possibilities.
Enhancing Creativity Through Exploration
Generative models thrive on diversity and variation, showcasing designs that a human designer may not have considered. When designers work within traditional frameworks, they often subconsciously limit their creativity based on their experiences and knowledge. In contrast, generative models reveal alternative forms, materials, and configurations that challenge existing paradigms and expand the creative palette.
This capability to transcend conventional thinking is particularly beneficial in fields where aesthetics play a key role. By employing generative models, designers can create visually striking solutions that are not only pragmatic but also strikingly innovative. For example, in product design, these models can generate unique shapes and forms that enhance user interaction while also improving functionality.
Optimization and Sustainability
In a world increasingly concerned with sustainability, generative models can play a pivotal role in creating efficient and eco-friendly designs. By analyzing material properties and performance data, these models can suggest configurations that minimize waste and optimize resource usage. This becomes especially crucial in industries like automotive and aerospace, where reducing weight can lead to significant savings in fuel consumption without compromising strength or durability.
Furthermore, generative models can enable faster iterations and testing phases, allowing companies to bring products to market more quickly while ensuring they adhere to both quality and sustainability standards. Sustainability is no longer just a requirement but a competitive advantage, and generative design is uniquely positioned to advance these goals.
Challenges and Limitations of Generative Models

Despite the numerous advantages offered by generative models in industrial design, several challenges and limitations remain. One of the primary concerns is the need for robust datasets. The quality and diversity of the training data directly impact the outcomes produced by generative models; inadequate or biased datasets can lead to suboptimal or non-viable designs. Designers need to ensure they are inputting comprehensive and representative data to achieve accurate results.
The Role of Human Oversight
While generative models can produce innovative and optimized designs, they are not a replacement for human intuition and judgment. The interpretative aspect of design remains essential, as designers bring cultural, emotional, and aesthetic considerations that machines cannot grasp. It is vital that designers remain integral to the decision-making process, using generative models as collaborators rather than replacements. Relying too heavily on machine outputs without human oversight can lead to designs that fail to address user needs or resonate emotionally with consumers.
Additionally, human oversight ensures ethical considerations are addressed. As with any form of AI, generative models can inadvertently perpetuate existing biases if not monitored carefully. For instance, if the training dataset reflects a limited perspective, the generated designs may also lack broad appeal or fail to represent the diversity of potential users. Therefore, maintaining a balance between machine-generated suggestions and human creativity is critical.
Technical Barriers and Adoption Challenges
Finally, the integration of generative models into existing design workflows may pose additional technical barriers. These tools require not only robust computing power but also familiarity with software platforms and programming languages that may be new to designers. As a result, potential users may face a steep learning curve, creating resistance to adoption, especially in traditional design environments.
Moreover, solving specific design problems requires clear communication between teams and disciplines. Misalignment between software capabilities and design objectives can lead to frustration and inertia, slowing progress. To maximize the potential of generative models, companies must invest in training, provide adequate support, and foster a culture of innovation that empowers designers to experiment with these new tools.
Conclusion
The advent of generative models in industrial design represents a transformative shift, merging technological capabilities with human creativity in unprecedented ways. By embracing these innovations, designers can enhance their processes through expanded creativity, improved efficiency, and a focus on sustainability. The potential for generating novel designs that align with user needs while optimizing material usage cannot be understated, marking a crucial evolution in how we approach product development.
However, while generative models offer significant advantages, the challenges of dataset quality, human oversight, and technical barriers cannot be ignored. Successful implementation hinges on a collaborative mindset, where designers actively participate in guiding generative processes. The future of industrial design is undoubtedly interwoven with the continued advancement of generative technologies, but it will require a balanced approach that values both machine-generated insights and human creative intuition.
In conclusion, the exploration of generative models in industrial design is just beginning, and as the technology continues to mature, it promises to bring even more exciting opportunities for innovation. As we continue to navigate this landscape, the blend of artistry and technology will play a critical role in shaping the products of tomorrow, fostering a future where technology and human ingenuity come together to enhance everyday life.
If you want to read more articles similar to Exploring the Potential of Generative Models in Industrial Design, you can visit the Industrial Automation category.
You Must Read