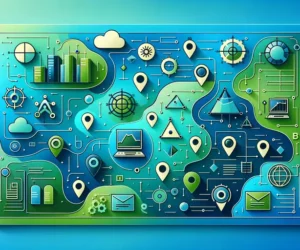
How Autoencoders are Revolutionizing Music Creation Processes
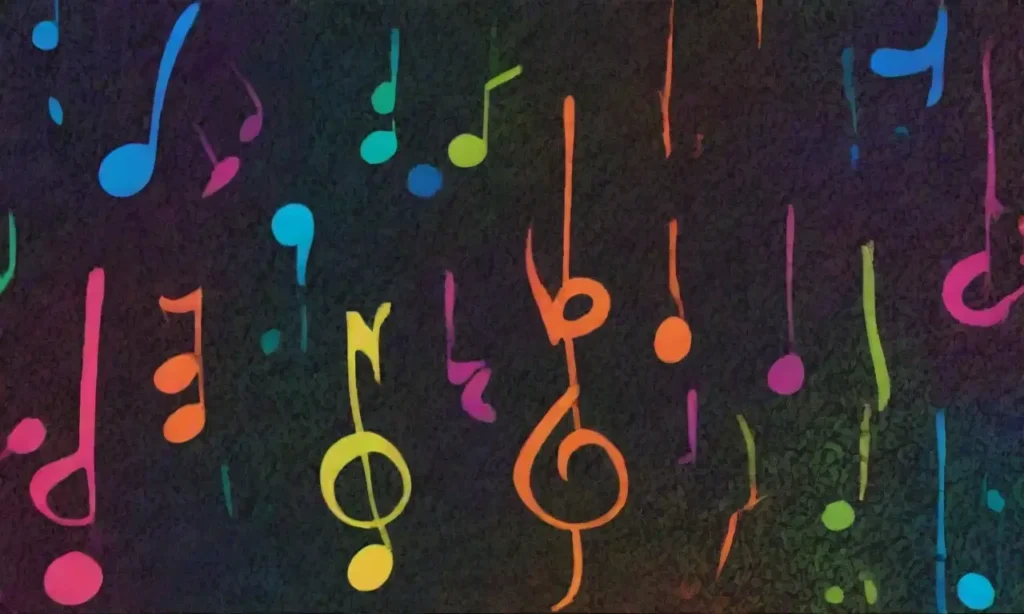
Introduction
In recent years, advancements in artificial intelligence (AI) have permeated nearly every facet of our lives, from autonomous vehicles to smart home devices. Among these innovations, autoencoders have emerged as a powerful tool for creative processes, particularly in the realm of music creation. Leveraging the principles of machine learning, autoencoders can analyze and replicate complex patterns found in musical compositions, giving rise to new avenues for musical exploration and innovation.
This article aims to explore the various ways autoencoders are transforming the music creation landscape. From their technical underpinnings to their practical applications, we'll delve into how this cutting-edge technology is not only assisting musicians but also reshaping the traditional music industry.
Understanding Autoencoders
Autoencoders are a specific type of neural network designed for unsupervised learning, primarily used for dimensionality reduction and feature extraction. They consist of two main components: the encoder and the decoder. The encoder compresses the input data (in this case, musical notes or audio signals) into a lower-dimensional representation, while the decoder reconstructs the original input data from this compressed form.
In musical applications, autoencoders can process audio files and learn their underlying features, such as rhythm, melody, and harmony. By effectively capturing the essence of the music, they can generate new compositions that exhibit similar characteristics. This capability not only streamlines the music creation process but also enables artists to explore diverse musical styles and combinations they might not have considered otherwise.
Building Your First AI Music Generator with Open Source LibrariesThe Architecture of Autoencoders
To fully grasp how autoencoders operate in the context of music, it is essential to understand their architecture. An autoencoder usually consists of an input layer, one or more hidden layers (which include the encoder and decoder), and an output layer. The hidden layers are crucial, as they capture the most informative aspects of the data while discarding noise and less relevant information.
The encoding step involves feeding the input data into the encoder, which maps it onto a reduced representation known as the latent space. This latent space is a compressed, abstract version of the input data and contains the key features that define it. Music, for example, can be represented in various forms, including Mel spectrograms or musical notation, allowing the autoencoder to learn the correlations and patterns inherent in the music.
The decoding step then takes the information from the latent space and attempts to reconstruct the original input, which helps in assessing how well the model has learned the data. Effective training of the autoencoder requires minimizing the loss function, which measures the difference between the original data and the reconstructed output. Over time, the autoencoder becomes proficient in capturing and recreating the intricacies of different musical pieces.
Applications of Autoencoders in Music Creation
The versatility and effectiveness of autoencoders in music creation have led to numerous exciting applications. One notable area is music generation, where autoencoders can create entirely new compositions by sampling from the latent space. Artists can leverage these generated pieces as inspiration, leading to innovative musical ideas and compositions that blend various genres and styles.
Using Constraint Satisfaction in Algorithms for Music GenerationMoreover, autoencoders can aid in music transformation or style transfer, where a piece of music is transformed into a different style while retaining its core elements. For instance, an autoencoder could take a jazz composition and reimagine it in a classical style, showcasing the strengths of both genres and resulting in a hybrid piece that could attract diverse audiences.
Another captivating application is audio restoration, wherein autoencoders can process degraded recordings, such as older vinyl records, and enhance their quality. By learning the characteristics of high-quality audio, autoencoders can effectively strip away noise and artifacts, breathe new life into old recordings, and make them more enjoyable for modern listeners. This convergence of technology and artistry illustrates how AI can preserve musical history while offering fresh experiences for contemporary audiences.
The Impact of Autoencoders on the Music Industry
The integration of autoencoders into the music industry is not just a technological curiosity; it has far-reaching implications for artists, producers, and listeners alike. As musicians increasingly embrace machine learning tools, the role of the artist is evolving. Rather than merely being creators, artists can now position themselves as curators or collaborators with AI, enhancing their creative processes while maintaining their unique voices.
One significant impact of this technology is on music democratization. With the advent of tools powered by autoencoders and other AI algorithms, aspiring musicians now have access to professional-grade music production capabilities that were once confined to studios and experienced producers. This accessibility empowers individuals to experiment and create music even without formal training or substantial financial backing, democratizing the music landscape.
Interactive Music Generation: Algorithms that Learn from User InputAnother fascinating consequence is the potential for personalization in music consumption. As autoencoders can analyze individual preferences and tastes, they can inform music recommendation systems that curate playlists tailored to listeners' unique auditory profiles. This can lead to a more enriching experience, allowing fans to discover lesser-known artists and genres that resonate with them, thereby expanding the market for diverse musical expressions.
The Role of Collaboration between Artists and AI
The interplay between artists and autoencoders transcends traditional boundaries, paving the way for unprecedented collaborations. Musicians can treat autoencoders not only as tools but also as collaborators in the creative process. By experimenting with autoencoder-generated outputs, artists can explore new creative directions they might not have taken otherwise, thereby fostering innovation in their work.
Additionally, some artists have begun to integrate autoencoders into live performances, where real-time audio processing allows for sonic experimentation. For instance, an artist might create interactive installations that adjust to audience reactions or blend different musical styles on the fly, showcasing the potential of machine learning in a dynamic and engaging context.
Collaborations between autoencoders and musicians highlight an evolving sector where creativity and technology coexist. Artists can now use AI-generated elements in their compositions while retaining their human touch, creating a unique juxtaposition that elevates the art form.
Exploring Variational Autoencoders in Music Composition WorkflowsConclusion
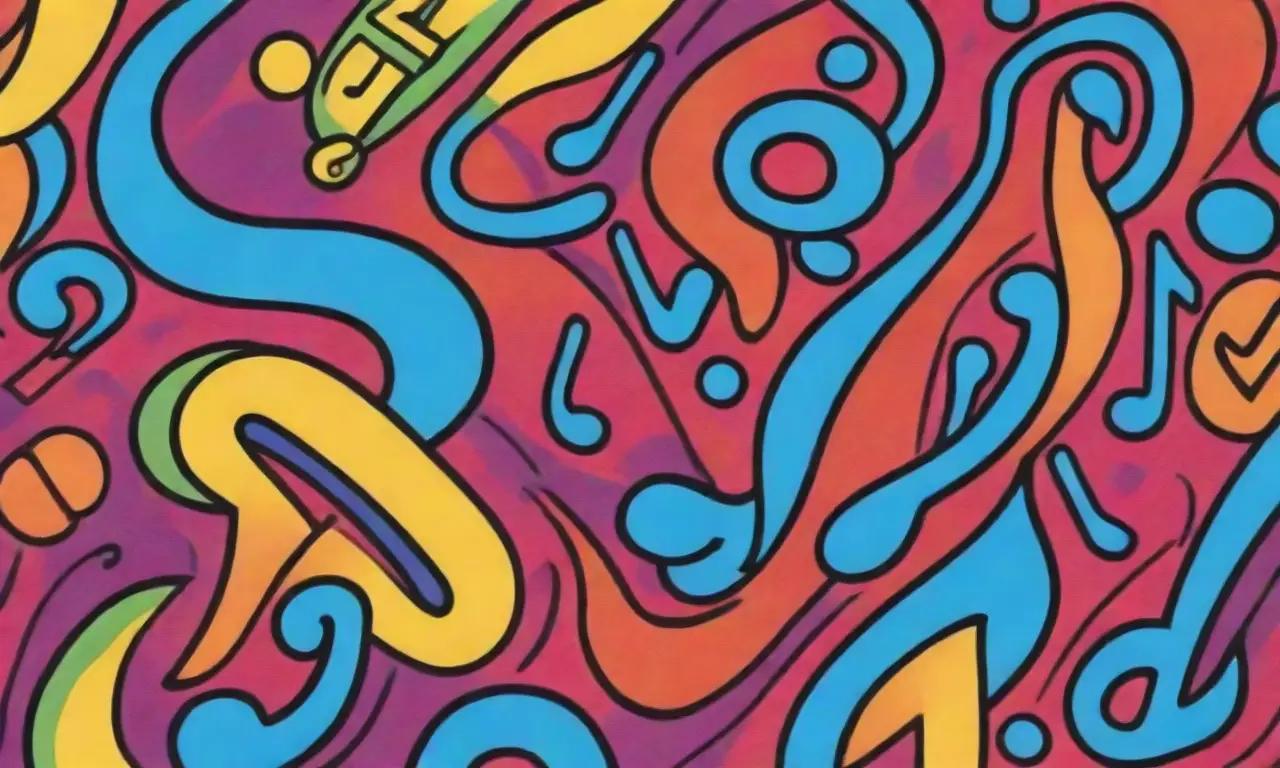
The advent of autoencoders in the music creation process represents a remarkable chapter in the intersection of technology and artistry. By learning and reproducing the intricacies of music, these neural networks have redefined the role of both musicians and listeners, making music production more accessible and collaborative than ever.
Through applications such as music generation, transformation, and restoration, autoencoders are not merely reshaping how music is created but also how it is consumed and appreciated. As these technologies continue to evolve, we can anticipate further innovations that will push the boundaries of musical expression and creativity.
However, the harmonious collaboration between artists and autoencoders also invites ongoing discussions about the nature of creativity and the role of human intuition in artistic processes. Balanced carefully, this relationship holds the potential to forge a new paradigm where both human creativity and artificial intelligence coalesce to inspire extraordinary musical experiences.
Collaborative AI: Working with Machines to Generate New MusicAs we look towards the future, it’s clear that the impact of autoencoders on music creation will only grow, inviting a new era of exploration for artists and listeners alike. The revolution in music creation processes brought about by autoencoders is just the beginning of an exciting journey, one that promises to continuously innovate the soundscape for generations to come.
If you want to read more articles similar to How Autoencoders are Revolutionizing Music Creation Processes, you can visit the Music Generation category.
You Must Read