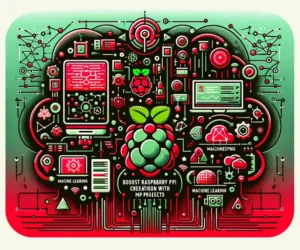
The Future of Text Classification: Trends and Predictions for 2024
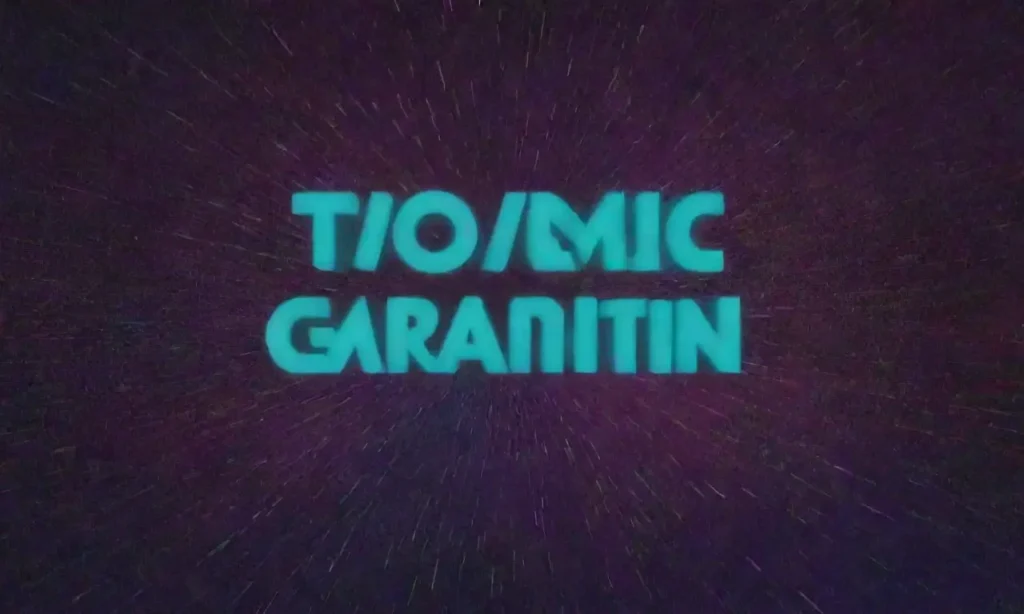
Introduction
Text classification is a critical area within the field of Natural Language Processing (NLP), which allows machines to analyze, understand, and categorize textual data. As we enter 2024, the landscape of text classification is evolving rapidly, driven by technological advancements, increased data availability, and the growing importance of real-time analytics. This article aims to explore the future of text classification, examining the emerging trends, the impact of advancements in artificial intelligence (AI) and machine learning (ML), and offering predictions that may shape the field in the coming year.
In this in-depth analysis, we will break down key trends anticipated for 2024, leveraging expert insights and data projections. We will discuss how the advancements in large-scale models, the integration of multimodal data, and the ethical implications of text classification technologies will redefine the boundaries of what is possible. By painting a comprehensive picture of the future landscape, this article serves as a guide for professionals, researchers, and enthusiasts who are keen to understand the nuances of text classification and its trajectory as we pave the way into 2024.
The Rise of Large Language Models
One of the most significant trends in text classification is the rise of large language models (LLMs). In recent years, models such as OpenAI's GPT-3 and Google's BERT have showcased remarkable abilities in understanding and processing language. As organizations increasingly recognize the undeniable benefits of implementing LLMs for text classification tasks, we can expect this trend to intensify in 2024.
Large language models are pre-trained on massive corpora of text, enabling them to grasp contextual relationships, idiomatic expressions, and subtleties of language. This leads to improved classification accuracy, allowing businesses to enhance their customer service strategies, sentiment analysis, and content categorization. The fine-tuning of LLMs on specialized datasets will allow organizations to create highly accurate classifiers to fit their unique needs, ensuring that even niche topics can be effectively analyzed and categorized.
The Evolution of Text Classification: From Rule-Based to AI-DrivenMoreover, the ongoing research within the NLP community is making strides in optimizing these large models for efficiency. By 2024, we expect to see techniques like distillation and quantization being implemented more widely, which would allow organizations to reduce the computational overhead associated with LLMs while maintaining high performance. This efficiency will democratize access, enabling smaller companies to implement sophisticated text classification systems that were previously restricted to well-funded tech giants.
Multimodal Data Integration
As artificial intelligence continues to evolve, the integration of multimodal data—the combination of text data with images, audio, and video—will emerge as another critical trend in text classification for 2024. Historically, text classification has primarily revolved around processing textual information in isolation. However, the future lies in understanding how different data types interact with each other to provide a more holistic perspective.
For example, consider social media posts, where text, images, and even short video clips all contribute to the overall context. Text classification systems that can analyze the interplay between these various modalities will be far superior in discerning the underlying sentiments, themes, and messages. Such integrated approaches will be invaluable across sectors such as marketing, healthcare, and finance, where contextual understanding can influence decision-making and strategy.
The development of advanced fusion techniques will be crucial in making sense of multimodal data in text classification. Techniques that combine deep learning architectures, such as Convolutional Neural Networks (CNNs) for images and transformers for text, will enable these systems to learn from richer representations rather than mere text patterns alone. As a result, organizations can unlock new dimensions in their text classification processes, leading to deeper insights and more accurate outcomes.
The Influence of Domain Knowledge in Text Classification SystemsEthical Considerations in Text Classification

With the growth of text classification technologies, ethical considerations will become increasingly pertinent in 2024. The power to classify and analyze massive amounts of text data raises concerns about bias, privacy, and misuse. Recognizing these ethical dilemmas is essential for organizations that leverage text classification to ensure they act responsibly and transparently.
One focus area will be the potential for algorithmic bias. If trained on data that reflects societal biases or discriminatory practices, classification systems risk perpetuating these issues, leading to unfair outcomes. Therefore, organizations must strive to create more representative training datasets and continuously evaluate their algorithms for bias. Fairness audits will become more commonplace, ensuring classifiers adhere to ethical standards and promote equitable treatment for all individuals.
Additionally, the issue of data privacy cannot be overlooked. As text classification systems become capable of extracting sensitive insights from user-generated content, there is an inherent risk of invading privacy. Striking a balance between leveraging valuable insights and maintaining individuals' rights will remain a critical challenge. Organizations will need to ensure compliance with regulations like the General Data Protection Regulation (GDPR) and establish clear protocols for user consent and data handling.
How to Implement Text Classification Using BERT and GPT-3User-Friendly Tools and Accessibility
In 2024, we anticipate significant advancements towards making text classification tools more user-friendly and accessible. In the past, deploying robust text classification models often required specialized technical knowledge, acting as a barrier to entry for many potential users. However, the trend towards democratizing AI tools is gaining momentum, as developers create intuitive, low-code or no-code interfaces that empower non-experts to harness the benefits of machine learning without in-depth programming expertise.
As textual data continues to proliferate across various platforms, the demand for easy-to-use classification tools will grow concurrently. These tools will allow users to quickly ingest texts, determine categories, and visualize results through simplified dashboards, fostering an environment where end users can take ownership of their text classification needs. Educational resources, online tutorials, and community-driven platforms will contribute to this shift by equipping users with the necessary knowledge and skills to navigate text classification efficiently.
Furthermore, initiatives to open-source text classification models and frameworks will likely accelerate in 2024. The rise of community-contributed models and pre-trained classifiers will help speed up deployment, allowing organizations to leverage cutting-edge models while avoiding the challenges associated with building them from scratch. As a consequence, smaller businesses and individual developers will be able to deploy high-performance text classification systems that would previously have been prohibitively expensive or technically complex.
Applications Across Industries
The implications of advancements in text classification will resonate across multiple industries in 2024. In healthcare, for instance, text classification will aid in streamlining patient feedback analysis, extracting valuable insights from unstructured clinical notes, and categorizing patient interactions for better decision-making. This will lead to improved patient care and operational efficiency within healthcare systems.
Practical Applications of Text Classification in Real-World ScenariosWithin finance, text classification will find applications in fraud detection, where systems will analyze transaction descriptions, customer communications, and news articles to recognize potentially fraudulent activities quickly. Automated alerts triggered by classifiers can help financial institutions stay ahead of fraudulent activities, ensuring a safer environment for users.
In the realm of marketing, businesses will increasingly rely on text classification to analyze customer feedback, social media sentiment, and online reviews. By identifying trends and customer opinions, brands can effectively gauge the public's perception, enabling them to modify strategies, enhance product offerings, or engage with their audience more effectively.
Conclusion
As we stand on the brink of 2024, the future of text classification is rife with potential and opportunity. The advancements in large language models, the integration of multimodal data, and a focus on ethical considerations will shape the direction of this crucial field. With significant strides anticipated in user-friendliness and accessibility, we can expect that text classification tools will empower a broader audience to harness the power of NLP.
It is essential for individuals and organizations to remain agile and adaptable as the landscape evolves. By keeping a close eye on these emerging trends and developments, stakeholders can better prepare themselves for the exciting innovations that lie ahead. As we approach the future, fostering collaboration across industries, prioritizing ethical practices, and embracing new technologies will be key to maximizing the benefits of text classification, ultimately driving progress across various sectors. In summary, 2024 promises to be a transformative year, heralding advancements that will redefine the way we approach text classification and its applications.
Enterprise Solutions for Scalable Text Classification Across OrganizationsIf you want to read more articles similar to The Future of Text Classification: Trends and Predictions for 2024, you can visit the Text Classification category.
You Must Read