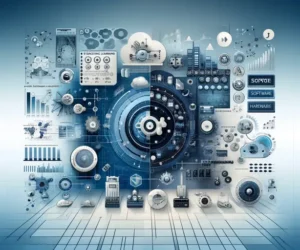
Future Directions for Weather Prediction: Harnessing AI Advances
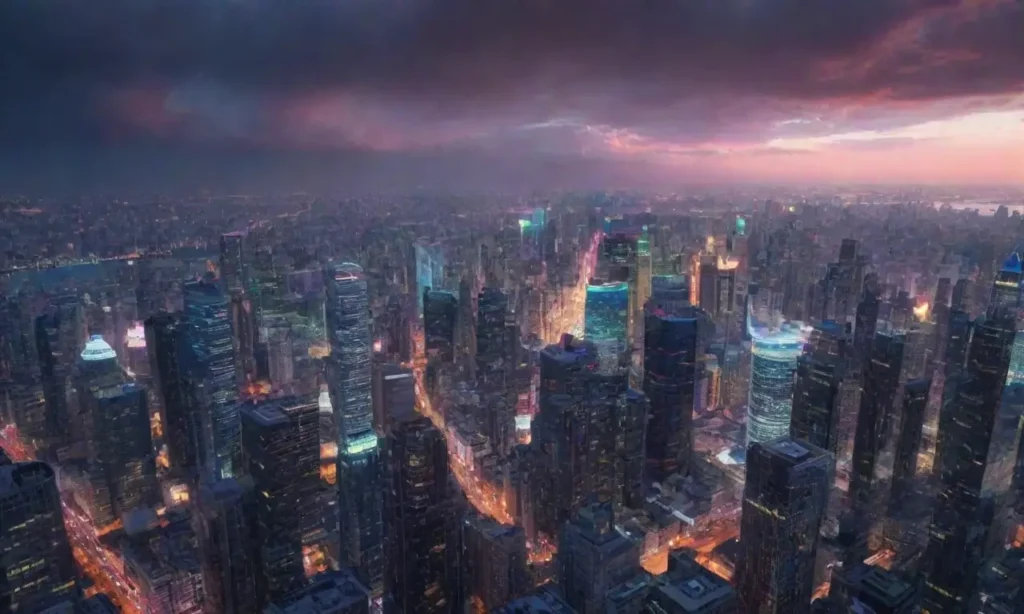
Introduction
Understanding weather patterns and making accurate predictions has always been a crucial endeavor for society, impacting everything from agriculture to disaster management. Over the last few decades, traditional meteorological methods and models have significantly advanced, but the proliferation of data and the complexity of atmospheric phenomena present ongoing challenges. Recently, there has been a growing interest in leveraging advancements in artificial intelligence (AI) and machine learning to improve the accuracy of weather predictions.
This article examines the future directions of weather prediction with a specific focus on how AI can transform this field. We will explore the potential applications of AI, the challenges faced in its implementation, and the innovations on the horizon that promise to enhance our understanding and forecasting of weather systems. By the end of this article, we aim to provide a comprehensive overview of how AI technology can reshape meteorology and thus improve our preparedness for various weather-related challenges.
The Role of AI in Weather Prediction
AI technologies have the potential to revolutionize weather forecasting by improving both speed and accuracy. Traditional meteorological models rely on a set of deterministic equations that describe physical processes in the atmosphere. While these models are mathematically sound, they can be extremely resource-intensive and may not capture all the complexities of weather systems. AI algorithms, specifically machine learning techniques, can analyze massive datasets more efficiently and catch subtle patterns that traditional models may miss.
The main advantage of using AI lies in its ability to learn from vast quantities of historical climate data. By feeding AI systems vast amounts of observational data, including temperature, humidity, wind speed, and pressure, they can identify non-linear relationships that are often obscured in conventional forecasting methods. For instance, deep learning, a branch of AI, can uncover latent structures in data, allowing for predictive insights that can offer super-resolution in local weather forecasting.
Moreover, AI-powered systems can integrate real-time data from various sources, such as satellites, weather stations, and environmental sensors, leading to improved forecasts. The ability to continually adapt and improve predictions based on new incoming data holds great promise. As these systems evolve, researchers believe that AI could lead to the development of highly localized and precise weather models, giving communities better warnings and allowing for tailored responses to impending weather events.
Data Collection and Integration: New Frontiers
The foundation of any forecasting model is data. As we strive to harness AI in weather prediction, one critical area is data collection and integration. The advent of the Internet of Things (IoT) has generated a massive influx of climatic data from sensors placed in various environments, such as urban areas, oceans, and forests. The integration of this data into existing models, coupled with AI analytics, can yield more refined results.
One key innovation in data collection is the use of drone technology. Drones equipped with sensors can gather hyper-localized atmospheric data quickly and efficiently, particularly in areas that are hard to reach due to geographical features. By deploying these drones during extreme weather events, scientists can receive real-time data to improve short-term forecasting accuracy. This integration of drone-captured data into AI models can refine predictions on a micro-scale, ensuring that forecast services are tailored to specific needs.
Another facet is the utilization of crowdsourced weather data. Citizens can contribute data through mobile applications, reporting not only on temperature and precipitation but also on subjective experiences related to weather conditions. AI algorithms could analyze this crowdsourced data to provide immediate updates and localized insights, enhancing traditional forecasting methods and fostering community engagement in meteorological processes.
Additionally, oceanic and atmospheric data are becoming increasingly sophisticated through the use of remote sensing technologies. Satellites can observe large-scale weather patterns and phenomena, such as hurricanes or El Niño events, leveraging AI to process information and issuing timely warnings. The synergy between AI and satellite data can enhance our understanding of evolving systems and offer risk assessments that were previously unattainable.
Challenges in Implementation
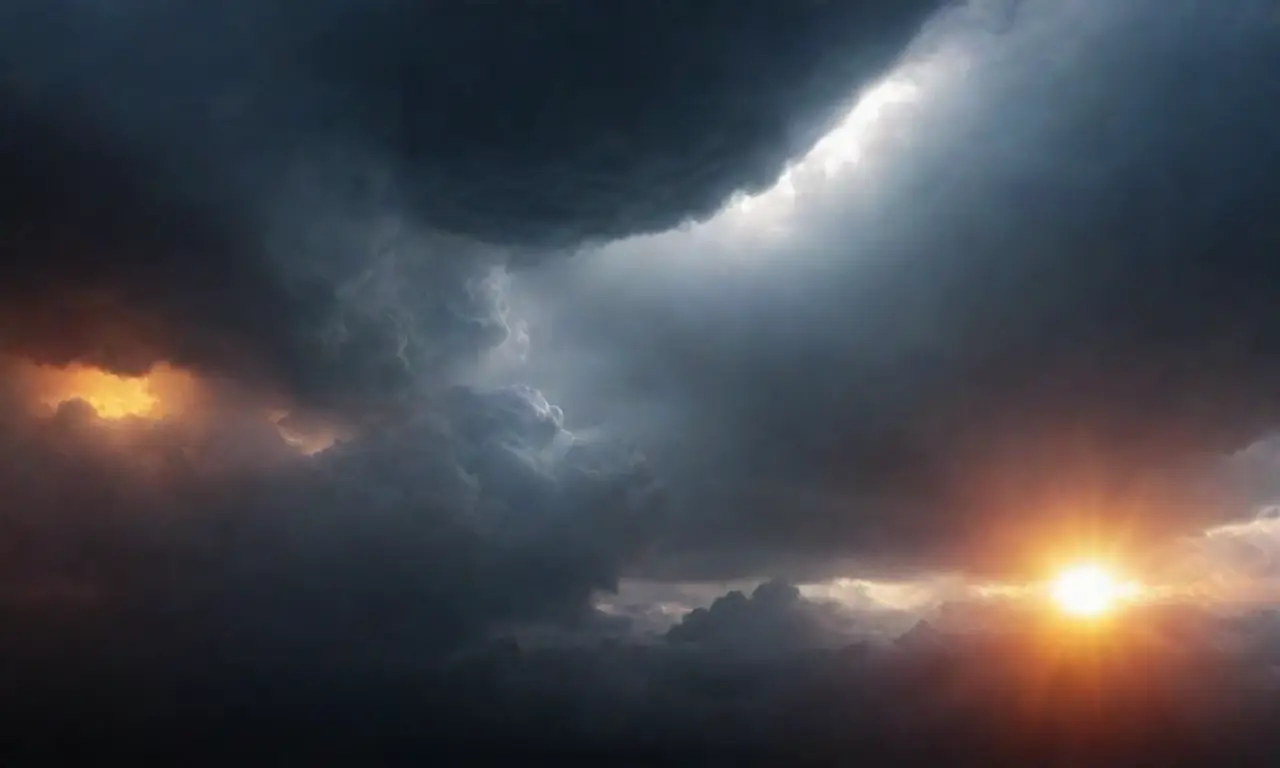
While the promise of AI in weather prediction is exciting, there are several considerable challenges in its practical implementation. One predominant issue is the quality of data. AI algorithms rely on well-curated and extensive datasets for training, and any gaps or inaccuracies in weather data can lead to misleading forecasts. Inconsistent data sources, particularly in remote areas with limited infrastructure, pose a significant hurdle to creating robust AI-powered systems.
Equally important is addressing concerns related to interpretability. Many AI models, especially deep learning networks, operate as “black boxes,” meaning that while they can produce predictions, it can often be difficult to understand how they arrived at those conclusions. This lack of transparency can create skepticism among meteorologists and policymakers, who rely on comprehensible and verifiable models to make decisions regarding public safety, disaster preparedness, and response.
There is also the challenge of scalability. While AI systems can be powerful in isolated applications, developing comprehensive models that can be deployed at different scales—from local to regional to global—remains an ongoing area of research. The need for continuous training and retraining of AI models can also be resource-intensive, especially as the complexity of weather systems evolves with climate change. Economically viable methods to implement these technologies must be explored to ensure that they are accessible to meteorological agencies, regardless of their size or budget.
Innovations on the Horizon
Despite the challenges, numerous innovative avenues are being explored to ensure that AI continues to enhance weather prediction capabilities. One promising trend is the development of neuromorphic computing, a paradigm that mimics the human brain's structure and functionality. By mimicking the way our brains process information, neuromorphic chips can learn from data more efficiently and handle complexity in real-time. This could lead to the creation of smarter weather forecasting models that can operate continuously and improve accuracy in dynamic environments.
Another exciting development is ensemble modeling using AI. Instead of relying on a singular deterministic model, researchers are exploring utilizing multiple models to produce a range of forecasts, which can then be aggregated using machine learning techniques. This ensemble approach acknowledges the inherent uncertainty in weather systems and allows for the generation of probabilistic forecasts that provide more nuanced risk assessments, aiding decision-makers in planning and prevention strategies.
In addition, collaborations between governments, academic institutions, and technology companies are essential to driving forward AI in meteorology. By encouraging an interdisciplinary approach, the combined expertise of data scientists, meteorologists, and environmental scientists can lead to tailored solutions better suited for various applications. Initiatives that promote data sharing and collaborative research will be pivotal in building a more unified and agile weather prediction ecosystem.
Conclusion
The integration of artificial intelligence into weather prediction marks a transformative journey towards enhanced forecasting capabilities. While significant challenges remain, the potential benefits of utilizing AI technologies in meteorology are vast. From improved speed and efficiency in processing weather data to more accurate and localized predictions, AI holds the key to understanding the complexities of our atmosphere in unprecedented ways.
As we look towards the future, ongoing research, innovation, and collaboration will be essential in overcoming existing barriers and fully harnessing AI's power. The ongoing evolution of weather prediction fueled by AI advancements is not just a scientific endeavor; it is a societal necessity aimed at growing safer and more resilient communities in the face of increasingly unpredictable weather patterns stemming from climate change.
In conclusion, embracing the promise of AI in weather prediction requires not only technical advancements but also a commitment to creating inclusive and reliable systems that prioritize transparency, data quality, and accessibility. By ensuring that both technological and ethical frameworks are in place, society can look forward to forecasts that are not only reliable but significantly improve our preparedness for the future.
If you want to read more articles similar to Future Directions for Weather Prediction: Harnessing AI Advances, you can visit the Weather Prediction category.
You Must Read