
Realizing Smart Growth: Best Practices for Implementing ML Strategies
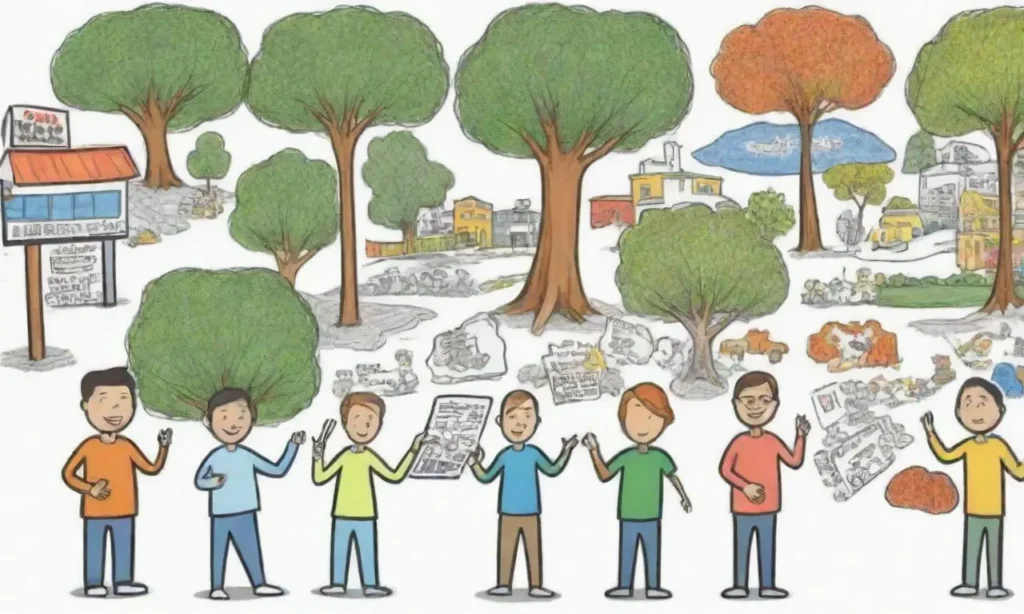
Introduction
In today's rapidly evolving technological landscape, Machine Learning (ML) has emerged as a transformative tool that holds the potential to propel businesses and organizations toward unprecedented levels of growth and efficiency. It enables data-driven decision-making, optimizes processes, and enhances customer experiences. However, realizing smart growth through ML requires more than just the application of sophisticated algorithms; it necessitates a strategic approach that aligns ML initiatives with the long-term goals of an organization. This is particularly important considering the pace of digital transformation and the increasing competition that organizations face across various sectors.
This article aims to explore effective best practices for implementing machine learning strategies that not only foster growth but do so in a sustainable and ethical manner. By delving into aspects such as data management, talent acquisition, stakeholder engagement, and the importance of ethical considerations in ML strategies, the article will provide a comprehensive guide for organizations looking to capitalize on the capabilities of machine learning while remaining conscientious of their broader impacts.
Understanding the Foundation of Machine Learning
The Role of Data in Machine Learning
At the core of any successful machine learning strategy is the effective management of data. Data is the foundation upon which ML algorithms are built, and its quality determines the efficacy of the outcomes produced. Organizations must prioritize data collection, storage, and processing techniques that ensure they have access to high-quality datasets. This involves establishing data governance frameworks that encompass policies for data access, usage, and protection, ensuring compliance with regulations such as the General Data Protection Regulation (GDPR) and the California Consumer Privacy Act (CCPA).
Moreover, data comes in various forms—structured and unstructured, internal and external. To fully leverage the capabilities of ML, organizations must be adept at integrating diverse data sources. This might include customer feedback, social media interactions, transactional records, and even sensor data for industries such as manufacturing or logistics. By creating a data lake that aggregates these various forms of data, organizations can enable more complex analyses and contribute to the development of innovative ML models.
In addition to data diversity, organizations should invest in analytics capabilities that allow for better data interpretation. Tools such as data visualization platforms can help stakeholders understand trends and insights drawn from ML outputs, supporting informed decision-making processes. Data scientists and analysts should work closely with business units to ensure that data-driven insights translate into actionable strategies.
Algorithm Selection and Model Development
Once high-quality data is available, the next crucial step is the selection and development of ML algorithms that align with business goals. Organizations must assess the various types of machine learning techniques—such as supervised, unsupervised, and reinforcement learning—and select models that best fit their use case. For instance, predictive models can be ideal for customer segmentation, while clustering models work well in anomaly detection tasks.
During the model development stage, it is vital that cross-functional teams collaborate to experiment with different algorithms and refining them based on performance metrics. Model evaluation is a critical step in this process, involving techniques such as cross-validation and the use of metrics like precision, recall, and the F1 score. By continuously monitoring these models after deployment, organizations can adapt and ensure that they maintain relevance and accuracy in a dynamic market.
Moreover, organizations should consider the computational resources required for ML model training and inference. Leveraging cloud computing platforms can provide scalable resources to manage large datasets and computational demands. This not only optimizes operational efficiency but also allows for flexibility when adjusting to varying workloads, an essential factor in fostering smart growth.
Talent Acquisition and Team Structure
To implement machine learning strategies effectively, organizations must recognize the importance of human capital. Hiring data scientists, ML engineers, and domain experts with the right skills is essential for the successful deployment of ML applications. Companies should foster a talent acquisition strategy that not only seeks technical proficiency but also emphasizes the candidate's ability to work collaboratively within interdisciplinary teams.
Creating a conducive environment for continuous learning and professional development is paramount. Organizations can facilitate this through training programs, workshops, and access to online courses that help upskill existing employees. By cultivating a culture of innovation and encouraging experimentation, organizations can position themselves to adapt to evolving technologies in the ML landscape.
Additionally, structuring teams to include diverse perspectives is critical. Incorporating members from varying backgrounds—such as business, technology, and social sciences—ensures that solutions are comprehensive and consider different stakeholder viewpoints. This multidisciplinary approach enriches discussions surrounding machine learning ethics, bias detection, and the societal implications of ML deployments.
Engaging Stakeholders and Driving Adoption
Stakeholder Engagement and Collaboration
Successfully implementing machine learning strategies requires effective engagement with both internal and external stakeholders. Involving stakeholders from the outset can foster a sense of ownership and facilitate smoother transitions throughout the adoption process. By communicating the value of machine learning initiatives clearly, organizations can build trust and understanding, which are essential for overcoming resistance to change.
Regularly engaging with relevant stakeholders—such as executives, IT departments, and front-line employees—can provide valuable insights that contribute to a more tailored approach in ML project deployment. This may include conducting focus groups, surveys, or workshops, which help gather feedback and refine ML solutions to better meet the specific needs of end-users.
In addition to internal stakeholders, organizations should also consider external collaboration with industry partners, academic institutions, and research organizations. These partnerships can lead to knowledge sharing, foster innovation, and access new datasets that enhance ML applications. Moreover, participating in industry forums and discussions can help organizations stay abreast of emerging trends and best practices while contributing to the collective understanding of responsible ML development.
Change Management and User Training
One of the most significant challenges organizations face during the implementation of machine learning strategies is ensuring user adoption. Even the most sophisticated ML models will fail to yield benefits if they are not embraced by users. As such, a clear change management strategy is crucial. This includes educating employees about the implications and benefits of ML solutions, addressing concerns about job displacement, and highlighting how these tools can augment their skills.
Investing in user training is a critical component of the adoption process. The training should not only cover the technical aspects of using ML-enabled tools but also emphasize how these technologies can enhance productivity and inform decision-making processes. Organizations may offer hands-on workshops, online tutorials, or mentorship programs that enable users to gain confidence in utilizing new systems.
Furthermore, ongoing support and feedback mechanisms should be instituted to ensure that users feel supported throughout their journey with ML tools. By creating an open line of communication for users to share their experiences, organizations can consistently gather valuable feedback and make adjustments that enhance usability and engagement.
Measuring Success and Iterating Strategies
To gauge the impact of implemented machine learning strategies, organizations must establish key performance indicators (KPIs) that align with defined objectives. These metrics could include improved efficiency, cost savings, increased customer satisfaction rates, and enhanced decision-making speed. Periodically assessing performance against these metrics enables organizations to evaluate their return on investment and make informed decisions regarding further investments in artificial intelligence.
Moreover, organizations should adopt an agile approach to iterate on their ML strategies continually. As new technologies emerge and market conditions change, strategies must evolve. By fostering a culture of experimentation, organizations can quickly pivot and adjust their ML initiatives based on real-time performance data and stakeholder feedback. This responsiveness enhances the organization's ability to realize not only short-term gains but also sustainable, long-term growth.
Conclusion
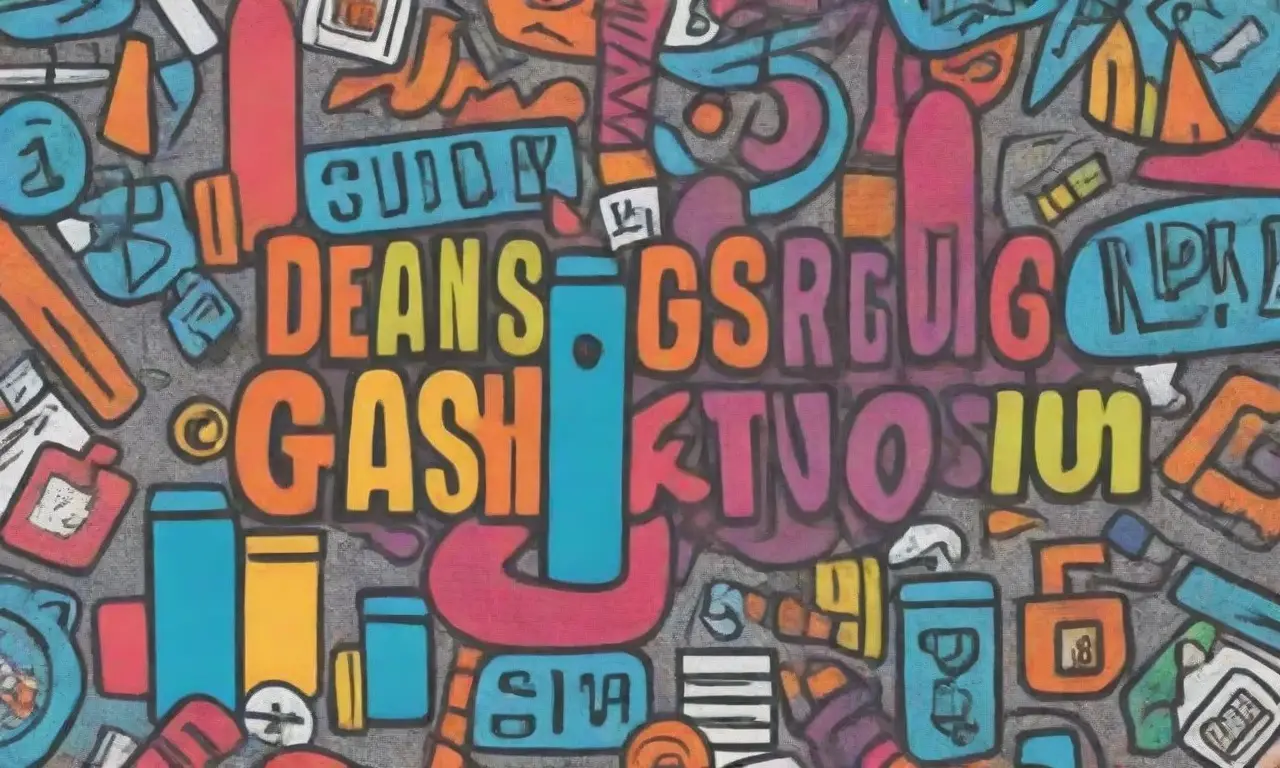
Realizing smart growth through the implementation of machine learning strategies is a multifaceted endeavor that requires thoughtful planning, execution, and continuous evaluation. From the foundational elements of data management and algorithm selection to engaging stakeholders and fostering user adoption, each step plays a crucial role in ensuring the successful deployment of machine learning initiatives.
By prioritizing ethical considerations and sustainability, organizations can contribute to a responsible and conscientious approach to technology that aligns with broader societal values. In an era of rapid digital transformation, embracing machine learning while adhering to best practices will not only drive efficiency and innovation but also foster trust and acceptance among stakeholders.
Ultimately, the journey of implementing machine learning strategies is an ongoing process that requires organizations to remain vigilant, agile, and committed to the principles of collaboration, transparency, and continuous learning. By cultivating a robust framework for ML integration, organizations are well-equipped to thrive amid the complexities of the modern business landscape and achieve sustainable growth for years to come.
If you want to read more articles similar to Realizing Smart Growth: Best Practices for Implementing ML Strategies, you can visit the Urban Planning category.
You Must Read