
Improving Overstock and Understock Situations Using AI Methods
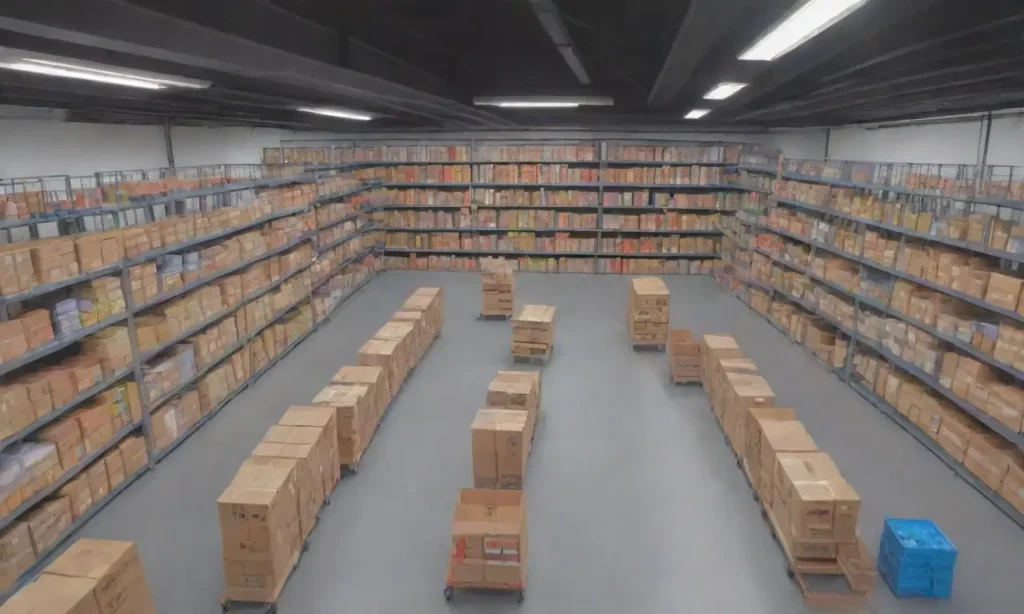
Introduction
The retail environment has undergone a substantial transformation in recent years, driven by advancements in technology and the rising expectations of consumers. Among the myriad of challenges that retailers face, inventory management remains a critical concern. Maintaining the perfect balance of stock is vital, as both excess inventory (overstock) and insufficient stock (understock) can significantly impact a business's profitability and customer satisfaction. Overstock can lead to increased holding costs and potential markdowns, while understock can result in lost sales and disappointed customers.
This article delves into the various challenges retailers face regarding overstock and understock situations and explores how Artificial Intelligence (AI) methods can effectively improve these issues. We will highlight the types of AI techniques, such as predictive analytics, demand forecasting, and machine learning, that can lead to a more streamlined inventory management process, enabling businesses to thrive in an increasingly competitive marketplace.
The Importance of Effective Inventory Management
Effective inventory management is critical for businesses, particularly in the retail sector. It is not merely about keeping track of products; it encompasses understanding market dynamics, consumer preferences, and optimizing purchasing decisions. Poor inventory management can lead to substantial financial losses, decreased customer satisfaction, and ultimately, tarnished company reputation.
Overstock situations typically materialize due to misjudgments in demand forecasting or inefficiencies in the supply chain. Retailers find themselves with surplus stock that incurs storage costs and may need to be discounted to clear the shelves. On the opposite end, understock creates a situation where demand outstrips supply, causing missed sales opportunities and potentially loosing loyal customers as they turn to competing stores to meet their needs. Hence, leveraging AI for inventory management is no longer a luxury but a necessity for modern retailers.
Utilizing Neural Networks for Accurate Inventory ForecastingA key aspect of effective inventory management lies in understanding consumer behavior and trends. Consumers are increasingly influenced by various factors, such as seasonality, promotions, and market trends. Analyzing this data using traditional methods can be labor-intensive and prone to inaccuracies. Hence, AI stands out as a game-changer, as it can process massive amounts of data in real time, yielding more accurate insights into consumer behaviors and preferences.
AI Methods for Improving Overstock Situations
Predictive Analytics
Predictive analytics is one of the foremost AI methodologies revolutionizing inventory management. Utilizing historical data, predictive analytics identifies patterns and trends that help forecast future demand. For instance, retailers can analyze past sales data and external factors, such as economic changes and holiday seasons, to predict how much of a product they will need at any given time.
By integrating predictive analytics into their inventory systems, retailers can effectively minimize overstock situations. For example, if sales of specific items typically surge during the holiday season, predictive analytics could suggest increasing stock levels well ahead of time. Conversely, if certain products are consistently underperforming, retailers could adjust their buying decisions to avoid excess stock, thus minimizing potential losses associated with overstock.
Additionally, vital insights gained from predictive analytics can improve supplier communication. By sharing accurate forecasts with suppliers, retailers can better align their inventory levels with market demand, ensuring that products arrive just in time to meet customer needs without saturating the market.
Optimizing Warehouse Operations with ML-Based Inventory SolutionsMachine Learning Algorithms
Another potent AI method for tackling overstock issues is the application of machine learning algorithms. These algorithms can delve deep into datasets, identify complexities that might not be apparent to human analysts, and continually refine their predictions as new data comes in. Machine learning works through a process where the algorithm learns from data and improves its forecasting ability autonomously without being explicitly programmed for every variable.
For instance, using machine learning algorithms, retailers can gather real-time data about customer purchasing patterns and seasonal fluctuations. By analyzing this data, the system can predict which products are likely to become overstocked and prompt the retailer to modify their supply quantities proactively. The real benefit of machine learning lies in its adaptability: as market conditions change, the algorithms adjust their parameters and deliver insights that are relevant to the current context.
Furthermore, machine learning can also integrate consumer sentiment analysis. Analyzing social media trends or customer reviews can provide insights into public perceptions of specific products or brands, thus informing inventory decisions to mitigate overstock risks. Retailers can pivot strategies based on sentiment analysis, managing inventory levels more responsively.
AI Methods for Improving Understock Situations

Demand Forecasting
Demand forecasting is another vital AI approach focusing on reducing understock situations. By synthesizing vast and diverse data from multiple sources, AI can help retailers make informed purchasing decisions. The integration of real-time data—such as sales history, market trends, and external factors—can provide an accurate demand forecast, allowing retailers to maintain optimal stock levels.
Advanced demand forecasting models leverage historical sales data alongside external variables such as weather, events, and competitor activities to predict future demand accurately. With this insight, retailers can better prepare for high-demand periods, ensuring that they have sufficient stock on hand. For example, an ice cream store can analyze weather forecasts to determine when warmer temperatures are predicted and prepare by ordering additional stock of popular flavors.
Moreover, AI-powered demand forecasting offers the possibility of scenario planning where retailers can simulate different situations and predict outcomes based on those scenarios. This allows for more dynamic decision-making in inventory management, as retailers can foresee potential risks—like sudden demand spikes due to a trending social media post and proactively act to mitigate understock situations.
Real-Time Inventory Tracking
Real-time inventory tracking is crucial for preventing understock situations. With the advent of AI, retailers can employ smart technologies such as IoT sensors and RFID tags that continuously monitor inventory levels. These systems provide immediate alerts and analytics data regarding stock levels throughout the supply chain, thus enabling real-time decision-making.
By implementing real-time inventory tracking, retailers gain precise oversight over their stock levels, allowing them to respond quickly to fluctuating demand patterns. For example, if a popular product begins to sell out rapidly, an alert can trigger the automated reorder systems, ensuring that stock is replenished promptly. This capability integrates seamlessly with demand forecasting predictions, creating a more responsive inventory management system that can capture market dynamics effectively.
Additionally, real-time inventory tracking supports data-driven decision-making, providing retailers with crucial metrics for their product performance. Retailers can analyze sell-through rates, identify slow-moving items, and make informed decisions about replenishing fast-selling products, further enhancing their ability to avoid understock situations.
Conclusion
In the ever-evolving retail landscape, the ability to strike a balance between overstock and understock is of paramount importance. Artificial Intelligence has emerged as a revolutionary force in the realm of inventory management, providing robust methodologies that enable retailers to make informed, data-driven decisions.
Through the application of predictive analytics, machine learning, demand forecasting, and real-time inventory tracking, retailers can proactively manage their stock levels and enhance customer satisfaction. The benefits of implementing these AI methods are numerous; not only do they reduce operational costs tied to overstock, but they also ensure that customers can find the products they want when they want them.
As consumer expectations continue to grow and competition intensifies, embracing AI in inventory management is not merely advantageous but essential for survival. Retailers willing to invest in AI-driven strategies will be well-equipped to navigate the complexities of the modern marketplace, making smarter inventory decisions that benefit their operations and elevate the shopping experience for consumers. As we continue to witness technological advancements, the future of inventory management will undoubtedly be shaped by the innovative capabilities of AI.
If you want to read more articles similar to Improving Overstock and Understock Situations Using AI Methods, you can visit the Inventory Management category.
You Must Read