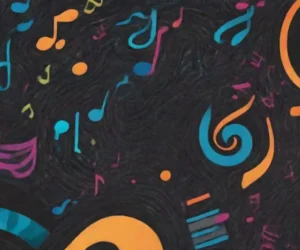
Optimizing Warehouse Operations with ML-Based Inventory Solutions
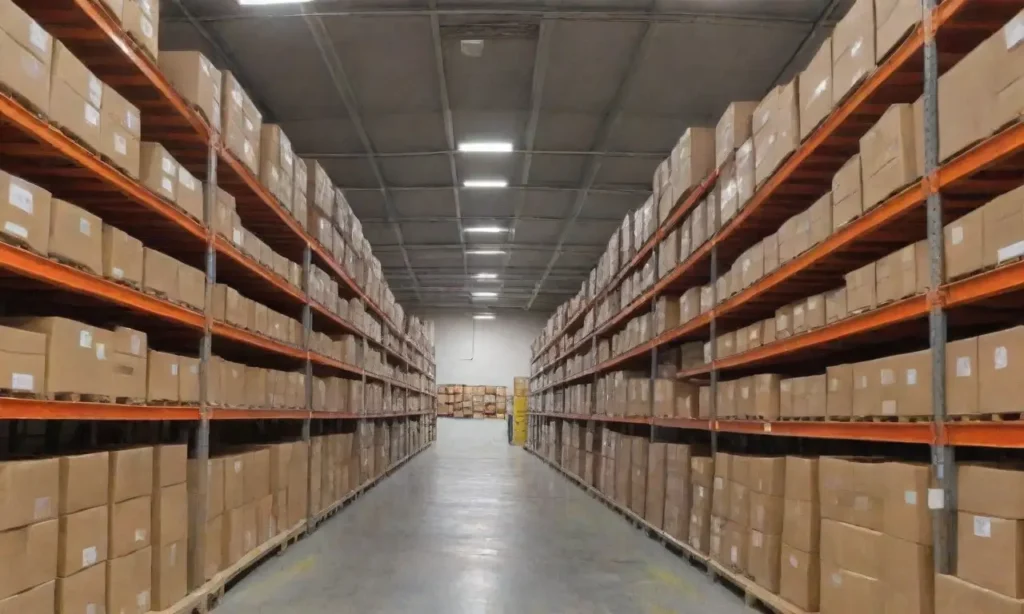
Introduction
In the fast-paced world of logistics and supply chain management, warehouse operations play a pivotal role in maintaining efficiency and cost-effectiveness. As businesses strive to keep up with customer demands while minimizing overheads, the stakes have never been higher. In this context, many organizations are turning to Machine Learning (ML)-based inventory solutions to streamline their processes and optimize warehouse performance.
This article will delve into how ML-based inventory management systems can revolutionize warehouse operations. We will explore the key benefits, the technology behind ML algorithms, the challenges in implementing these solutions, and real-world applications of ML within warehouse settings. By the end of this comprehensive discussion, you will have a solid understanding of how employing ML can significantly enhance the operational efficiency of warehouses.
The Importance of Inventory Optimization
A critical function of warehouse management is inventory optimization. This involves maintaining the ideal balance of stock to meet customer demand without tying up too much capital in unsold goods. Efficient inventory management not only improves cash flow but also reduces storage costs and minimizes loss due to obsolescence or spoilage.
One of the primary objectives of optimizing inventory is to enhance order fulfillment rates. When inventory levels are accurately forecasted, organizations can ensure that products are available when customers need them, leading to improved customer satisfaction. High fulfillment rates reflect positively on a company's reputation, helping to retain existing clients and attract new ones. In contrast, poor inventory management can result in lost sales opportunities when stock is unavailable or deteriorating customer trust due to delays.
Improving Overstock and Understock Situations Using AI MethodsFurthermore, effective inventory optimization is instrumental in reducing waste and lowering operational costs. By leveraging Machine Learning, companies can analyze vast datasets to understand historical demand patterns and forecast future needs, thereby making informed stock management decisions. The predictive power of ML can decrease the likelihood of overstocking or understocking, ultimately resulting in a more efficient warehouse operation.
How Machine Learning Enhances Inventory Management
Predictive Analytics
One of the most significant advantages of using ML in inventory management is the capability of predictive analytics. Traditional inventory systems often struggle with the unpredictable nature of consumer demand. Using historical data, ML algorithms can identify patterns and trends that may not be readily visible to human operators. For instance, they can analyze seasonal fluctuations, promotional events, and even economic indicators to offer accurate demand forecasts.
By implementing ML-based predictive analytics, businesses can make proactive decisions regarding order placements and stock replenishments. Instead of relying solely on historical sales data, organizations can take into account various external factors affecting demand. This leads to a more dynamic and responsive inventory management strategy, preventing both excess stock and stockouts.
Moreover, predictive analytics aids in demand sensing, a critical aspect for industries like retail, where consumer preferences can change rapidly. This enables companies to shift resources swiftly and align their inventory levels with the most current market demands.
Automated Inventory Management
The second area where ML provides significant benefits is in automated inventory management processes. With the rise of AI technologies, automated systems can take over routine tasks that were once performed manually. This includes inventory tracking, reordering products, and data entry, thus reducing the risk of human error.
Automation powered by ML means that stock levels can be continuously monitored in real-time. When inventory levels drop below predefined thresholds, the system automatically generates restocking orders, ensuring that products are always available when needed. This not only saves time for warehouse staff but also eliminates delays in restocking processes, leading to greater operational efficiency.
Additionally, automated inventory management leverages technologies like RFID scanners and IoT sensors, which can track product movement throughout the warehouse. With these advancements, stakeholders can gain insights into inventory turnover rates, helping identify slow-moving products that may need to be discounted or promoted more heavily.
Smart Warehousing Innovations
A further dimension of ML application in inventory management is the rise of smart warehousing. This futuristic approach combines ML algorithms with warehouse automation technologies, creating environments where operations are guided by data-driven insights.
For instance, ML can optimize the layout of a warehouse, ensuring that high-demand items are stored in easily accessible locations, reducing the time workers spend picking orders. This optimization can extend to delivery scheduling as well, with ML algorithms helping to devise the most efficient routes for trucks based on traffic conditions and delivery windows.
Moreover, predictive maintenance is another essential component of smart warehousing. By analyzing usage patterns of machinery and equipment, ML can foresee potential failures before they occur. This preemptive approach can lead to significant cost savings and reduced downtime, allowing operations to run smoothly without interruptions.
Challenges in Implementing ML-Based Inventory Solutions
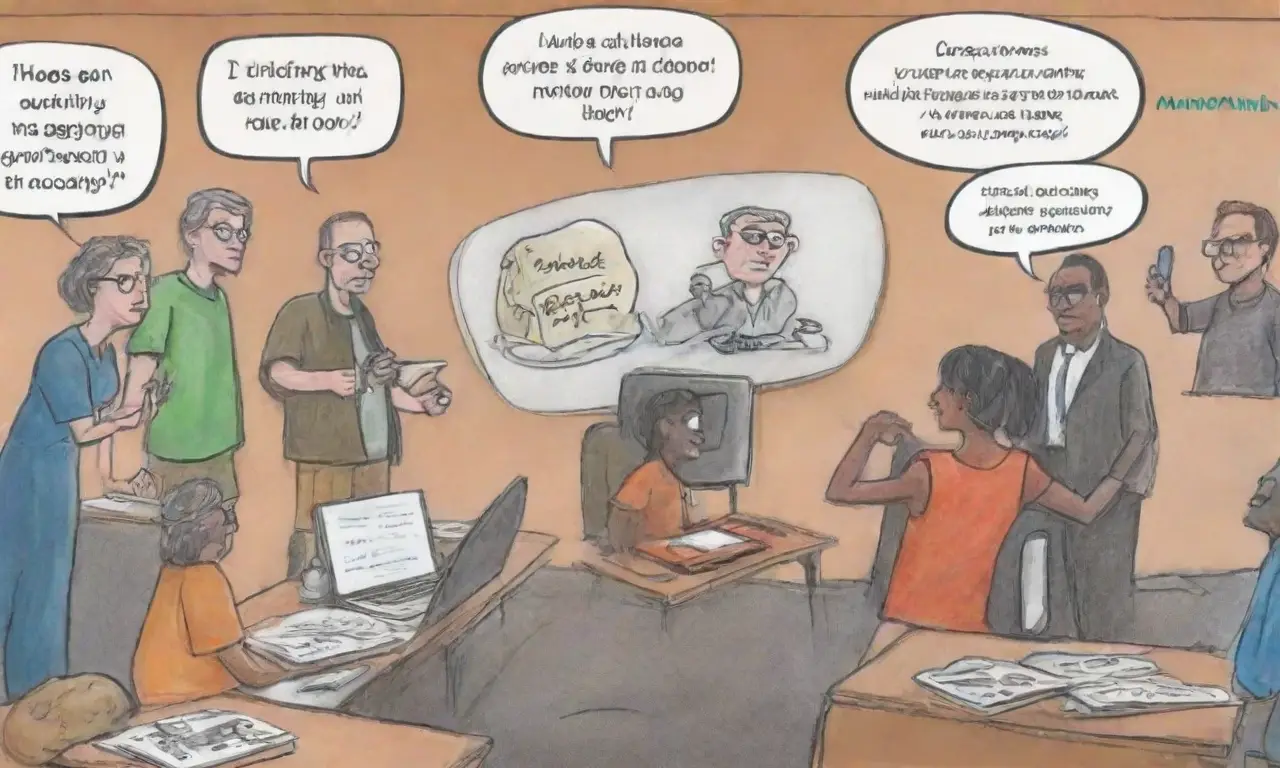
Data Management Issues
Despite its numerous advantages, implementing ML-based inventory solutions does not come without challenges. One of the primary concerns is data management. For ML algorithms to function effectively, vast amounts of accurate and relevant data must be collected and processed. This requires proper data infrastructure and governance, as well as strategies to manage and integrate disparate data sources.
Companies often struggle with data silos, where information is isolated within different departments or systems. To harness the full potential of ML, organizations must ensure a consolidated and cohesive dataset that reflects the realities of inventory and customer behavior. Data quality is equally critical; outdated or erroneous data can lead to inaccurate forecasts that may negatively impact inventory levels.
Resistance to Change
Another hurdle in adopting ML technology is the resistance to change within organizations. Employees may be skeptical about new technologies, particularly when they perceive these tools as a threat to their roles. Overcoming this resistance requires effective change management strategies that foster adaptability and upskill staff to work alongside ML systems. Moreover, educating employees about the potential benefits of these technologies can play a crucial role in easing fears and promoting acceptance.
Leaders should also emphasize that ML is designed to enhance human decision-making, rather than replace it. Building a culture of innovation that embraces technological advancements as a means of empowerment can help create a more conducive environment for ML adoption.
Initial Costs and Investment
Lastly, the initial costs associated with implementing ML-based inventory solutions can be daunting for many organizations, particularly small to medium-sized enterprises. Investing in the necessary technology, infrastructure, and skilled personnel can strain budgets and resources. However, businesses should look at these investments as long-term commitments that will yield significant returns through enhanced efficiency and cost savings.
Financial models that highlight the return on investment (ROI) from optimized inventory management can help decision-makers understand the value of adopting ML solutions. It’s about balancing the upfront capital outlay with potential operational improvements that, over time, can far outweigh the initial expenditures.
Conclusion
The integration of Machine Learning into warehouse operations represents a revolutionary step towards achieving greater efficiency and effectiveness in inventory management. By employing ML-based solutions, organizations can utilize predictive analytics, automation, and smart technology innovations to optimize their warehousing processes.
As businesses navigate the ever-evolving landscape of consumer demands and supply chain complexities, the advantages of enhanced forecasting, automated tracking, and intelligent decision-making become increasingly vital. However, companies must also be proactive about addressing the challenges associated with data management, employee adaptation, and initial investments.
In conclusion, the future of warehouse operations appears to be firmly linked with ML technologies. As more organizations recognize the potential benefits of leveraging advanced analytics and artificial intelligence, we can anticipate a new standard for operational excellence in supply chain management. By embracing these innovations, businesses will not only optimize their inventory processes but also enhance their overall competitive edge in today's marketplace.
If you want to read more articles similar to Optimizing Warehouse Operations with ML-Based Inventory Solutions, you can visit the Inventory Management category.
You Must Read