
Implementing Machine Learning in Incident Response Strategies Today
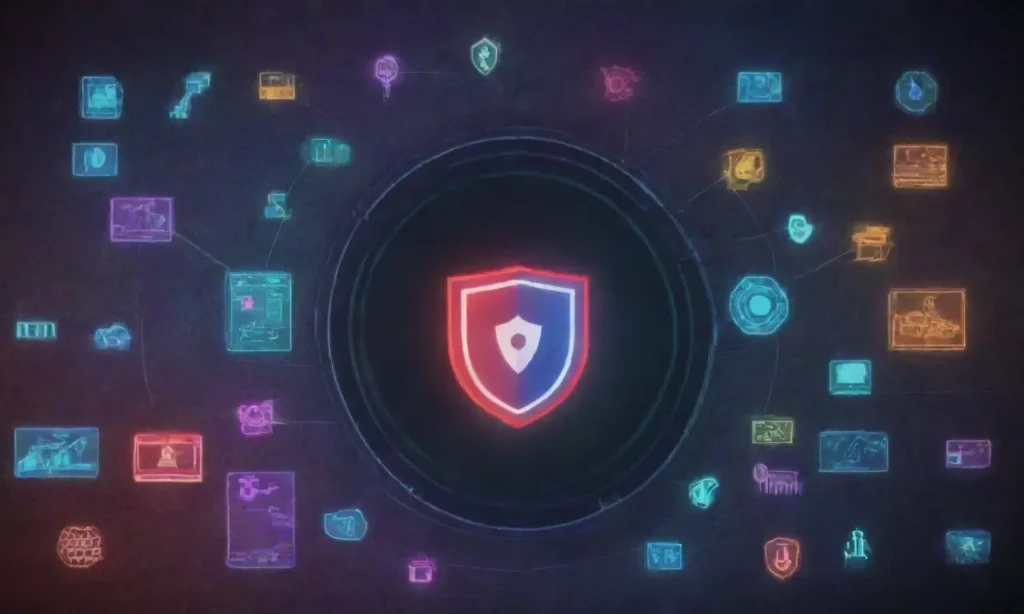
Introduction
In the modern era of cybersecurity, organizations face an unprecedented number of threats with growing sophistication. Every day, millions of attacks are launched worldwide, targeting vulnerabilities in systems, applications, and networks. While traditional incident response practices have played a crucial role in mitigating risks, the inclusion of Machine Learning (ML) stands to radically enhance these capabilities. The integration of ML into incident response strategies not only elevates the speed and accuracy of threat detection but also enables organizations to respond effectively to complex and evolving attacks.
This article aims to provide a deep dive into the significance of implementing ML in incident response strategies. We will explore how machine learning can transform the landscape of cybersecurity incident management, discuss the various techniques applicable in today's environments, and provide practical steps for organizations looking to incorporate ML into their existing frameworks. Along the way, we will highlight best practices, potential challenges, and future trends shaping the incident response field.
Understanding Machine Learning in Cybersecurity
Machine learning is a subset of artificial intelligence (AI) that enables systems to learn from data, improve their performance over time, and make decisions without human intervention. Within the context of cybersecurity, ML models can analyze extensive datasets to identify patterns, predict future occurrences, and automate response actions.
Machine learning algorithms can be categorized into several types, including supervised, unsupervised, and reinforcement learning. Supervised learning involves training models on labeled datasets where the outcome is known, thus increasing accuracy in identifying known threats. Conversely, unsupervised learning deals with unlabeled data, enabling the identification of hidden patterns or anomalies without predefined outcomes, making it particularly beneficial for detecting zero-day attacks or new malware types that have not yet been categorized.
Guidelines for Developing Machine Learning Models in CybersecurityFurthermore, reinforcement learning uses trial and error to teach algorithms the best action to take in different situations. This method has a significant role in adaptive incident response, where systems learn to improve their actions based on prior successes and failures.
The Role of Machine Learning in Incident Response
The role of ML in incident response goes beyond merely identifying threats; it includes prioritizing incidents, streamlining remediation processes, and offering predictive capabilities that assist teams in anticipating potential attacks before they manifest.
Threat Detection and Analysis
One of the most notable applications of machine learning in incident response is its ability to enhance threat detection and analysis. Traditional systems rely on predefined rules and signatures to detect threats, leaving gaps regarding new or evolving attack patterns. ML algorithms, however, can process vast amounts of network data in real-time, learning to differentiate between normal and anomalous behavior. For instance, an ML model can develop an understanding of typical user behavior on a corporate network. When an employee suddenly downloads large volumes of sensitive data or accesses restricted sections of the network, the system can trigger alerts for further investigation.
Another critical task involves identifying potential indicators of compromise (IoCs). ML has the capacity to analyze logs, traffic, and files to surface IoCs that human analysts may overlook due to the sheer volume of data. By automating the analysis of extensive datasets, organizations can gain insights far more quickly and accurately than before.
How to Choose the Right Machine Learning Algorithm for Cyber NeedsIncident Prioritization and Risk Assessment
Upon detecting a potential incident, the next step involves assessing its severity and potential impact. Machine learning can significantly streamline this process by evaluating the context and historical data related to similar incidents. For instance, some ML models can estimate the likelihood of data exfiltration based on attack vectors, the profiles of assets under siege, and prior incident histories.
Moreover, prioritization is crucial in incident response as organizations often deal with many incidents simultaneously. By employing ML algorithms trained on historical incidents, response teams can automate the prioritization process based on risk levels, potential damage, and required response times. This approach ensures that the most pressing threats receive immediate attention, allowing organizations to allocate their limited resources effectively.
Automation of Incident Response
ML integration extends to automating certain aspects of the incident response lifecycle. Once an incident has been detected and prioritized, various automated responses can be executed. This may involve isolating affected systems, blocking suspicious IP addresses, or enforcing security policies without waiting for manual intervention.
For instance, Security Orchestration, Automation, and Response (SOAR) platforms can integrate ML to implement automated workflows, significantly reducing the response times associated with incident containment. These automated responses not only minimize the impact of attacks but also free up human security analysts to focus on more complex or high-level tasks that require critical thinking and deeper investigation.
Understanding the Role of AI in Modern Cybersecurity PracticesChallenges and Best Practices
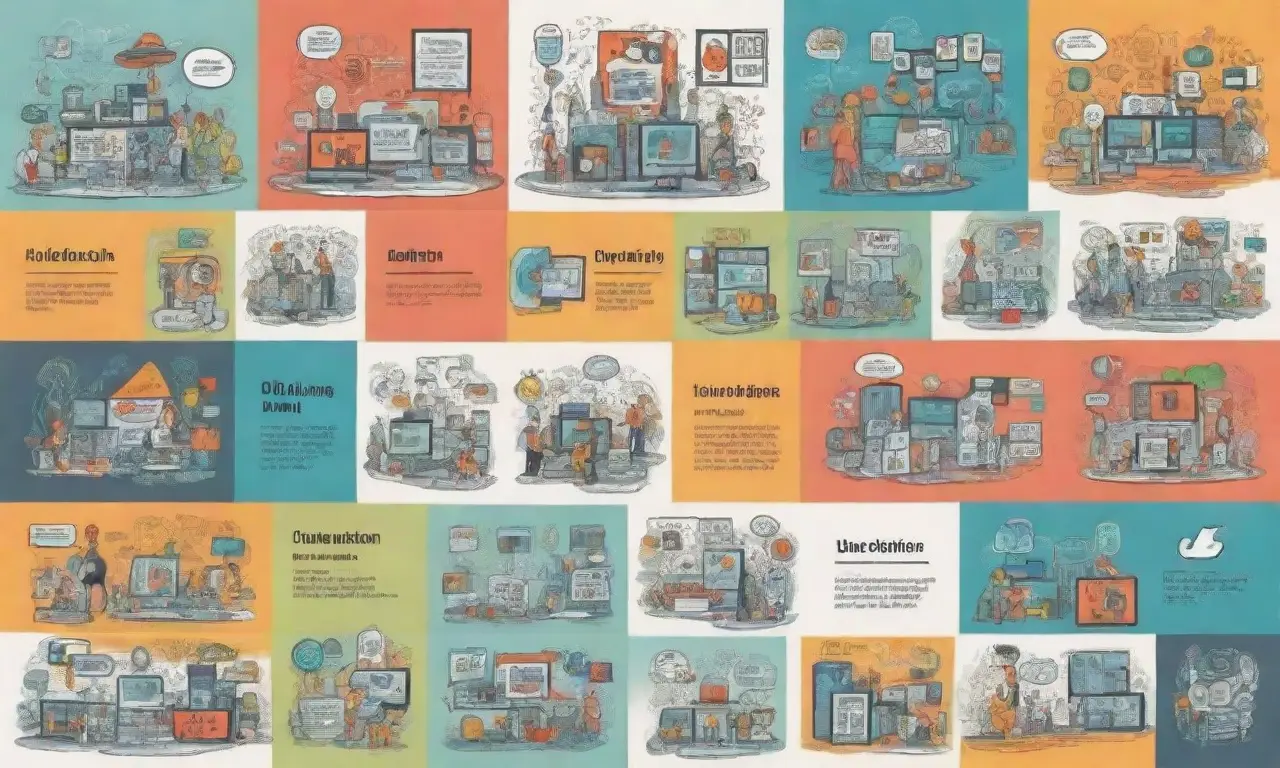
While the benefits of implementing machine learning in incident response are profound, there are challenges organizations must navigate to achieve successful integration.
Data Quality and Availability
Machine learning models rely heavily on high-quality data to function effectively. If the data used to train models is incomplete, unstructured, or biased, it can significantly diminish the accuracy of predictions. Therefore, organizations must invest in data collection and preparation processes that ensure the integrity and reliability of the datasets fed into ML models.
Another component of data quality is the challenge of availability. Organizations need comprehensive access to data across various systems, platforms, and logs to create holistic models. To combat this, enterprises should establish robust data governance policies that ensure an organized approach to data management.
Unsupervised Learning Approaches to Identify Cybersecurity ThreatsTalent Gap and Expertise
Integrating machine learning into incident response demands a certain level of expertise that many organizations may not have readily available. There is often a significant gap between traditional cybersecurity practices and the skill sets required to develop and implement machine learning models. Seeking professionals with a unique blend of cybersecurity and data science qualifications is essential, yet often challenging.
To address this issue, organizations should focus on continuous training and upskilling of their existing cybersecurity personnel in data science and machine learning. Collaborating with external experts or consulting firms can also supplement internal capabilities.
Ethical and Privacy Considerations
The utilization of machine learning in incident response raises potential ethical and privacy considerations. The automation of processes carries intrinsic risks, where the reliance on algorithms may inadvertently overlook critical nuances of human judgment. It is crucial that organizations maintain a balanced approach by incorporating human oversight in decision-making processes.
Moreover, organizations must remain vigilant regarding data privacy and compliance regulations, ensuring that their data collection and processing practices abide by relevant laws and ethical standards. This is especially pertinent in light of regulations such as the General Data Protection Regulation (GDPR) and the California Consumer Privacy Act (CCPA).
Effective Machine Learning Models for Threat Detection in CybersecurityConclusion
Implementing machine learning in incident response strategies is not just a trend; it is a response to escalating cyber threats and the need for proactive defense mechanisms. By learning from vast amounts of data, automating critical functions, and improving detection accuracy, ML drastically reshapes how organizations manage cybersecurity incidents. Enhanced threat detection, risk assessment, and automated responses all contribute to more resilient security infrastructures.
However, organizations must be diligent in addressing data quality issues, bridging the talent gap, and navigating ethical considerations that arise with ML integration. This will require investment in both technologies and training to create a culture of continuous learning and adaptation. As cyber threats continue to evolve, organizations that adopt machine learning as a core component of their incident response frameworks will not only be better positioned to mitigate current threats but also to anticipate future attacks.
In conclusion, for organizations aiming to stay ahead of the curve in cybersecurity, integrating machine learning into incident response is crucial. It represents a transformative shift in the approach to threat management, paving the way for faster, more effective incident responses and ultimately leading to a more secure digital environment. By embracing this innovative technology, organizations can safeguard their assets and maintain the trust of their customers and stakeholders in an increasingly complex threat landscape.
If you want to read more articles similar to Implementing Machine Learning in Incident Response Strategies Today, you can visit the Cybersecurity category.
You Must Read