
Integrating Wearable Technology Data with Machine Learning Tools
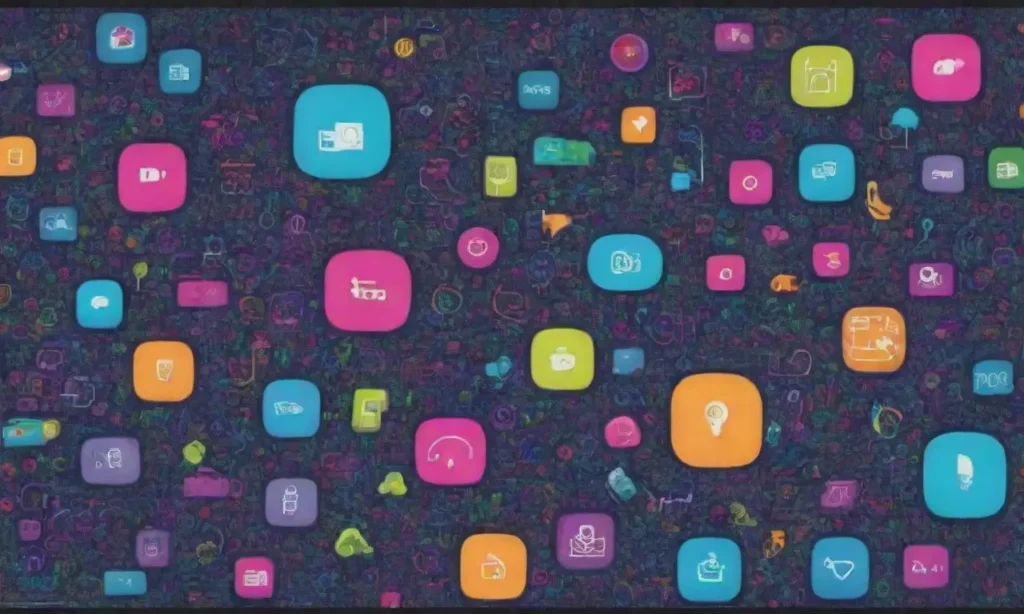
Content
Introduction
In recent years, the surge in wearable technology has transformed the way we gather and analyze health and fitness data. Devices like smartwatches, fitness trackers, and health monitors have become ubiquitous, offering a continuous stream of real-time data about various physiological metrics including heart rate, steps taken, sleep patterns, and even blood oxygen levels. The ability to collect this vast amount of data opens up new opportunities for analysis, insights, and ultimately better health outcomes.
This article delves into the integration of wearable technology data with machine learning tools, examining the potential benefits, challenges, and real-world applications. We will explore how the fusion of these technologies can empower individuals and organizations to make more informed decisions based on data-driven insights. By the end of this article, readers will have a comprehensive understanding of how wearable devices, coupled with machine learning, can enhance health monitoring and predictive analytics.
The Rise of Wearable Technology
Wearable technology has made significant strides since devices emerged on the market. Initially basic pedometers evolved into sophisticated gadgets that can track a multitude of metrics with astonishing accuracy. Smartwatches, for instance, are not just timekeepers; they are mini-computers strapped to our wrists, capable of monitoring cardiovascular health, tracking physical activity, and even sending notifications. Similarly, devices like the Oura Ring or Fitbit have expanded the range of health metrics available to users, collecting information about sleep cycles, temperature variations, and even stress levels.
The data collected by these devices is substantial and often updated in real time. This continuous stream of information creates a unique environment for data analysis. Instead of relying on sporadic health check-ups, individuals can now track their progress closely, allowing for a deeper understanding of how daily habits influence overall health. With the sheer volume of data being produced, however, comes the challenge of analysis. This is where machine learning shines, with algorithms capable of identifying patterns and gleaning insights that may go unnoticed through traditional analysis methods.
Statistical Models vs. Machine Learning in Sports Performance AnalysisIntegration not only amplifies the utility of wearable technology but can also add tremendous value to health-related services. Hospitals and fitness enterprises can leverage data from wearables to enhance patient care and customer engagement. For instance, telehealth can incorporate data from wearables to provide more personalized and timely interventions, establishing a proactive approach rather than a reactive one.
The Role of Machine Learning in Data Analysis
Machine learning, a subset of artificial intelligence, involves the use of algorithms and statistical models to allow computers to perform specific tasks without explicit instructions. In the realm of wearable technology, this capability is invaluable for extracting useful insights from the complex datasets these devices generate. Machine learning models can analyze the data collected over time, looking for trends or anomalies that can be indicative of potential health issues.
Types of Machine Learning Algorithms
When integrating data from wearable technology, various machine learning algorithms can be employed. Supervised learning involves training a model on a labeled dataset, allowing the model to make predictions based on new data. For example, a supervised learning model can be trained on historical health data to predict a user’s likelihood of developing a specific condition based on their wearable data.
In contrast, unsupervised learning analyzes data that is not labeled, enabling the model to identify hidden patterns. For instance, analysts can use clustering algorithms to group users based on similar activity patterns or health metrics, uncovering trends that may not have been previously recognized.
Leveraging Spatial Data for Enhanced Sports Performance AnalysisAnother approach is reinforcement learning, where the algorithm learns to make decisions by receiving feedback from its actions. This is particularly useful in dynamic environments like health monitoring, where behavior modification can lead to improved health outcomes through personalized recommendations based on real-time data.
Data Preprocessing and Feature Engineering
Before machine learning models can be applied to the data collected from wearables, it's essential to preprocess and cleanse the data. This involves removing any noise or irrelevant information, handling missing values, and possibly normalizing the data so that it can be utilized effectively by the algorithms.
Feature engineering is another essential step that involves selecting and manipulating variables to improve the performance of the machine learning models. For wearable devices, this may mean deriving new features. For instance, raw heart rate data can be transformed into metrics such as average heart rate during exercise, variability over time, or recovery heart rate. These engineered features can provide deeper insights into an individual’s health, allowing for more accurate modeling and predictions.
Combining data from different wearable devices can also result in richer datasets, which can contribute to the effectiveness of the machine learning algorithms. Moreover, the fusion of various data types—from biometric information such as heart rate and sleep quality to physical activity measures like daily steps—creates a comprehensive view of one’s health status.
Applications of Wearable Technology and Machine Learning Integration
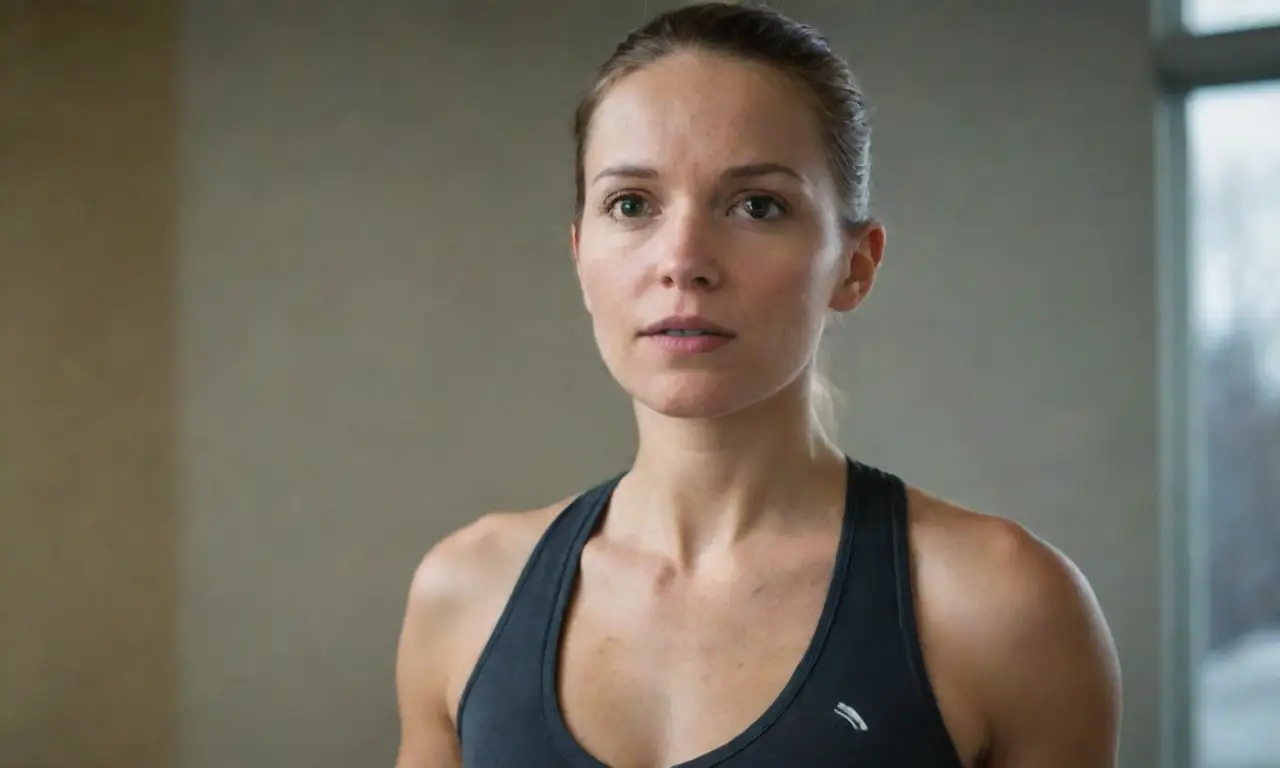
The combination of wearable technology and machine learning has practical applications across various domains but is particularly significant in health and fitness. For instance, predictive analytics can identify health patterns that lead to chronic conditions. By continuously monitoring users, machine learning models can analyze trends that may indicate increased risk factors—like abnormal heart rates or irregular sleep patterns—and alert healthcare providers or users themselves.
Remote Patient Monitoring
Telemedicine and remote patient monitoring are rapidly evolving fields where wearables play an integral role. By integrating machine learning tools with data collected via wearables, healthcare providers can continuously monitor patients’ health metrics without requiring them to be physically present in a hospital setting. For example, patients with chronic diseases can benefit from having their data monitored in real time, while physicians can receive alerts when a patient’s metrics deviate from established norms. This capability not only enhances patient care but can reduce healthcare costs significantly.
Moreover, machine learning models can assist in the development of personalized treatment plans. By analyzing data from wearables specific to an individual, healthcare providers can tailor interventions that align with that patient's unique health habits and lifestyle, leading to better compliance and outcomes.
Fitness and Performance Optimization
Athletes and fitness enthusiasts are another group that greatly benefits from the marriage of wearable technology and machine learning. By analyzing vast amounts of performance data, fitness trackers can provide insights into how specific workouts affect individuals' health metrics. Whether it’s assessing recovery times, analyzing heart rate zones during exercise, or optimizing training regimens based on individual metrics, machine learning tools help athletes fine-tune their performance.
For example, an individual preparing for a marathon can use wearable technology to track their training progress, monitor body fatigue levels, and predict their performance on race day. Machine learning algorithms can analyze their training data to determine the optimal pacing strategy or rest intervals, ensuring the best possible performance.
Mental Health Monitoring
Another arena where the combination of wearable technology and machine learning can have an unprecedented impact is in mental health. Wearable devices can track physiological indicators linked to mental states, such as heart rate variability and sleep quality. Machine learning can analyze these metrics to identify signs of stress, anxiety, or depression.
Such monitoring can facilitate timely interventions, enabling individuals to seek help before conditions escalate. Additionally, algorithms designed to analyze speech or text in conjunction with wearable data can provide comprehensive insights into mental health trends over time. For a more robust solution, integrating wearables with mental health applications can create a holistic approach to wellbeing.
Conclusion
The integration of wearable technology data with machine learning tools represents a transformative shift in how we monitor, analyze, and ultimately enhance our health. This convergence not only enables more personalized health monitoring and interventions but also brings predictive analytics into the forefront, allowing for action before health issues develop into more serious conditions.
However, with exciting advancements come challenges such as data privacy concerns, the need for interoperability among devices, and the requirement for robust machine learning algorithms that can effectively utilize the data generated by wearables. Striking a balance between technological innovation, user convenience, and ethical considerations will be paramount as we move forward.
As we embrace this new digital healthcare age, it is essential for stakeholders—be it healthcare providers, technology developers, or patients—to work together to maximize the benefits while addressing the ethical implications of data use. Ultimately, the goal is a healthier society empowered by technology that understands and promotes individual health and wellness like never before.
If you want to read more articles similar to Integrating Wearable Technology Data with Machine Learning Tools, you can visit the Sports Analytics category.
You Must Read