
Integrating Machine Learning Tools with Existing Support Systems
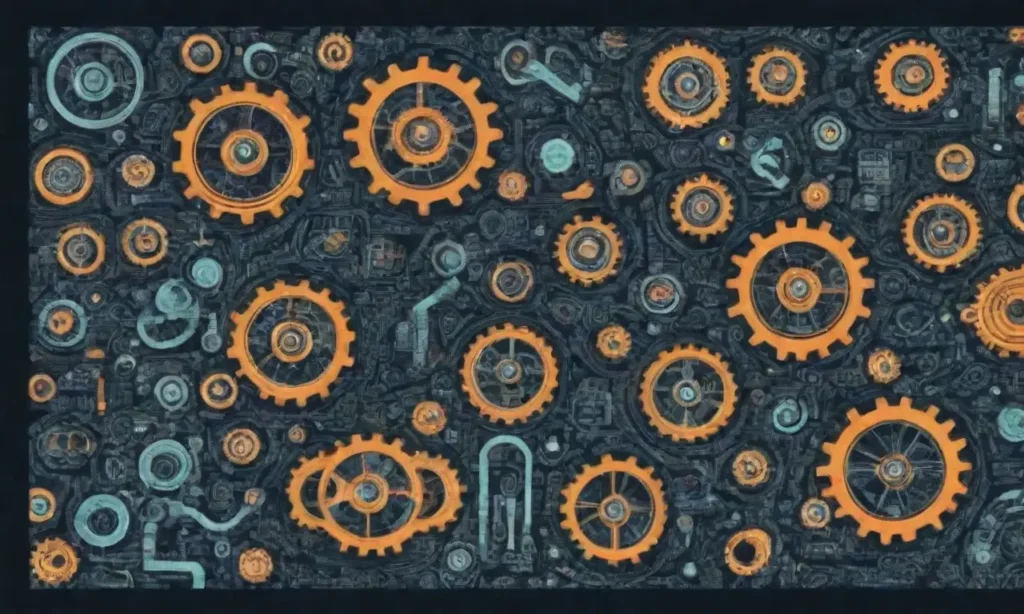
Introduction
In recent years, the evolution of machine learning (ML) has transformed various fields, significantly altering how tasks are performed and services are offered. One particular area where these advancements have made a noteworthy impact is in customer support systems. By leveraging machine learning algorithms, organizations can streamline their operations, enhance user experiences, and ultimately provide superior services to their customers. However, integrating these advanced tools into existing support frameworks is a complex process that requires a sound understanding of both the technology and the current support infrastructure.
This article endeavors to explore the multifaceted nature of integrating machine learning tools with existing support systems. We will delve into the background of machine learning in customer support, the key benefits of integration, the challenges businesses might face, and the best practices for a successful deployment. For organizations looking to modernize their support operations, this comprehensive guide offers actionable insights and detailed steps to navigate the integration process effectively.
Understanding Machine Learning in Support Systems
Machine learning refers to a subset of artificial intelligence that enables systems to learn from data and improve their performance over time without being explicitly programmed. In customer support, machine learning can be employed to automate processes, analyze customer data, enhance decision-making, and deliver personalized experiences.
A primary application of machine learning in support systems is natural language processing (NLP), which allows systems to understand and interpret human language. This capability powers chatbots that can engage customers in real-time or assist support agents by parsing through vast amounts of queries to identify trends and issues. Another application is predictive analytics, where machine learning algorithms analyze historical customer interactions to forecast future needs, enabling teams to proactively address potential concerns before they escalate.
Building Better Customer Relationships Through ML InsightsMoreover, machine learning can play a critical role in sentiment analysis. By evaluating customer communications, support teams can gauge customer satisfaction levels and identify potential areas for improvement. Such insights can lead to more informed strategies in enhancing customer loyalty and trust—two essential components in building lasting relationships with clients.
Benefits of Integrating Machine Learning Tools
Enhanced Efficiency and Automation
One cannot overlook the immense efficiency gains achieved through the automation of repetitive tasks. With the incorporation of machine learning, organizations can take advantage of intelligent routing systems that direct customer queries to the appropriate support personnel based on the complexity and content of the request. This not only expedites response times but also reduces the workload on human agents, allowing them to focus on more intricate and meaningful tasks.
Additionally, automation can extend to ticketing systems, where machine learning algorithms analyze previous resolutions and suggest potential answers for common questions. This facilitates a faster resolution cycle, leading to improved customer satisfaction. As ticketing systems evolve, incorporating ML tools can refine the decision-making process, ensuring that relevant data and solutions are always at the registration of a support agent.
Personalized Customer Experiences
With the power of machine learning, support systems become capable of delivering highly personalized experiences that can significantly enhance customer satisfaction rates. By analyzing a wealth of customer data—from browsing behavior to purchase history—organisations can tailor their support interactions to meet the unique needs of each customer.
Exploring the Intersection of ML and Human Touch in SupportFor instance, proactive outreach may be facilitated, where support teams can anticipate customer needs based on their interactions with the brand. If the algorithm detects that a customer often has inquiries about a specific product, it can trigger an automated suggestion or allow agents to prioritize that customer’s follow-up. This level of personalization not only fosters a more rewarding experience for customers but strengthens their loyalty to the brand.
Data-Driven Decision-Making
The integration of machine learning tools leads to more informed and data-driven decision-making processes in customer support. With access to advanced analytics, organizations can analyze customer data more thoroughly, identifying trends, common issues, and service bottlenecks. Such insights can be invaluable when making strategic decisions related to resource allocation, training needs for support staff, and the development of new products or services.
Moreover, organizations can continually enhance their machine learning models through feedback loops. By collecting data on how well the system resolves customer queries and integrating that data into future algorithm iterations, companies can cultivate a culture of continuous improvement. This iterative approach to system adjustments vastly increases operational efficiency and improves overall service excellence.
Challenges in Integration
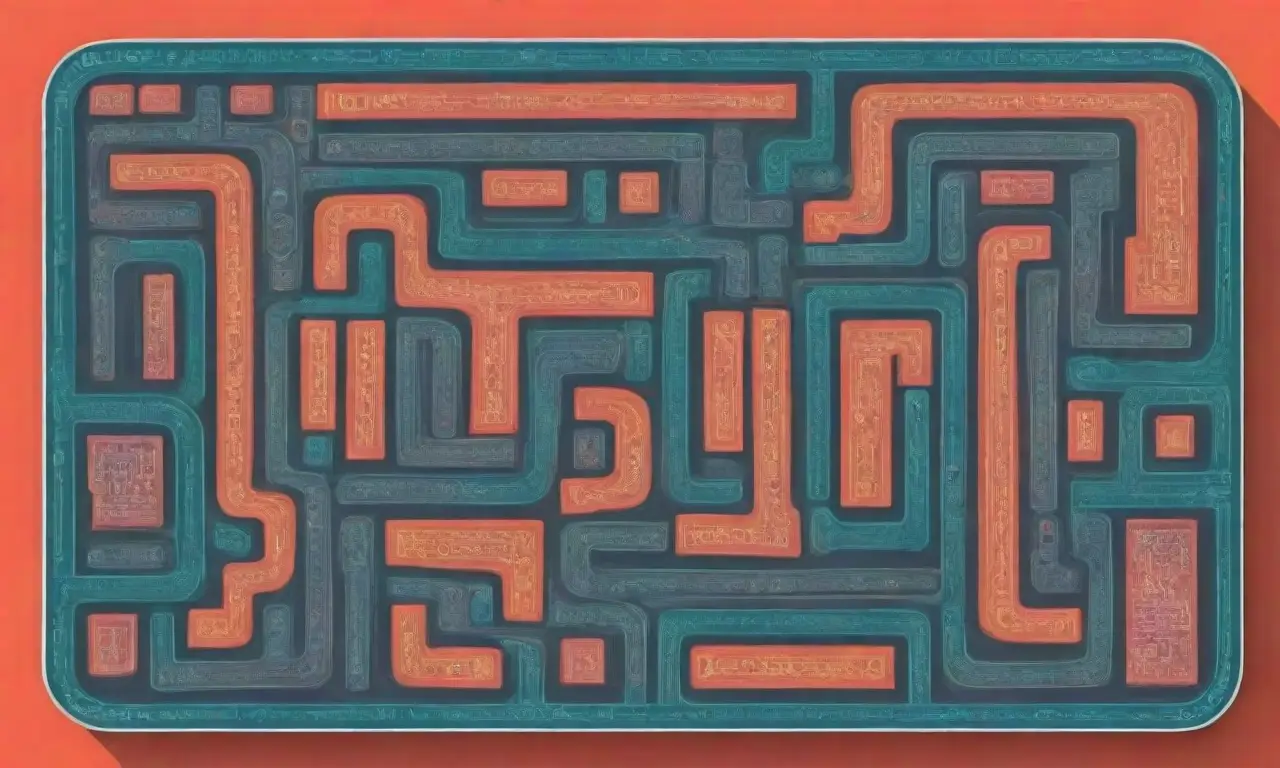
Technical Compatibility
Integrating machine learning tools with established support systems can be fraught with challenges, one of the most significant being technical compatibility. Legacy systems, in particular, may not easily interface with modern machine learning algorithms due to outdated technologies or lack of APIs. Organizations need to assess their existing technological infrastructure and determine whether it can be upgraded or if a full system overhaul is required to accommodate new solutions.
Furthermore, data silos can complicate integration efforts. When customer-related data is spread across various platforms—such as old CRMs, databases, and disparate systems—it becomes challenging to derive holistic insights that machine learning models require for effective functionality. Thus, companies must prioritize data unification before attempting integration, ensuring that their machine learning strategies have access to comprehensive and high-quality data.
Change Management
In many periods of technological advancement, managing the transition from traditional methods to modern approaches can be difficult for companies. Resistance to change among support teams and employees may hinder the effective implementation of machine learning tools. Staff may feel threatened by automation or may not fully understand how to utilize advanced technologies, which can lead to confusion and mixed morale.
To address this, organizations must invest in robust change management strategies. Training programs that educate employees on the benefits and functionalities of new machine learning tools can build greater acceptance. Open communication about the reasons behind the changes, fostering a culture of collaboration and continuous learning, is vital to ensure that teams feel empowered and are agile in adapting to new processes.
Real-time Customer Support: ML Models that Respond InstantlyEthical Considerations and Bias
Another critical challenge in the integration of machine learning tools lies in addressing ethical considerations, particularly concerning data privacy and bias. ML models are only as good as the data they are trained on; if that data reflects human biases, the model's outputs may inadvertently perpetuate unfair practices or stereotypes. For customer support systems, this can lead to unequal treatment of customers based on their profiles and backgrounds.
Companies must prioritize the ethical training of their machine learning algorithms by ensuring an unbiased dataset. It is essential to perform regular audits and assessments of the model outcomes to identify and rectify potential biases. Moreover, maintaining transparency with customers regarding how their data is used in optimizing support processes can bolster trust.
Best Practices for Successful Integration
Define Clear Objectives
Before embarking on the integration of machine learning tools with support systems, companies must clearly define their objectives. What specific challenges are you looking to solve? Is it enhancing response time, increasing resolution rates, or improving customer satisfaction? Defining specific goals allows for tailored strategies that focus on desired outcomes. This clarity is also essential in communicating the vision to team members and stakeholders, ensuring alignment across the board.
Pilot Programs
Introducing machine learning tools through a pilot program allows organizations to test their effectiveness without committing extensive resources upfront. By initially integrating ML solutions in small, controlled environments, companies can monitor and evaluate performance metrics before a larger rollout. This approach enables organizations to identify potential pitfalls and gather feedback that can be utilized to fine-tune functionalities.
Actionable Steps for Deploying ML in Customer Service TeamsAdditionally, piloting programs encourages collaborative involvement from support staff who will be using the machine learning tools. By involving frontline employees in testing phases, organizations gain valuable insights into user experience, paving the way for a smoother transition when the technology is fully implemented.
Continuous Monitoring and Improvement
Successful integration of machine learning tools does not end with deployment; continuous monitoring and improvement processes are fundamental. Collecting performance data and customer feedback post-integration is crucial in evaluating the effectiveness of the tools. Organizations should use established metrics—such as resolution times, customer satisfaction scores, and ticket volume trends—to assess success.
Regularly evaluating these metrics enables organizations to make data-driven decisions and iterate on machine learning models, refining algorithms to better serve customer needs. This ongoing commitment to improvement reaffirms a customer-centric approach and supports a culture of excellence within the support team.
Conclusion
Integrating machine learning tools with existing support systems is a transformative journey that holds great potential for enhancing customer service and operational efficiency. By automating processes, providing personalized experiences, and enabling data-driven decision-making, organizations can fundamentally elevate their customer support frameworks.
Optimizing Customer Support Workflows with Machine LearningWhile the benefits of integration are substantial, it is essential to navigate the accompanying challenges represented by technical compatibility, change management, and ethical considerations. By defining clear objectives, launching pilot programs, and committing to continuous improvement, organizations can effectively harness the power of machine learning.
As we move further into the digital age, organizations that embrace these advancements will remain competitive and responsive to customer needs. By embarking on this journey with preparedness and strategy, businesses can not only enhance their support functions today but also build a robust foundation for future innovations in customer care.
If you want to read more articles similar to Integrating Machine Learning Tools with Existing Support Systems, you can visit the Customer Support Automation category.
You Must Read