
Harnessing Transfer Learning for Enhanced Speech Synthesis
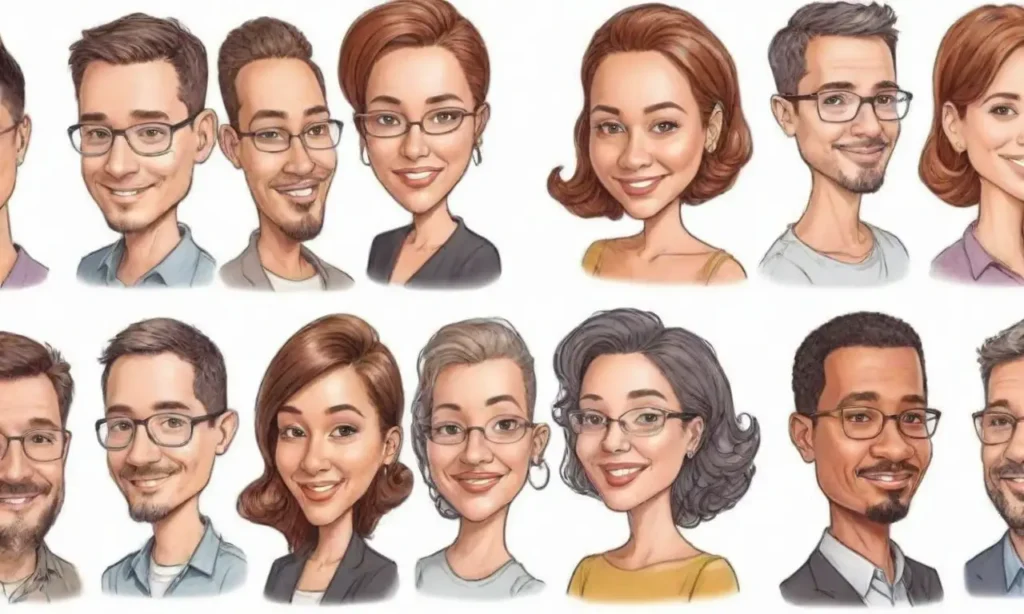
Introduction
In recent years, the advancement of speech synthesis has become increasingly pertinent, reshaping the ways we interact with technology. Speech synthesis refers to the artificial production of human speech, a discipline that has seen remarkable progress thanks to recent technologies such as deep learning. One of the most significant breakthroughs in this field has been the development of transfer learning, a powerful technique that allows models to leverage pre-existing knowledge to enhance their performance on new tasks. This article explores the integration of transfer learning into speech synthesis and how it has the potential to elevate the quality, efficiency, and accessibility of synthetic speech.
This article will delve into the concepts of transfer learning, the underlying mechanisms of speech synthesis, and specific applications where this synergy leads to improved outcomes. In doing so, we aim to not only elucidate the complexities of these technologies but also inspire enthusiasts, researchers, and industry professionals to explore the vast opportunities presented by the fusion of these groundbreaking methodologies. By the end of this discussion, readers will gain a comprehensive understanding of the interplay between transfer learning and speech synthesis, as well as practical implications and future trends in this exciting field.
Understanding Speech Synthesis
Speech synthesis technology has come a long way since its rudimentary beginnings in the mid-20th century, evolving from simple concatenative approaches to sophisticated neural networks. Text-to-Speech (TTS) is the most recognized form of speech synthesis, transforming written text into spoken language. TTS systems can be divided into two main categories: rule-based and data-driven methods.
Rule-Based Approaches
Rule-based systems traditionally relied on phonetic rules and linguistic knowledge to generate speech. These methods often employed a process known as concatenative synthesis, where segments of pre-recorded speech (e.g., phonemes, diphones) were pieced together to form coherent utterances. While effective to some extent, this approach had limitations, chiefly the lack of naturalness and expressiveness in the synthesized speech. Such systems struggled with emotional inflection, cadence, and natural rhythm, resulting in monotone outputs that were often mechanical and far from human-like.
The Impact of AI on the Future of Speech Synthesis TechnologyData-Driven Approaches
The introduction of data-driven approaches marked a significant shift in the field. Leveraging large datasets of recorded speech, researchers began to apply machine learning techniques, particularly neural networks, to create more sophisticated TTS systems. One prominent architecture in this field is WaveNet, developed by DeepMind, which employs deep generative models to produce highly realistic speech waveforms. By learning the statistical relationships between phonetic input and acoustic output, data-driven TTS systems have dramatically improved in terms of naturalness and expressiveness, demonstrating a level of nuance previously deemed unattainable.
However, even with state-of-the-art neural networks boasting unprecedented performance, challenges such as domain adaptation, data scarcity for low-resource languages, and personalized speech synthesis remain significant hurdles requiring innovative solutions.
The Power of Transfer Learning
What is Transfer Learning?
Transfer learning refers to the technique of transferring knowledge gained from one domain or task to enhance the performance of a model on a different but related task. This methodology is especially valuable in scenarios where collecting large datasets is either impractical or prohibitively resource-intensive. For speech synthesis, transfer learning allows models trained on high-resource languages or datasets to adapt their learned features when synthesizing speech in underrepresented languages or specific domains.
Mechanisms of Transfer Learning
The mechanisms of transfer learning typically involve initial training on a large, diverse dataset (called the source domain) and subsequent fine-tuning on a smaller, more specific dataset (referred to as the target domain). By retaining the foundational knowledge acquired during the first training phase, the model can quickly adapt to the target task with minimal additional data, often outperforming models trained exclusively from scratch.
How Speech Synthesis is Transforming Accessibility in TechnologyIn the context of speech synthesis, an example would be training a TTS model on a comprehensive English language dataset before fine-tuning it on a smaller dataset of a less represented language like Swahili or even specific accents within a language. This efficient approach significantly reduces data requirements and enhances the model's ability to replicate unique phonetic and prosodic characteristics specific to the target domain.
Advantages of Transfer Learning in Speech Synthesis
The advantages of incorporating transfer learning into speech synthesis are manifold. Firstly, it streamlines the training process, enabling faster convergence and reducing computational costs. Companies can save precious time and resources that would otherwise be allocated to curating large datasets or extensive training from scratch. Secondly, it imparts robustness and flexibility to the TTS models, allowing them to generalize better across different linguistic contexts, further enhancing their utility. Lastly, transfer learning fosters inclusivity, as it enables the development of high-quality speech synthesis systems for languages or dialects with limited available data, thus broadening the potential applications in diverse cultural settings.
Applications of Transfer Learning in Speech Synthesis
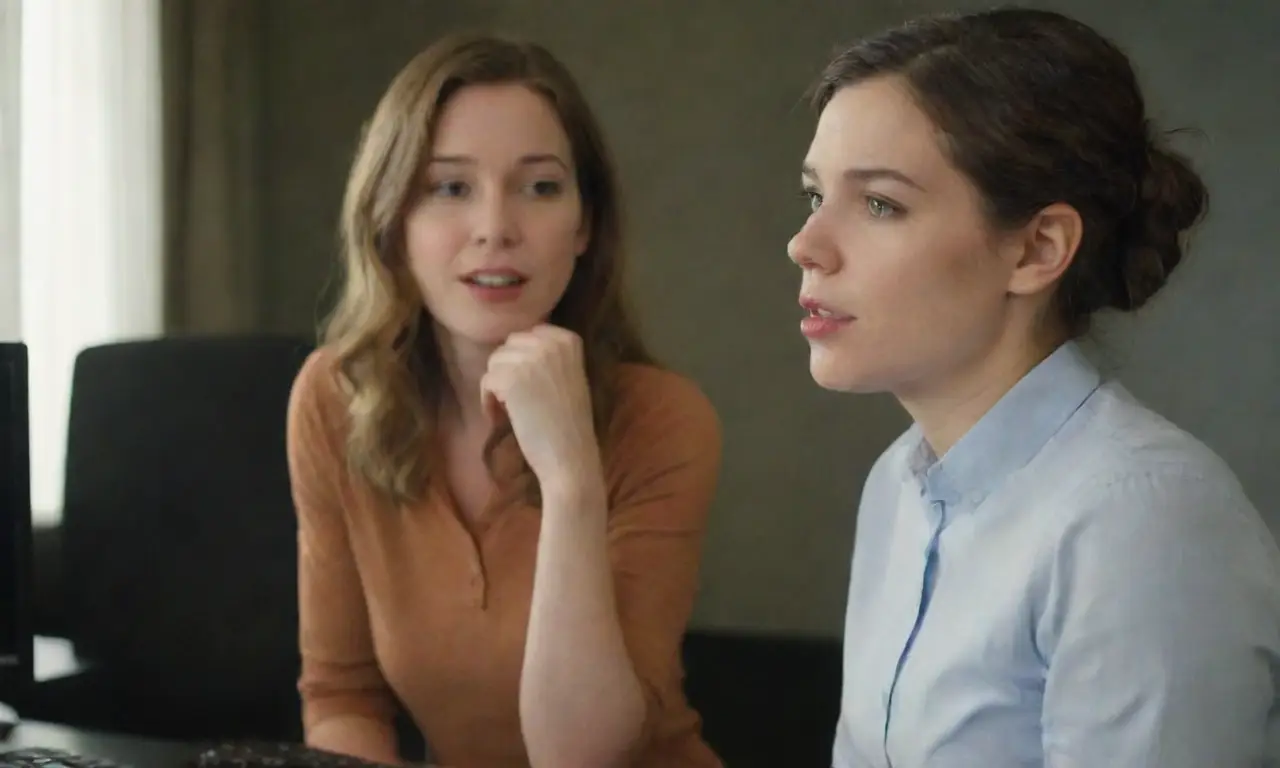
Low-Resource Language Synthesis
One of the most promising applications of transfer learning in speech synthesis lies in the domain of low-resource languages. Many languages around the world lack sufficient training data to build effective TTS systems. Transfer learning provides a robust framework to leverage resources from high-resource languages, enabling the model to learn phonetic and linguistic patterns that can be transferred to synthesizing speech in underrepresented languages. This not only democratizes access to technology but also preserves cultural identities by empowering local communities with the means to produce meaningful digital content in their native languages.
Speech Synthesis Techniques for Multilingual ApplicationsVoice Personalization and Emotion Modeling
Voice personalization is another fascinating area enhanced by transfer learning. For instance, if a TTS model is initially trained on a large dataset containing multiple speakers, it can use transfer learning to adapt to the unique vocal features of a specific individual. This process might involve tuning the model with just a few recordings of the target speaker, resulting in a voice that closely resembles that individual’s natural speech.
Moreover, transfer learning can enable emotion modeling in synthetic speech output. By training models on emotionally diverse datasets and using transfer learning techniques, researchers can enhance the synthesis of prosody and inflection tailored for specific emotional contexts. This capability could transform applications in industries like gaming, entertainment, and customer service, where personalized and contextually relevant responses are increasingly vital.
Enhancing Voice Quality with Pre-trained Models
Another significant avenue in which transfer learning proves beneficial is enhancing voice quality. Through pre-trained models that have established robust representations of linguistic features and natural speech production, researchers can fine-tune these models to improve aspects such as clarity, intonation, and expressiveness. The ongoing refinement of voice quality is crucial, especially in applications that require high levels of human-like interaction, such as virtual assistants, audiobooks, and educational tools.
Future Trends in Speech Synthesis and Transfer Learning
Rising Adoption of Multi-Lingual Models
As globalization continues to unify cultures and languages, there is an increasing demand for multilingual capabilities in speech synthesis systems. The future of TTS technology will likely see the development of multi-lingual models that leverage transfer learning extensively. These models can adopt techniques that allow them to switch seamlessly between languages while maintaining a high fidelity of synthesis quality. Furthermore, advancements in unsupervised and semi-supervised learning techniques will further enhance these models’ capabilities to learn from sparse datasets across diverse linguistic inputs.
Challenges and Solutions in Speech Synthesis Technology DevelopmentIntegration with Conversational AI
The synergy between transfer learning in speech synthesis and conversational AI represents another exciting frontier. As virtual assistants and chatbots continue to proliferate, enhancing their ability to communicate in human-like speech is paramount. By utilizing transfer learning, TTS systems can better adapt to the context and tone of conversations, producing responses that align more closely with user expectations. This dual enhancement of both understanding and output will create more engaging and productive interactions between users and technology.
Ethical Considerations and Accessibility
As we embrace the power of transfer learning in developing advanced speech synthesis technologies, it is vital to remain vigilant concerning the ethical considerations that arise. The potential for misuse, including deepfakes and misrepresentation, poses significant risks. Researchers and developers should prioritize creating responsible guidelines to ensure the ethical use of this technology. Additionally, equity in accessibility must be a primary consideration, as we strive to ensure that all communities, no matter their language or resources, have access to quality speech synthesis tools.
Conclusion
The convergence of transfer learning and speech synthesis is a remarkable testament to the capabilities and potential of modern AI technologies. Through innovative applications in low-resource language synthesis, voice personalization, and enhanced voice quality, transfer learning is changing the landscape of synthetic speech, making it more robust, versatile, and accessible than ever before. Moreover, as we peer into the future of this technology, the promise of widespread adoption, increased integration with conversational AI, and a focus on ethical considerations sets the stage for transformative developments.
As we continue our journey into this exciting realm, it is imperative for researchers, developers, and stakeholders to collaborate in harnessing the advantages of transfer learning ethically and inclusively. By doing so, we can ensure that the advancements in speech synthesis not only enhance our machines’ capabilities but also reflect the richness and diversity of human communication. In a world where sound and speech underpin so much of our interaction, the potential for empowering voices across the spectrum of languages is truly profound, heralding a future where everyone can be heard and understood in a manner that feels naturally theirs.
Ethical Considerations in Speech Synthesis and Voice CloningIf you want to read more articles similar to Harnessing Transfer Learning for Enhanced Speech Synthesis, you can visit the Speech Synthesis Applications category.
You Must Read