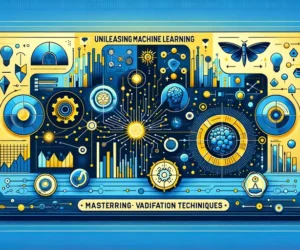
Real Estate Development: Machine Learning Insights for Predictive Analysis
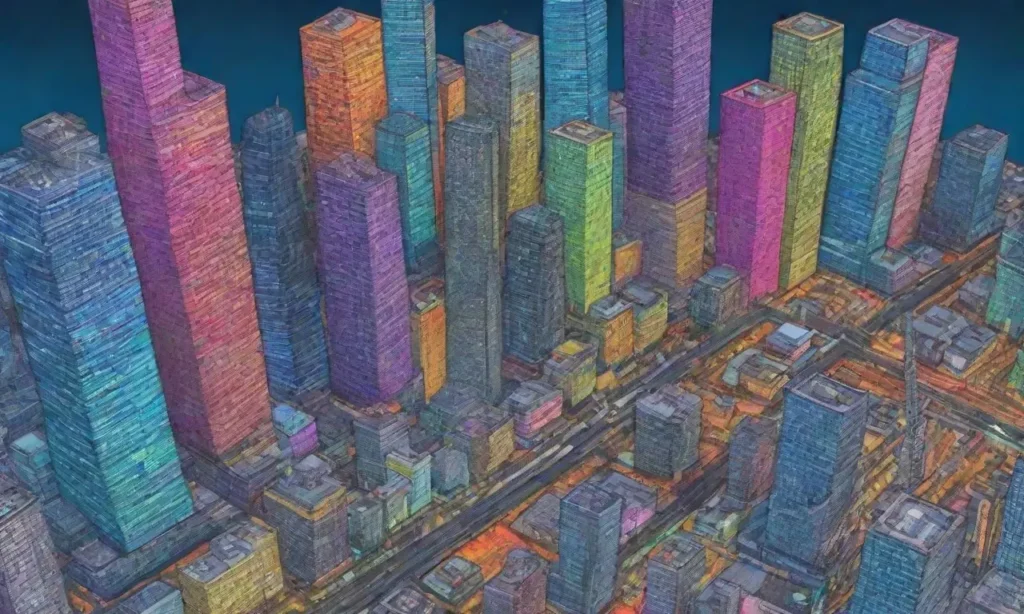
Introduction
In recent years, the real estate development industry has experienced radical transformations due to technological advancements, particularly in machine learning. As a field that often relies on large datasets to forecast market trends and property values, real estate developers are increasingly turning to machine learning for insights that can drive more informed decision-making processes. This juxtaposition of data science and real estate creates a synergetic relationship, leading to more efficient practices and heightened profitability.
This article aims to provide a comprehensive understanding of how machine learning can be leveraged for predictive analysis in real estate development. We'll delve into various machine learning techniques, the types of data commonly utilized, the potential benefits and challenges associated with implementing such technologies, and case studies that showcase the transformative impact of predictive analytics on the industry.
Understanding Machine Learning in Real Estate Development
Machine learning is a branch of artificial intelligence (AI) that enables systems to learn from data, identify patterns, and make decisions with minimal human intervention. In the context of real estate, machine learning algorithms can analyze historical data and recognize trends that might indicate future market conditions. For developers, insights generated from these analyses can be pivotal in determining the types of properties to invest in, appropriate pricing strategies, and even location-specific market predictions.
To utilize machine learning effectively, developers must gather relevant data. This data often encompasses historical sales records, property characteristics, economic indicators, demographic statistics, and geographical information. With the vast amounts of data available today, machine learning models can become extraordinarily accurate in predicting market behavior. These insights can empower stakeholders to make decisions based on data rather than intuition alone, minimizing risk and optimizing growth opportunities.
Realizing Smart Growth: Best Practices for Implementing ML StrategiesAmong the popular machine learning algorithms employed in real estate, we find techniques such as regression analysis, decision trees, and neural networks. Each of these approaches offers distinct advantages in terms of data handling and predictive accuracy. A thorough understanding of these algorithms can be instrumental for developers aiming to leverage technology to their benefit.
Data Types and Sources for Predictive Analysis
The effectiveness of machine learning in real estate development largely hinges on the quality and breadth of data utilized in predictive modeling. There are various types of data that stakeholders can explore. Here are some essential categories:
Historical Sales Data
One of the foundational data sources for predictive analysis in real estate consists of historical sales data. This data includes details such as previous sale prices, time on the market, and property specifications (e.g., number of bedrooms, square footage, and amenities). Analyses of this dataset empower developers and real estate investors to identify patterns and trends that can indicate future price movements. Furthermore, historical data can inform more granular analyses, such as seasonal price fluctuations and the impact of economic conditions on real estate values.
Economic and Demographic Indicators
Economic conditions play a crucial role in the health of the real estate market. Factors like interest rates, job growth, and overall economic development significantly influence buyer behavior. Therefore, incorporating economic indicators into machine learning models allows developers to gain a comprehensive understanding of market dynamics. Similarly, demographic data — such as population growth, income levels, and age distribution — provides key insights into demand for different property types. This multifaceted approach acknowledges that the real estate market does not exist in a vacuum; instead, it intertwines with broader economic and social trends.
Geospatial Analysis with Machine Learning: Urban Case StudiesGeographic and Environmental Factors
Geographic data encompasses details like location, proximity to amenities, and environmental attributes of neighborhoods (such as parks or schools). The integration of geographic data through location intelligence tools can facilitate the identification of burgeoning markets and areas ripe for development. Additionally, incorporating environmental factors such as flood zones or air quality into predictive models can enable developers to evaluate risk and sustainability, ultimately informing better investment decisions.
The Benefits of Machine Learning in Real Estate Development
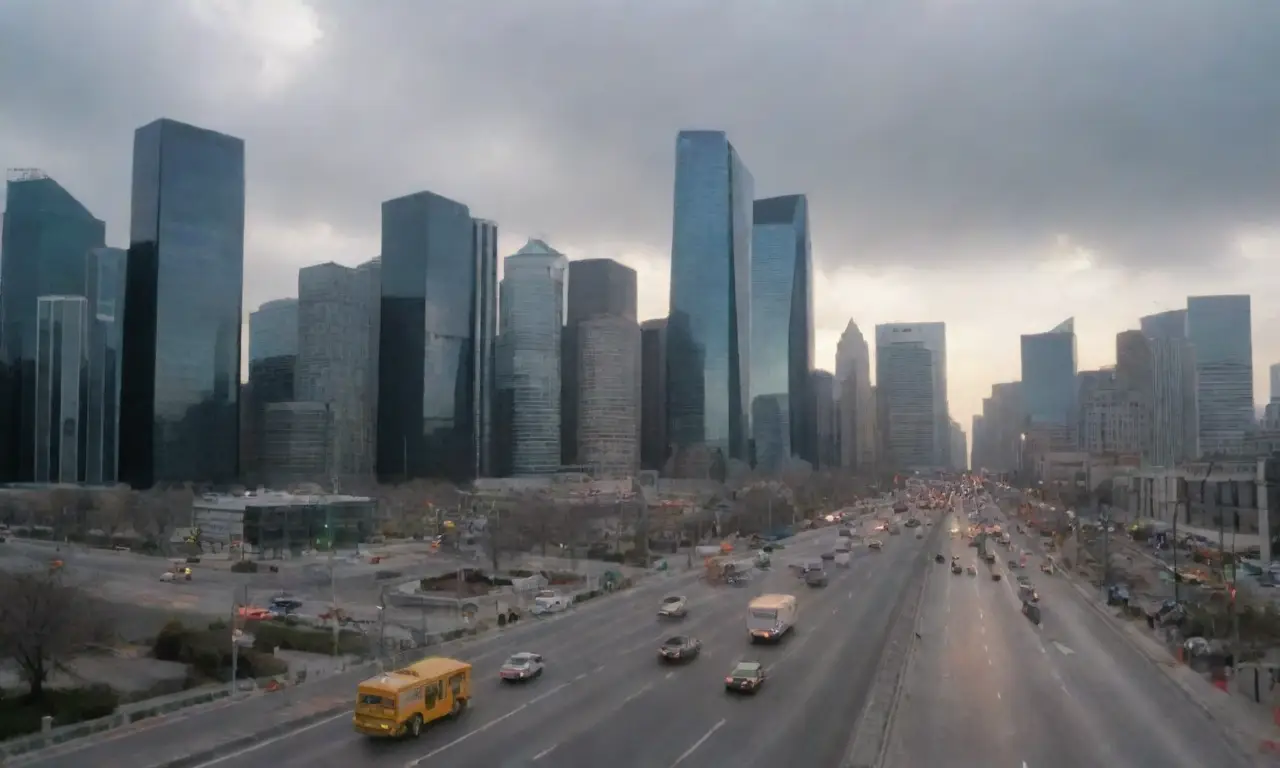
Integrating machine learning with real estate development brings forth numerous benefits that can significantly enhance decision-making processes and overall market success.
Improved Predictive Accuracy
Machine learning models, thanks to their ability to analyze vast datasets and uncover hidden patterns, often produce more accurate predictions than traditional analytical methods. As developers engage with sophisticated algorithms, they can fine-tune their models through continuous learning and adaptation. This leads to more precise forecasts regarding property appreciation, rental income potential, and occupancy rates. Such accuracy fosters a level of confidence in decision-making that is unattainable with intuition-based approaches alone.
Data-Driven Restore Urban Spaces: Machine Learning Success StoriesRisk Reduction and Resource Allocation
By utilizing predictive analytics, real estate developers can better understand potential market risks and allocate resources more efficiently. For example, machine learning can help assess the likelihood that a specific development project will yield a profitable return on investment. By analyzing historical data, developers can obtain clarifying insights on which property features yield the highest returns, enabling better budgeting and resource management. This capacity to minimize risk is invaluable, particularly in a market characterized by volatility and uncertainty.
Enhanced Marketing Strategies
Machine learning also offers the potential to refine marketing strategies through the analysis of consumer behavior. By studying patterns in buyer demographics and preferences, developers can tailor their marketing efforts more effectively. For instance, machine learning models can analyze data regarding property views and inquiries to identify target populations, which can lead to more personalized marketing campaigns. This results in increased engagement and, ultimately, accelerated sales cycles.
Challenges in Implementing Machine Learning
While the benefits of machine learning in real estate development are manifold, various challenges must be addressed for smooth implementation. Understanding these challenges is crucial for developers considering adopting machine learning-based solutions.
Data Quality and Variability
The success of any machine learning endeavor hinges on the quality of input data. In the real estate sector, data can often be fragmented, outdated, or inconsistent. To build accurate predictive models, developers must ensure that the data they utilize is reliable, which may necessitate the integration of data from multiple sources. Moreover, variability in data formats and standards can pose additional complications in data processing.
Predictive Maintenance for Urban Infrastructure: The Role of MLTechnical Knowledge and Expertise
Implementing machine learning involves a learning curve, and understanding how to build and maintain models requires specialized knowledge. Developers who may excel in project management and market analysis may find it difficult to adapt to the technical aspects of machine learning. This gap highlights the necessity of collaboration between real estate professionals and data scientists or IT specialists to bridge any knowledge divide.
Resistance to Change
Real estate development has long relied on established practices and intuition-driven approaches. As with any industry undergoing transformation, there may be resistance to adopting new technologies. Some professionals may hesitate to rely on data-driven insights, fearing it could undermine their experience-based judgment. Addressing this cultural resistance requires clear communication of the benefits that machine learning can bring, alongside strategic education on how it can complement — rather than replace — human expertise.
Case Studies of Successful Machine Learning Integration
To further illustrate the transformative impact of machine learning in real estate development, it is essential to examine several notable case studies that showcase successful integration.
Zillow's Zestimate
The real estate listing platform Zillow has become synonymous with data-driven pricing through its Zestimate feature. This tool leverages machine learning algorithms to calculate property values based on various factors, including historical sales, square footage, and local market trends. Zillow's ability to provide updated property valuations in near real-time demonstrates how machine learning can significantly enhance the traditional approach to property appraisal. This level of transparency and accuracy boosts consumer confidence and empowers buyers and sellers alike.
Urban Land Use Predictions: Leveraging Machine Learning ApproachesCompass's Predictive Analytics
Real estate technology company Compass utilizes machine learning techniques to help agents understand local markets better. Their platform employs predictive analytics to offer insights into buyer demand and neighborhood trends. By analyzing these trends, agents can dynamically adjust their marketing strategies and tools in real-time, ensuring that they remain competitive in rapidly evolving market landscapes. This adaptation is critical for staying ahead of the curve and addressing client needs more effectively.
Redfin and Pricing Optimization
Redfin, a technology-driven real estate brokerage, has implemented machine learning to enhance property pricing optimization. By analyzing over 100 variables related to both the property itself and the local market conditions, the platform can produce predictive pricing models that maximize sales potential. This innovation has allowed Redfin to achieve faster sales and improved customer satisfaction, reinforcing how predictive analytics can manifest tangible results in real estate transactions.
Conclusion
In summary, the intersection of real estate development and machine learning opens up a wealth of opportunities for predictive analysis that can lead to more informed decision-making, improved risk assessment, and enhanced customer engagement. As developers embrace innovative technologies and adopt predictive analytics, they can expect to experience significant transformations in how projects are approached, from identifying burgeoning markets to enhancing marketing strategies.
However, to navigate the challenges of implementing machine learning — such as ensuring data quality, acquiring technical expertise, and overcoming resistance to change — real estate professionals must engage in open dialogues with data scientists and IT experts. The successful integration of machine learning into real estate development not only requires an understanding of the technology but also a commitment to merging data insights with industry experience.
As the real estate landscape continues to evolve, the future holds exciting possibilities for those willing to embrace this technological revolution. With the continuing refinement of machine learning algorithms and growing datasets, the potential for innovative and predictive analytics in real estate will only expand, paving the way for data-driven decisions that benefit developers, buyers, and the overall market alike.
If you want to read more articles similar to Real Estate Development: Machine Learning Insights for Predictive Analysis, you can visit the Urban Planning category.
You Must Read