
Integrating Machine Learning Algorithms into HR Hiring Processes
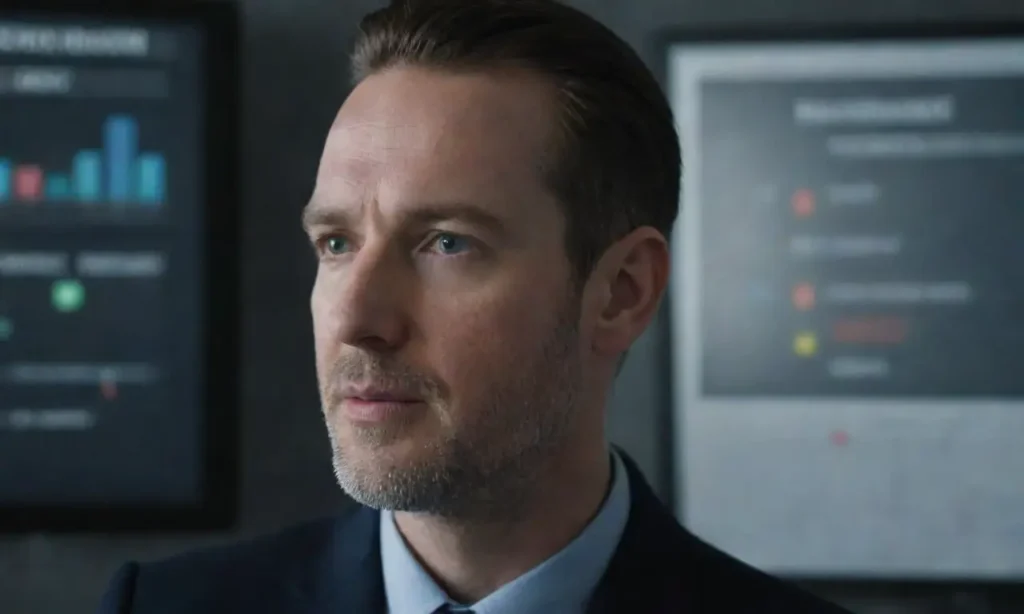
Introduction
In the rapidly evolving landscape of modern recruitment, Machine Learning (ML) has emerged as a powerful tool aimed at optimizing hiring processes. This infusion of technology has the potential to not only streamline administrative tasks but also enhance the precision of decision-making in candidate selection. With the massive amounts of data generated every day, traditional manual approaches to hiring can be inefficient and biased. Integrating machine learning algorithms into the hiring process can help organizations sift through vast candidate pools, identify the most suitable candidates, and foster a diverse workforce.
This article will dive deep into the specifics of how machine learning can be integrated into the hiring processes of Human Resources (HR) departments. We will explore the types of algorithms used, the stages of the hiring process that can be augmented with ML, the potential benefits and challenges, and best practices for successful implementation. By the end of this discussion, HR professionals will have an in-depth understanding of how to leverage these advanced technologies effectively.
Understanding Machine Learning and Its Relevance in HR
Machine Learning is a subset of artificial intelligence (AI) that enables systems to learn from data, improve their performance over time, and make predictions without being explicitly programmed to do so. In HR, this means using algorithms to analyze employee data, identify patterns, and predict outcomes concerning hiring, employee performance, and retention. The primary goal of employing machine learning in HR is to eliminate biases, streamline workflows, and improve the overall candidate experience.
Types of Machine Learning Algorithms
There are three primary types of machine learning algorithms that are particularly relevant to HR processes: supervised learning, unsupervised learning, and reinforcement learning.
Exploring Case Studies of Successful Machine Learning in HRSupervised Learning: This approach relies on labeled data sets to train algorithms. In the hiring context, supervised learning can be applied to analyze previous hiring outcomes to predict the likelihood of success for future candidates. For instance, resumes of previously successful employees can be used to create a model that scores new applicants based on similarities.
Unsupervised Learning: Unlike supervised learning, this method works with unlabeled data to identify hidden patterns or groupings. In hiring, it could help in segmenting candidates based on various attributes such as skills, experience, and cultural fit, leading to more nuanced candidate assessments.
Reinforcement Learning: This is a type of machine learning where algorithms learn behavior based on responses to actions taken in an environment. In HR, reinforcement learning can optimize recruitment strategies by evaluating candidate engagement and performance based on how individuals respond to different hiring practices.
The Role of Data in Machine Learning Initiatives
The success of machine learning initiatives in HR is predominantly reliant on data quality and availability. Organizations often have access to a wealth of data that can be harnessed effectively to train algorithms, including resumes, application forms, employee performance reviews, and more. However, the data must be comprehensive and devoid of biases to ensure fair outcomes. This is particularly critical in the hiring process, as biased algorithms can perpetuate discrimination against certain demographics.
Streamlining HR Processes with Predictive Machine Learning ModelsWhen considering data sources, it is essential to implement practices for data cleaning and preprocessing to remove inaccuracies or irrelevant information. Additionally, organizations should ensure compliance with legal frameworks such as the General Data Protection Regulation (GDPR) and other data protection laws, which may dictate how personal data can be used and stored.
Stages of the Hiring Process Enhanced by Machine Learning
Integrating machine learning into the hiring process can be beneficial at various stages. These include sourcing candidates, screening applicants, interviewing, and onboarding. Let's explore each of these stages in detail.
Sourcing Candidates
The initial stage of the hiring process involves identifying suitable candidates. Machine learning algorithms can analyze data from various sources including social media, job boards, and company databases to find potential matches efficiently. For example, Natural Language Processing (NLP), a branch of machine learning, can be used to parse job descriptions and resumes, linking the right skill sets with job opportunities.
Moreover, predictive analytics can help HR professionals identify emerging talent or anticipate the need for specific roles based on workforce trends and requirements. By employing such algorithms, HR teams can move beyond traditional sourcing methods, which often rely heavily on job postings, and instead proactively engage with passive candidates who may not actively apply but possess desirable skills.
The Role of AI in Enhancing Employee Performance EvaluationsScreening Applicants
The screening process is critical in determining which candidates eventually make it to the interview stage. With dozens or even hundreds of applications to sift through, machine learning algorithms can significantly reduce workload and enhance efficiency. Using trained models, machine learning can automatically score resumes against predefined hiring criteria, such as experience, qualifications, and skills.
In this context, skill assessments can also be automated through algorithm-driven evaluations. For instance, some organizations deploy online assessments that automatically score candidate responses. By filtering candidates based on their scores and cultural fit metrics, HR can ensure that only the most relevant applicants are selected for interviews, thus optimizing time and resources.
Interviewing and Assessment
The interview process can also be greatly improved using machine learning technologies. Conducting structured interviews with the help of algorithm-driven frameworks not only standardizes the process but also reduces biases often associated with human judgment. Analyzing candidates' responses can provide insights into their behavioral traits and competencies, ensuring a more objective assessment.
Furthermore, video interview platforms that incorporate machine learning algorithms can analyze verbal and non-verbal cues during interviews. Such platforms can gauge the candidate's confidence, enthusiasm, and communication skills, offering valuable feedback that can complement traditional interview methods.
Benefits and Challenges of ML Integration in HR

Integrating machine learning into HR hiring processes offers several distinct benefits, yet it is not without its challenges.
Benefits of ML Integration
Enhanced Efficiency: By automating various hiring tasks, machine learning reduces the time and effort HR professionals need to spend on repetitive tasks, speeding up the overall recruiting process significantly.
Data-Driven Decision-Making: ML algorithms provide data-backed insights, allowing HR professionals to make more informed decisions regarding candidate selection and reducing subjective biases.
Improved Candidate Experience: The use of machine learning in the hiring process can streamline communication with candidates, resulting in faster response times and a more engaging experience, which can improve an organization's employer brand.
Challenges of ML Integration
Data Bias: If the training data used to develop machine learning algorithms is biased or unrepresentative of diversity in the workplace, it can lead to biased hiring decisions, perpetuating inequality.
Implementation Costs: Organizations may face hurdles in terms of integration costs, software acquisition, and the need to train HR staff to utilize these advanced systems effectively.
Change Management: Transitioning to a technology-driven hiring process may face resistance from traditional HR professionals who are more accustomed to conventional hiring practices. Educational initiatives and change management strategies will be crucial to mitigate this resistance.
Conclusion
As organizations continually strive to enhance their recruitment processes, integrating machine learning into HR hiring processes represents a promising solution that can lead to improved efficiency, fairness, and effectiveness. However, it is essential to recognize that successful ML integration is a journey that requires a thoughtful approach to data management, algorithm selection, and personnel training.
By embracing the potential of machine learning while being cognizant of its challenges, HR departments can forge a more inclusive and data-driven approach to hiring. This not only benefits the organization in terms of improved talent acquisition but also contributes to a diverse and skilled workforce poised to tackle the dynamic challenges of the modern business landscape. As technology continues to advance, the synergy between HR practices and machine learning will undoubtedly play a pivotal role in shaping the future of work and talent management. With the right strategies in place, organizations can leverage these innovative technologies to create a fairer, more efficient, and strategic hiring process.
If you want to read more articles similar to Integrating Machine Learning Algorithms into HR Hiring Processes, you can visit the Human Resource Management Systems category.
You Must Read