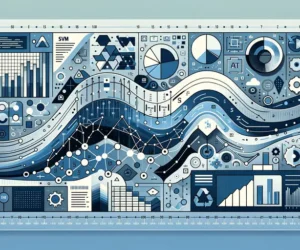
Leveraging NLP to Enhance Financial Market Sentiment Analysis
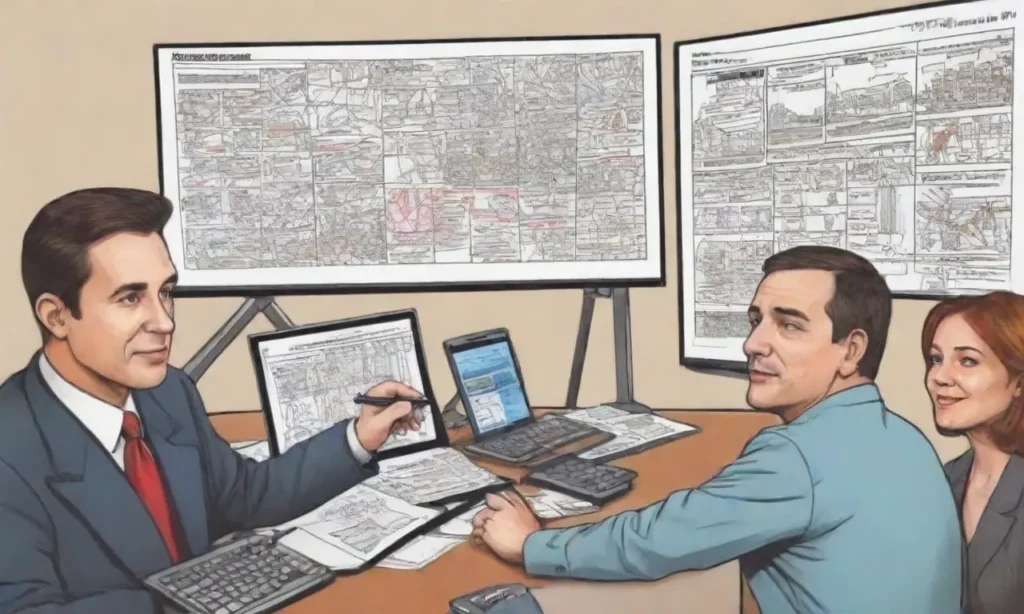
Introduction
In today's rapidly evolving financial landscape, the ability to swiftly analyze market sentiment is more crucial than ever. Financial institutions, traders, and investors all rely on sentiment analysis to effectively gauge public opinion, predict market trends, and make informed investment decisions. With the advent of Natural Language Processing (NLP), a subfield of artificial intelligence concerned with the interaction between computers and human languages, sentiment analysis has become not just feasible but increasingly sophisticated. This powerful technology unlocks insights from unstructured data sources like news articles, social media, earnings reports, and analyst opinions, enabling market participants to discern the emotional tone surrounding various financial instruments.
This article aims to delve deep into the intersection of NLP and financial market sentiment analysis, exploring how NLP techniques can enhance the accuracy and effectiveness of sentiment assessments. We will investigate various NLP methodologies, including text preprocessing, sentiment classification, and advanced deep learning models, while also discussing the practical implications of these advancements for traders and analysts alike. By the end of this exploration, you'll understand how leveraging NLP can lead to better decision-making in the financial markets and what future trends may shape the industry.
Understanding Sentiment Analysis in Finance
Sentiment analysis refers to the computational study of opinions, sentiments, and emotions expressed within textual data. In the context of financial markets, it involves evaluating the sentiments linked to specific investments or the broader economy. The primary goal is to capture the motivations of investors, the mood of the market, and any external factors like political events or economic reports that may influence these sentiments. The rise of social media and the digital economy has fundamentally transformed how sentiments can be captured and analyzed, as vast quantities of data are now generated at an unprecedented pace.
Sentiment analysis relies on specific methods like lexicon-based approaches and machine learning to ascertain the sentiment orientation (positive, negative, or neutral) of a given piece of text. Lexicon-based approaches utilize pre-defined vocabulary lists that assign sentiments to words. While these methods can be effective for straightforward texts, they often fall short when dealing with the complexities of financial language, where context and nuances play significant roles. For instance, the word "losing" in the sentence "The stock is losing ground" carries a different connotation than in "The company is losing focus on key projects."
Advanced Techniques for Predicting Currency Exchange RatesOn the other hand, machine learning-driven techniques allow for a much richer analysis, learning patterns in the data automatically. These methods use historical data to train classification models that can predict sentiments based on features extracted from text. The increasing availability of labeled datasets and advancements in computing power have made it easier for analysts to implement machine learning approaches, significantly improving the accuracy of sentiment analysis in financial applications.
Natural Language Processing Techniques for Sentiment Analysis
Text Preprocessing
Before diving into sentiment analysis, it is essential to engage in text preprocessing, which involves cleaning and preparing the data for analysis. The primary steps include tokenization, removal of stop words, stemming or lemmatization, and vectorization. Tokenization is the process of splitting text into individual words or phrases, enabling the model to analyze them independently. For instance, the sentence "The stock value has drastically increased" would be tokenized to "The," "stock," "value," "has," "drastically," and "increased."
The next key step is stop-word removal, where commonly occurring words (such as "and," "the," "is") are discarded because they add little meaning in sentiment analysis. This ensures that more informative words are analyzed, enhancing the overall efficacy of the model. Following this, stemming or lemmatization converts words to their base or root forms, which helps in normalizing the text. For example, "running," "ran," and "runs" would all be transformed to "run," allowing the model to focus on the sentiment rather than variations of the same word. Lastly, vectorization transforms the textual data into a numerical format that the machine learning algorithms can understand, using methods such as Bag-of-Words or TF-IDF (Term Frequency-Inverse Document Frequency).
Sentiment Classification Models
With preprocessed text in hand, the next phase involves constructing sentiment classification models. Various algorithms can be employed for this purpose, including Logistic Regression, Naive Bayes, and Support Vector Machines (SVM). Each of these models has its strengths and weaknesses, with Logistic Regression being particularly popular due to its simplicity and interpretability. Naive Bayes, on the other hand, benefits from its speed and efficiency, making it suitable for larger datasets.
Practical Applications of Random Forests in Financial ForecastingFor more complex sentiment analysis tasks, advanced methods like Recurrent Neural Networks (RNNs) and Long Short-Term Memory (LSTM) networks can be employed. These models are capable of understanding sequences, making them particularly adept at processing text data where context and order matter. Furthermore, the development of Transformer architectures, such as BERT (Bidirectional Encoder Representations from Transformers), has revolutionized the field by allowing models to capture not only the meaning of individual words but also their contextual relationships. Thanks to this capability, BERT can effectively handle sentiments embedded within intricate financial texts, delivering much more nuanced results.
Incorporating Alternative Data Sources
In today's financial ecosystem, traditional data sources like historical prices and trading volumes may no longer provide the complete picture of market sentiment. This is where the incorporation of alternative data sources becomes critical. Financial analysts can extract valuable insights from various platforms, including Twitter, Reddit, and financial news articles, enabling them to gauge public sentiment on financial instruments or market conditions in real time.
For example, social media platforms like Twitter have emerged as a valuable tool for sentiment analysis, as they provide rich and timely data about public opinions. By collecting tweets related to certain stocks or commodities, analysts can glean insights into investor attitudes before official price movements occur. This is especially pertinent in high-volatility situations, such as earnings announcements or geopolitical events, where public sentiment can shift dramatically.
However, extracting and analyzing sentiments from alternative data sources poses unique challenges. The need to filter out noise, disinformation, and irrelevant content necessitates the use of effective NLP techniques for data filtering and refinement. Here, techniques like social media listening tools and sentiment classifiers specifically trained on these datasets become integral. By effectively capturing and interpreting these insights, financial professionals can enhance their situational awareness and thus make more informed decisions.
Using Genetic Algorithms for Stock Market Strategy DevelopmentChallenges and Limitations in NLP for Sentiment Analysis

Ambiguity and Sarcasm Detection
Despite the leaps in technology, challenges remain inherent in the application of NLP for sentiment analysis. One of the foremost issues is dealing with ambiguity in the language; words or phrases can convey different meanings depending on the context in which they are used. A phrase like "This stock is a gem" could be viewed positively or negatively based on the tone and wider context. Similarly, sarcasm poses a significant barrier—sarcastic remarks can easily be misclassified, leading to incorrect sentiment assessments. Modern NLP models, while more adept at contextual understanding, still grapple with these nuances, underscoring the need for further development and refinement.
Data Quality and Volume
The quality and volume of data used in sentiment analysis can also significantly impact the accuracy of predictions. Financial texts are often characterized by jargon, domain-specific language, and complex structures. Models trained on generalized datasets may not perform well when applied to specialized financial texts without additional fine-tuning. Additionally, the financial landscape is subject to rapid changes; thus, the models must be updated continually with new data to remain relevant. Continuous monitoring and retraining are vital to ensure that the sentiment analysis remains aligned with the ever-evolving financial narrative.
Ethical Considerations and Data Privacy
Lastly, it's crucial to highlight the ethical considerations surrounding the use of NLP for sentiment analysis. The gathering of data from social media and online platforms raises questions about data privacy, informed consent, and accountability. Companies utilizing such data must ensure compliance with regulations such as GDPR (General Data Protection Regulation) while also addressing potential biases in their datasets, as this can lead to skewed analyses. In an era where ethical finance is gaining traction, ensuring that sentiment analysis practices are ethically sound can enhance trust and credibility among market participants.
The Use of Gradient Boosting for Investment Risk AssessmentConclusion
The fusion of Natural Language Processing (NLP) and sentiment analysis represents a transformative shift for financial market intelligence. As this technology continues to evolve, investors and analysts are better equipped to parse the complexities of market sentiment, enabling them to make informed decisions in a timely manner. From sophisticated preprocessing techniques to advanced machine learning models and the integration of alternative data, the potential for enhanced understanding of market dynamics is increasingly within reach.
Despite the inherent challenges, the future of sentiment analysis in finance appears promising. The ongoing development of context-aware models, improvements in data quality, and the rise of ethical guidelines for data usage will likely herald a new era in financial analysis. As firms and individuals leverage NLP for deeper insights, those who adapt quickly gain a competitive edge, making informed decisions based on real-time sentiment evaluations.
In summary, leveraging NLP to enhance financial market sentiment analysis provides an innovative pathway for traders and financial analysts alike. By adopting these advanced methodologies while considering ethical and practical implications, they can significantly improve their outcomes in an increasingly data-driven market landscape. The journey into the future of financial analytics is bright, filled with opportunities for those willing to embrace technology in their decision-making processes.
The Synergy of AI and Financial Engineering in Forecasting ModelsIf you want to read more articles similar to Leveraging NLP to Enhance Financial Market Sentiment Analysis, you can visit the Financial Forecasting category.
You Must Read