
Personalized Marketing: Effective Strategies Utilizing Machine Learning

Content
Introduction
In the fast-paced realm of modern marketing, personalization has emerged as a transformative strategy that resonates with consumers on a deeper level. By leveraging cutting-edge technologies, particularly Machine Learning (ML), marketers are now able to dissect vast troves of data to deliver tailored experiences that meet individual preferences. As audiences increasingly demand content that feels relevant and engaging, the incorporation of ML into marketing strategies is not just an option but a necessity for brands striving to stand out in a crowded marketplace.
This article aims to delve into the world of personalized marketing and explore effective strategies that harness the power of machine learning. We will discuss various approaches brands can take to implement personalized marketing campaigns, the role of ML in customer segmentation, predictive analytics, and enhance consumer interactions. By understanding these strategies and the technologies driving them, businesses can create marketing efforts that are more effective, relevant, and ultimately successful.
Understanding Personalized Marketing and Its Importance
Personalized marketing is a strategy that tailors marketing messages, content, and product offerings to the preferences and behaviors of individual consumers. By leveraging data from various sources such as purchase history, browsing behavior, and social media interactions, brands can create a more engaging and relevant user experience. This approach has shown significant improvements in customer satisfaction, loyalty, and conversion rates.
One of the primary benefits of personalized marketing is the ability to create a strong connection with customers. When consumers feel like a brand understands their needs and preferences, they are more likely to engage with that brand. Additionally, personalized marketing allows for more effective targeting, reducing the wastage of marketing budgets by ensuring that the right messages reach the right audiences at the right time. Such precision can lead to improved customer acquisition and retention rates, making it a crucial aspect of contemporary marketing strategies.
An Architect's Guide to Developing Personalization AlgorithmsMachine learning is at the forefront of this personalization revolution. It enables marketers to analyze vast datasets quickly and effectively, uncovering hidden patterns and insights about consumer behavior. As ML algorithms become increasingly sophisticated, they provide the ability to not only predict what customers may want but also to automate and optimize marketing efforts in real-time. This combination makes machine learning an indispensable tool for any brand looking to enhance its personalized marketing initiatives.
Key Strategies for Implementing Personalized Marketing with ML
To effectively harness machine learning for personalized marketing, brands can utilize several strategies. Each of these strategies taps into the capabilities of ML to deliver tailored experiences and improve customer engagement.
Customer Segmentation
Customer segmentation is the process of dividing a customer base into distinct groups based on shared characteristics. ML algorithms enhance this process by analyzing extensive datasets to uncover nuanced insights that traditional segmentation methods may overlook. By employing clustering techniques, brands can categorize customers based on demographics, purchasing behavior, preferences, and even psychographics.
For instance, a fashion retailer might use machine learning to segment its customers into groups such as “frequent buyers,” “occasional shoppers,” and “bargain hunters.” Each group would respond differently to marketing messages, and through targeted campaigns, the retailer can customize its approach. Frequent buyers might receive exclusive previews of new collections, while bargain hunters might receive notifications on discounts and special sales.
The Role of Machine Learning in Creating Tailored User ContentFurthermore, this segmentation can be dynamic, allowing businesses to adjust their strategies according to changes in consumer behavior. With ML algorithms continuously learning from new data, customer segments can be updated in real-time, ensuring that brands are always aligned with their audiences' current preferences.
Predictive Analytics
One of the most powerful applications of machine learning in personalized marketing is predictive analytics. This involves using historical data and machine learning models to forecast future consumer behavior. By understanding trends and predicting what products or services a customer may be interested in, brands can tailor their marketing strategy accordingly.
For example, an e-commerce platform can analyze customers’ past purchases and browsing history to identify potential future purchases. The platform may find that a customer who frequently buys hiking gear is likely to be interested in camping equipment or outdoor apparel. Machine learning models can be used to compel recommendations, improve upselling and cross-selling efforts, and create timely email reminders about relevant products.
Moreover, predictive analytics allows brands to proactively address customer needs. By anticipating when a customer might need a refill of a product or when they might be looking for complementary items, brands can reach out to customers with personalized messages that drive engagement. This not only improves the customer experience but also encourages repeat business.
Personalized User Interfaces: Enhancing Usability Through AlgorithmsReal-Time Personalization
In today’s digital landscape, real-time personalization has become crucial. With machine learning, companies can tailor experiences in real-time based on immediate customer interactions. For instance, personalization can occur on web pages, in emails, or during live chat interactions. This ensures that customers receive information that is relevant not only based on their previous behaviors but also on their current activities.
A classic example can be found on streaming platforms like Netflix. When a user logs in, machine learning algorithms analyze their viewing history, preferences, and even the time of day to display recommended content tailored to that moment. If a user has recently watched sci-fi movies, the platform might prioritize recommending similar genres during their next visit, effectively maximizing their engagement.
Moreover, companies can implement real-time adjustments to promotions and offers based on a user’s on-site behavior. If a user lingers on a product page or adds an item to their cart without completing the purchase, a targeted ad or notification can pop up, offering a discount or highlighting complementary products. This responsiveness powered by machine learning not only improves conversion rates but also enhances customer satisfaction.
Challenges of Implementing Personalized Marketing with ML
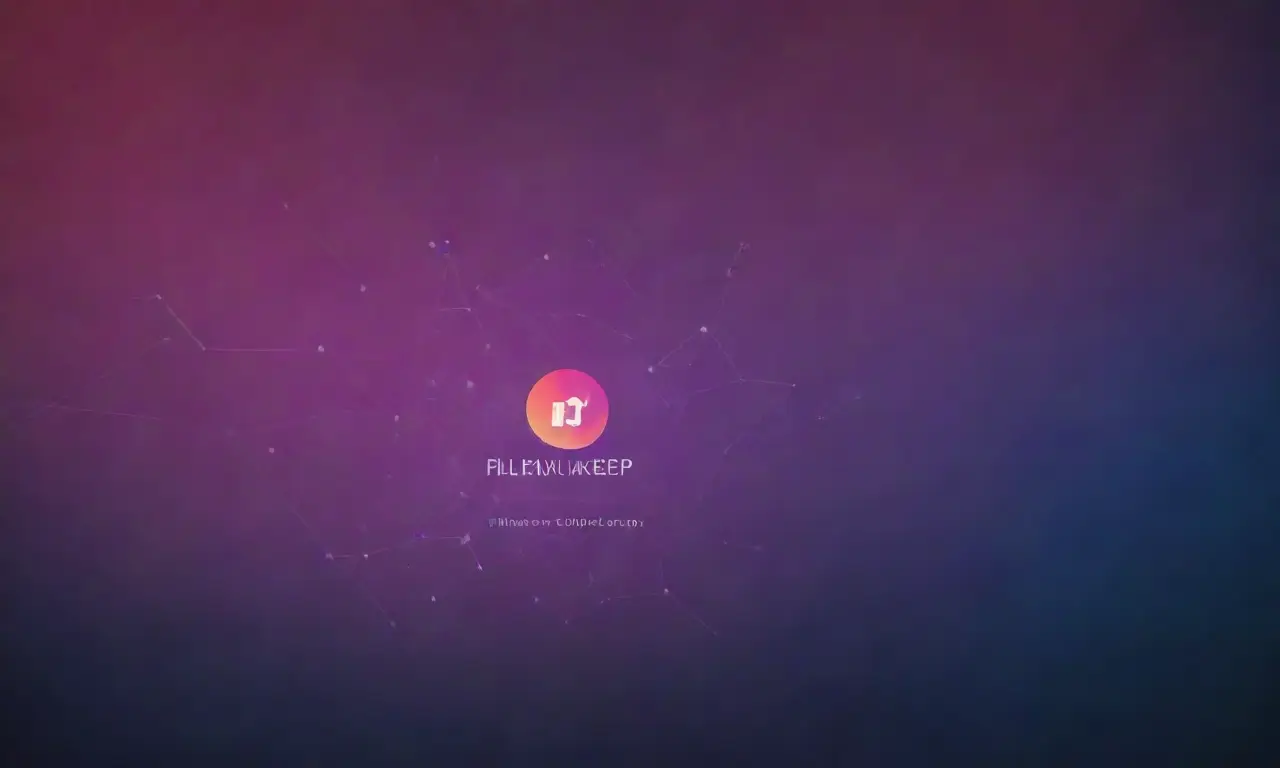
While the advantages of personalized marketing using machine learning are substantial, several challenges can arise during implementation. Understanding these obstacles is crucial for brands looking to optimize their marketing efforts.
Data Privacy Concerns
As brands gather vast amounts of data to fuel personalized marketing efforts, concerns regarding data privacy and consumer trust come to the forefront. Customers are increasingly aware of data collection practices, and any perceived misuse can lead to a loss of trust. Marketers must navigate regulations such as the General Data Protection Regulation (GDPR) or California Consumer Privacy Act (CCPA), which dictate how personal data can be collected, stored, and used.
To combat these concerns, brands must prioritize transparency in their data practices. Clear communication regarding what data is collected, how it is used, and the benefits to the customer is essential. By giving consumers control over their data and allowing them to opt in or out of data collection, brands can foster trust and build lasting relationships with their customers.
Algorithm Bias
Another significant challenge in the realm of machine learning is algorithm bias. Machine learning models can inadvertently perpetuate existing biases present in historical datasets. If a dataset reflects stereotypical behaviors or assumptions, the resulting predictions and recommendations will carry those biases, potentially alienating certain consumer groups or diminishing their experiences.
The Impact of Personalization on E-Commerce Success StoriesTo mitigate algorithm bias, brands need to ensure that their datasets are diverse and representative. Regular audits of ML models can help identify and correct biases, leading to more equitable personalization strategies. This not only leads to improved customer satisfaction but also enhances a brand’s reputation for fair practices.
Integration and Resource Allocation
Finally, the integration of machine learning into existing marketing strategies can be complex. Organizations need to ensure that they have the appropriate technology infrastructure, skilled personnel, and budgets to implement ML effectively. The integration process often requires significant investment in tools and training, which can be intimidating for businesses.
Brands should consider a phased approach to integration, starting with smaller projects or pilot programs to test the efficacy of machine learning-driven personalized marketing. Successful case studies can validate the investment required while building momentum for broader implementation. Additionally, allocating resources toward training employees about machine learning and data analysis is vital to ensure that teams can fully utilize the technology available.
Conclusion
As consumer expectations continue to evolve, the need for effective, personalized marketing strategies powered by machine learning is undeniable. Brands that successfully implement personalized marketing strategies utilizing ML can foster greater customer engagement, loyalty, and satisfaction. By employing techniques such as customer segmentation, predictive analytics, and real-time personalization, companies can provide tailored experiences that resonate deeply with their audiences.
However, it is essential to navigate the challenges that accompany this newfound power. Prioritizing data privacy, addressing algorithm biases, and securing the necessary resources for successful implementation are crucial steps in achieving personalized marketing success. By embracing these strategies responsibly, brands can build stronger connections with their customers, enhancing their overall marketing efforts.
In the end, as technology continues to advance and reshape the marketing landscape, those who adapt and innovate will thrive. By honing in on personalization through machine learning, businesses position themselves at the forefront of customer engagement, ensuring sustainable growth in an ever-competitive market.
If you want to read more articles similar to Personalized Marketing: Effective Strategies Utilizing Machine Learning, you can visit the Personalization Algorithms category.
You Must Read