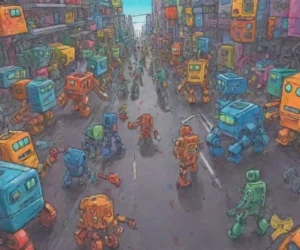
Risk and Return: Balancing Act with AI in Financial Forecasting
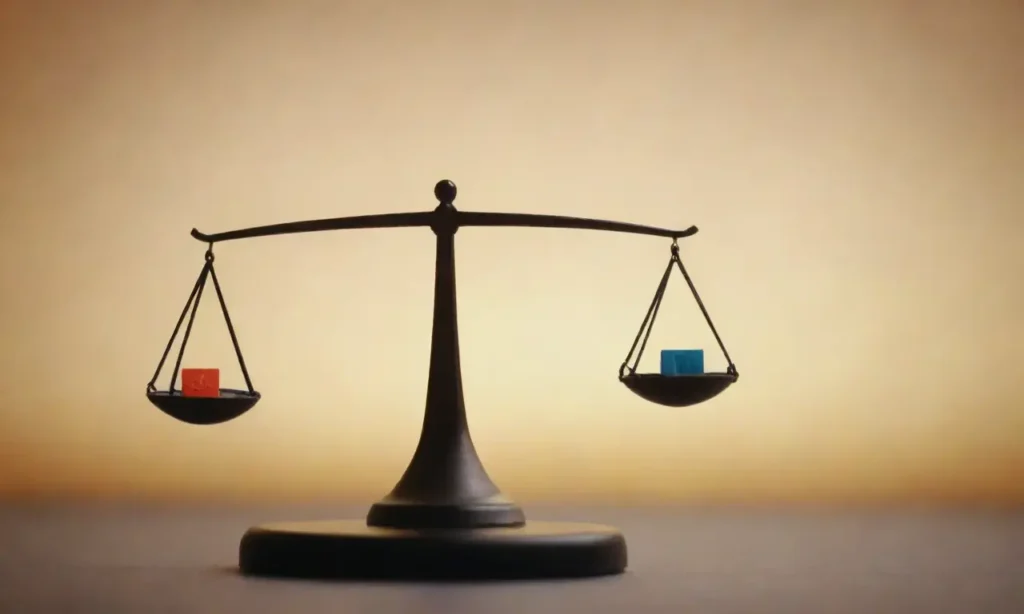
Introduction
In the realm of finance, the interplay between risk and return is a fundamental concept driving investment strategies and decision-making processes. Investors are constantly tasked with the challenge of maximizing returns while minimizing associated risks. This delicate balance, traditionally managed through various quantitative and qualitative approaches, is now increasingly being assisted by Artificial Intelligence (AI). The emergence of advanced AI tools has revolutionized financial forecasting, providing a more nuanced understanding of risk and return dynamics. This article delves into the complexities of risk and return in financial forecasting, examining how AI serves as a vital player in this balancing act.
In this article, we will explore the essential relationship between risk and return, how AI integrates with traditional financial forecasting methods, the new opportunities and challenges it presents, and some significant case studies showcasing its practical applications. By examining these various aspects, we aim to provide insights into how AI is shaping the future of financial forecasting and how investors can better equip themselves in understanding this transformative landscape.
Understanding Risk and Return
The relationship between risk and return is one of the foundational principles of investing. Risk refers to the uncertainty and potential for loss in an investment, while return is the financial gain derived from that investment over time. Investors inherently seek returns, but higher potential returns generally come with increased levels of risk. This trade-off creates what is often referred to as the risk-return spectrum, a framework used to analyze investment decisions.
The Concept of Risk
Risk can be categorized into various types, including market risk, credit risk, liquidity risk, and operational risk. Market risk is associated with the fluctuations of investment returns due to economic conditions, political events, or market sentiment. Credit risk pertains to the possibility of a borrower defaulting on a loan, while liquidity risk arises when an investor cannot execute a transaction at the desired price due to insufficient market activity. Finally, operational risk refers to losses resulting from inadequate internal processes or systems.
The Role of Bayesian Inference in Financial PredictionsUnderstanding these different types of risks is crucial for any investor. Risk assessment involves analyzing potential outcomes, determining the likelihood of their occurrence, and implementing strategies to mitigate those risks. Traditional approaches rely heavily on statistical methods and historical data; however, the dynamic and sometimes unpredictable nature of financial markets has made this increasingly challenging.
The Pursuit of Return
When investors talk about return, they are generally referring to the profit or income generated by an investment. This concept can be expressed in various forms, including capital gains, interest income, and dividends. For investors, the higher the expected return on an investment, the more likely they are to pursue it, given that it aligns with their risk tolerance.
To optimize returns, investors often employ different strategies, ranging from diversification—spreading investments across various assets to minimize risk—to more aggressive trading approaches, such as using leverage or options. These strategies can effectively increase potential returns but typically increase risk exposure at the same time. For instance, while stocks may offer higher long-term returns compared to bonds, they are also subject to greater volatility and potential losses.
The Risk-Return Trade-off
The risk-return trade-off reflects the fundamental balance that investors must strike. It is crucial for investors to understand their own risk tolerance and investment timeline, as these factors dramatically affect their approach to balancing risk and return. A younger investor with a longer investment horizon may be more inclined to take risks in pursuit of higher returns than a retiree who may prefer a more conservative investment strategy focused on preserving capital and generating income.
Advanced Techniques for Predicting Currency Exchange RatesThe challenge lies in accurately estimating both risks and expected returns. Traditional financial forecasting methods use historical data and statistical techniques, but these techniques have limitations. For example, they often struggle to account for sudden changes in market conditions, which can significantly affect risk and return profiles. This is where AI comes into play, offering innovative solutions to enhance forecasting capabilities.
The Role of AI in Financial Forecasting
AI technologies are increasingly infiltrating various sectors, and finance is no exception. AI-driven financial forecasting utilizes advanced algorithms and machine learning techniques to analyze vast amounts of data, identifying patterns and trends that human analysts may overlook. This not only improves accuracy but also enhances investment strategy formulation by integrating both qualitative and quantitative aspects of risk and return management.
Machine Learning Techniques
At the core of AI’s application in financial forecasting are machine learning techniques. These algorithms are designed to learn from data and improve over time without explicit programming. Classical machine learning methods such as regression analysis, decision trees, and neural networks are used to predict stock prices, evaluate credit risk, and even optimize asset allocation.
For instance, neural networks—a subset of machine learning—are particularly effective in recognizing complex patterns within large datasets. They can be trained to predict market movements by analyzing historical price data, trading volumes, and other relevant indicators. This allows for a more granular understanding of the dynamics at play and facilitates informed decision-making based on projected risk and return.
Practical Applications of Random Forests in Financial ForecastingBig Data Analytics
AI also leverages big data analytics, which refers to the process of examining large and varied datasets to uncover hidden patterns, correlations, and insights. The financial sector generates an overwhelming amount of data daily, from trade transactions and market news to social media sentiment and economic indicators. AI can process this information more quickly and accurately than traditional methods, leading to better-informed investment strategies.
For example, by analyzing customer feedback on trending financial products or evaluating sentiment from financial news outlets, AI can gauge public perception and sentiment towards specific investments. This ability helps in adjusting forecasting models to account for potential shifts in the market, making predictions more robust in the face of changing circumstances.
Enhancements in Risk Management
Beyond forecasting returns, AI enhances risk management processes. Financial institutions employ AI algorithms to monitor and assess risk exposure in real time. By integrating AI into risk management systems, organizations can identify potential risks faster and more effectively than using conventional risk assessment tools.
Using predictive models, AI can simulate various market scenarios, allowing risk managers to see how different factors influence potential outcomes. This foresight equips investors with the knowledge needed to make adjustments to their portfolios proactively, ensuring a more effective alignment of risk and return.
Using Genetic Algorithms for Stock Market Strategy DevelopmentOpportunities and Challenges in AI-Driven Forecasting
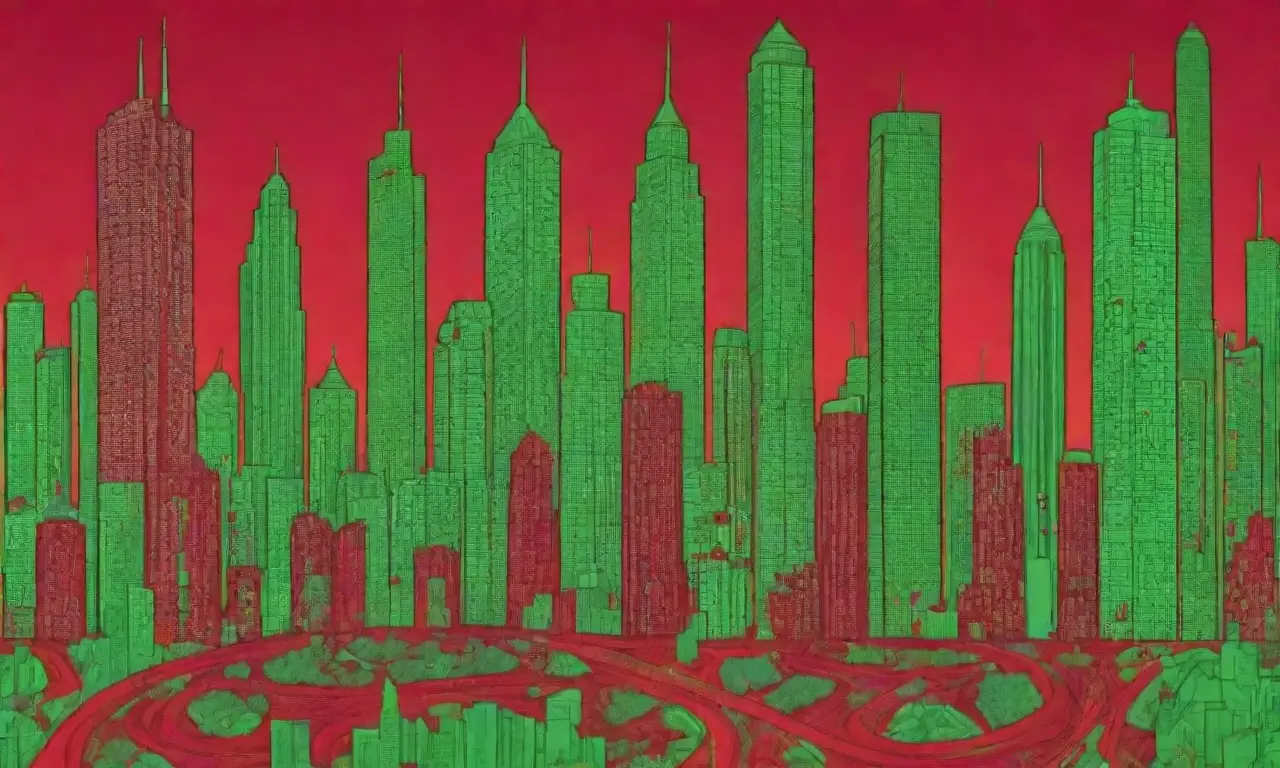
As with any technological advancement, the integration of AI into financial forecasting presents both opportunities and challenges. While AI enhances the level of precision and efficiency in predicting market movements, it also raises concerns regarding ethics, data privacy, and the potential for over-reliance on technology.
Opportunities for Enhanced Forecasting
The benefits of leveraging AI for financial forecasting are substantial. Enhanced accuracy in predicting market trends translates to better informed investment decisions, allowing investors to capitalize on opportunities with increased confidence. Moreover, AI facilitates real-time analysis, enabling firms to respond more swiftly to market changes, thus optimizing their risk-return profiles.
AI's data-driven insights foster the development of customized investment strategies, catering to diverse investor profiles and preferences. This newer level of personalization can lead to significantly improved customer satisfaction for financial services providers, who better meet their clients' unique needs.
Challenges in Implementation
However, implementing AI in financial forecasting is not without challenges. One significant issue is the data quality and availability. AI models rely heavily on historical data to predict future behavior; if this data is inaccurate or incomplete, it can lead to erroneous forecasting and misguided strategies. Data bias is also a concern, as training models on biased datasets can lead to flawed outputs, affecting investment performance and equity.
Furthermore, the complexity of AI models can create a black box phenomenon where understanding how models arrive at specific predictions becomes difficult. This lack of transparency can hinder trust among investors and stakeholders, particularly in a field where decisions come with substantial financial implications.
Ethical Considerations
AI's evolving role in finance also brings ethical considerations into focus. As decision-making processes become more automated, there is an increasing need for transparency and accountability to ensure that AI systems operate fairly and without unintended bias. Financial institutions must navigate regulatory requirements and ethical implications while balancing innovation and compliance in their AI implementations.
Conclusion
The dance between risk and return in financial forecasting will never be simple, but the integration of Artificial Intelligence is creating a paradigm shift in how investors and financial institutions approach this essential balance. AI empowers financial analysts and investors by providing a more granular view of market dynamics, enhancing both predictive accuracy and risk management capabilities.
The opportunities presented by AI in financial forecasting are significant, from improved investment strategies to real-time risk assessment. However, with these opportunities come challenges, such as the need for quality data, transparency in AI applications, and ethical considerations that must be carefully managed. As the financial landscape continues to evolve, it is crucial for investors to remain informed about these advancements and adapt their strategies accordingly.
In conclusion, embracing AI's capabilities while maintaining diligence in risk management and ethical considerations will enable investors to navigate the complex dance of risk and return effectively. By investing in knowledge and utilizing sophisticated tools, stakeholders can better position themselves for future successes in the ever-changing world of finance.
If you want to read more articles similar to Risk and Return: Balancing Act with AI in Financial Forecasting, you can visit the Financial Forecasting category.
You Must Read