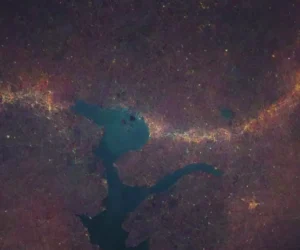
Navigating Customer Queries: ML Models that Transform Support
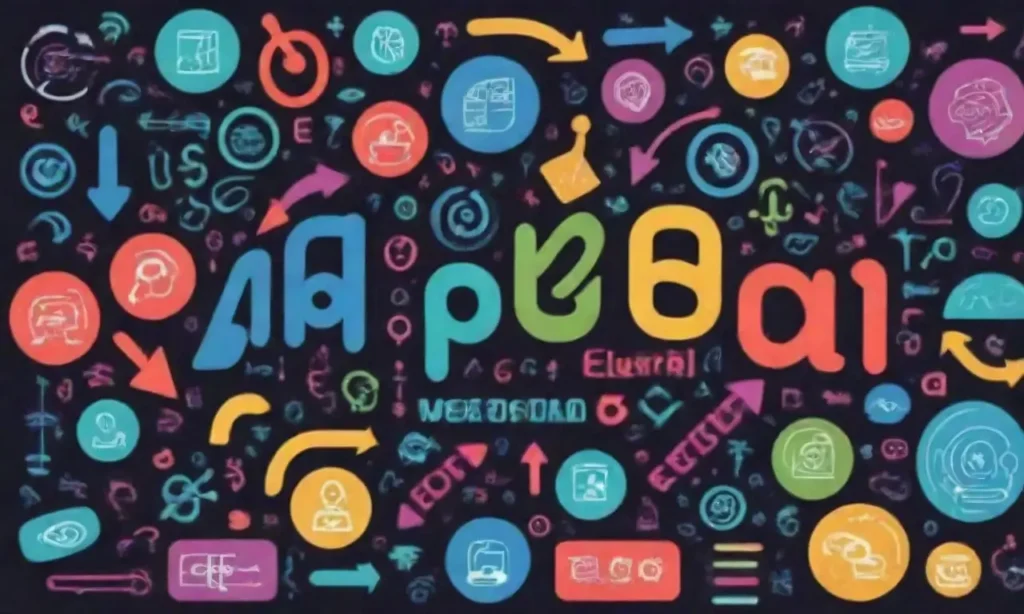
Introduction
In today's world, where real-time communication is the backbone of customer satisfaction and retention, navigating customer queries has become increasingly complex. Organizations are inundated with an overwhelming volume of inquiries spanning various platforms, from social media to traditional email. Apart from the sheer volume, the nature of these queries can range from simple FAQs to more complex concerns requiring thorough investigation. This changing landscape has necessitated organizations to evolve, leaning towards more efficient and innovative solutions.
In this article, we will delve into how Machine Learning (ML) models are radically transforming customer support systems. We will explain the nuanced ways in which these technologies can streamline operations, enhance user experiences, and ultimately drive business success. The conversation will also touch on various ML techniques, real-world applications, and the future implications of such technology in customer support.
The Necessity of Machine Learning in Customer Support
The rapid expansion of digital channels has brought a new set of challenges for customer support teams. Traditional customer service methods are often inadequate for addressing the speed and complexity of modern customer queries. Searching through extensive libraries of FAQs or relying solely on human agents can lead to slow response times and, consequently, a decline in customer satisfaction and loyalty.
Understanding Customer Needs with Data
Data analysis plays a crucial role in understanding customer needs and behaviors. By employing ML algorithms, organizations can analyze large datasets to identify patterns and predict customer inquiries. For instance, sophisticated ML models can evaluate historical data to discern trends in customer behavior, which can aid support teams in crafting proactive solutions.
Boosting Customer Engagement through ML-Powered ChatbotsOne prominent example is using sentiment analysis to gauge customer emotions behind inquiries. By deploying ML algorithms that analyze language patterns, support teams can not only address issues but also tailor their approach based on the customer's emotional state. This data-driven response not only enhances the individual experience but also fosters a stronger relationship between the brand and the consumer.
Automating Responses: The Rise of Chatbots
One of the most apparent evolutions in customer support is the rise of chatbots. Powered by ML, these automated systems can handle a multitude of basic queries without human intervention. Chatbots are equipped with Natural Language Processing (NLP) capabilities, enabling them to understand and simulate human conversation.
These systems are continually learning and improving based on customer interactions. Through reinforcement learning, they refine their responses, effectively building a more comprehensive understanding of customer intentions over time. Not only does this reduce the load on human agents, but it also allows customers to receive immediate answers regardless of the time of day.
Reducing Human Error
Reliance on human agents, while sometimes necessary, can lead to inconsistencies in responses and errors in judgment. By utilizing ML models, businesses can minimize these discrepancies, ensuring that customers receive accurate and standardized information consistently.
Integrating Machine Learning Tools with Existing Support SystemsFor example, an ML model can be trained on a database of past customer queries and solutions. Whenever a new query arises, the model references its training to deliver the most relevant and accurate response. This is particularly beneficial in organizations facing a high volume of repetitive inquiries, where accuracy is paramount to maintaining customer trust and satisfaction.
Techniques Employed in Machine Learning Models
To unlock the full potential of ML in customer support, various techniques can be employed. Understanding these techniques not only illuminates the workings behind successful implementations but also highlights areas for further enhancement.
Natural Language Processing (NLP)
NLP is a branch of ML focused on the interaction between computers and human language. In customer support, NLP algorithms can be employed to comprehend, interpret, and generate human language in a valuable way.
For instance, when customer queries are submitted via chat, social media, or email, NLP algorithms can analyze the content to extract important keywords and intent. This allows customer support systems to categorize inquiries efficiently, routing them to the correct department or providing instant answers operated by chatbots. By breaking down language barriers, NLP plays a pivotal role in ensuring that clients receive the timely assistance they require.
Building Better Customer Relationships Through ML InsightsPredictive Analytics
Predictive analytics harnesses the power of statistical algorithms and machine learning techniques to identify the likelihood of future outcomes based on historical data. This is especially useful in customer support; by analyzing past interactions, organizations can anticipate potential customer issues and devise preventative measures.
For example, if data shows an uptick in complaints related to a specific product, organizations can proactively reach out to affected customers with solutions or helpful information before they even need to contact support. This forward-thinking approach allows businesses to demonstrate that they prioritize customer satisfaction, effectively reinforcing brand loyalty.
Decision Trees and Random Forests
Decision Trees and Random Forest models are popular techniques within ML suited for classification tasks, including customer query handling. Decision Trees outline a simple flow of questions and answers that guide the model in making predictions for various inputs.
In the context of customer support, a Decision Tree can help classify queries into various categories (e.g., billing, technical support) based on initial customer inputs. Random Forests improve upon this by creating multiple decision trees, thus increasing the robustness of predictions. These methods not only make it easier to handle inquiries but also assist support teams in tracking and analyzing trends across various categories and subdivisions of queries.
Exploring the Intersection of ML and Human Touch in SupportReal-World Applications of ML in Customer Support
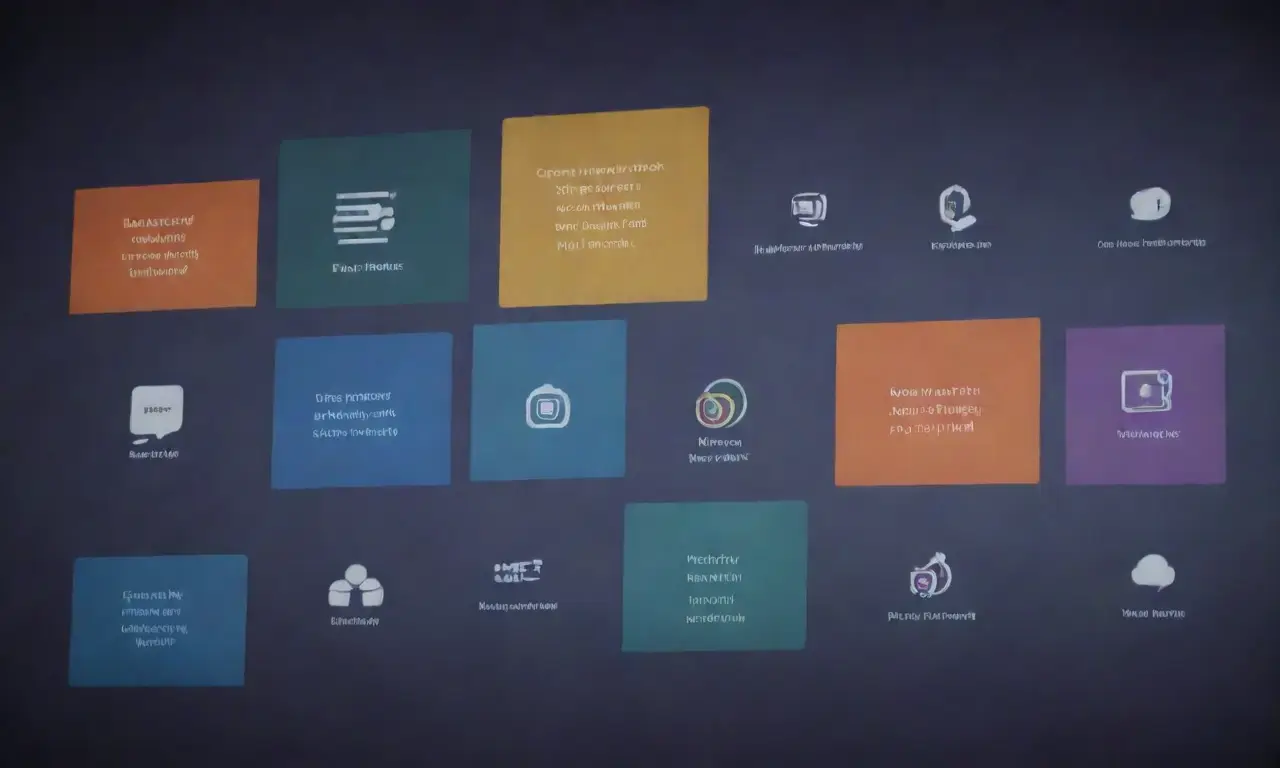
As organizations increasingly adopt ML models in their customer support processes, various real-world applications have emerged, showcasing the technology's wide-ranging effectiveness.
Enhanced Customer Interactions
A prime example is the retail industry, in which leading brands have deployed sophisticated ML chatbots to assist customers. For instance, the clothing retailer H&M utilizes a chatbot to help customers navigate through their inventory, assisting them in finding suitable clothes based on their preferences. By analyzing previous customer interactions, the ML system can make personalized recommendations, enhancing user experience exponentially.
Fraud Detection in Financial Services
In financial services, ML models are pivotal for detecting fraudulent activities. By analyzing customer transaction data, these models facilitate real-time alerts when behaviors deviate from established patterns. In supporting customers during fraudulent inquiries, organizations can respond more rapidly, minimizing financial losses while bolstering trust. This not only helps prevent fraud but also provides customers with peace of mind regarding their transactions and account security.
Creating Effective ML Workflows for Customer Support AutomationStreamlined Helpdesk Solutions
Companies like Zendesk are leveraging ML to streamline their helpdesk solutions. By implementing a unified platform where customers can submit requests via multiple channels, the system intelligently categorizes inquiries and assigns priority levels, all through ML algorithms. This allows support teams to address customer concerns based on urgency and historical context, ensuring that even critical issues are swiftly resolved.
Conclusion
As we have explored throughout this article, navigating customer queries in today’s complex landscape requires innovative solutions to enhance efficiency and maintain customer satisfaction. Machine Learning is poised to lead this transformation by automating responses, predicting customer needs, and refining interactions through increasingly sophisticated techniques.
The integration of ML models in customer support not only alleviates the pressures faced by support teams but also enhances customer experiences through timely and accurate responses. From NLP for understanding customer intent to predictive analytics shaping proactive measures, the capabilities of ML are vast and continuously evolving.
Importantly, the adaptation towards ML in customer support does not signify the disbanding of human agents; rather, it complements their expertise. Key to maintaining a successful support system lies in balancing automation with human empathy and problem-solving skills. Organizations focusing on achieving this balance stand to benefit tremendously in customer loyalty and overall satisfaction.
Real-time Customer Support: ML Models that Respond InstantlyThe future of customer support systems is undoubtedly entwined with Machine Learning advancements. As technology continues to evolve, we can anticipate even more innovative applications of ML in customer engagement, propelling organizations beyond traditional support and into a realm of unparalleled customer experiences. Ultimately, the journey of harnessing ML in customer support is not merely about efficiency—it's about cultivating meaningful interactions that resonate with customers and reinforce their loyalty to brands.
If you want to read more articles similar to Navigating Customer Queries: ML Models that Transform Support, you can visit the Customer Support Automation category.
You Must Read