
The Interplay Between Machine Learning and Ecological Risk Assessment
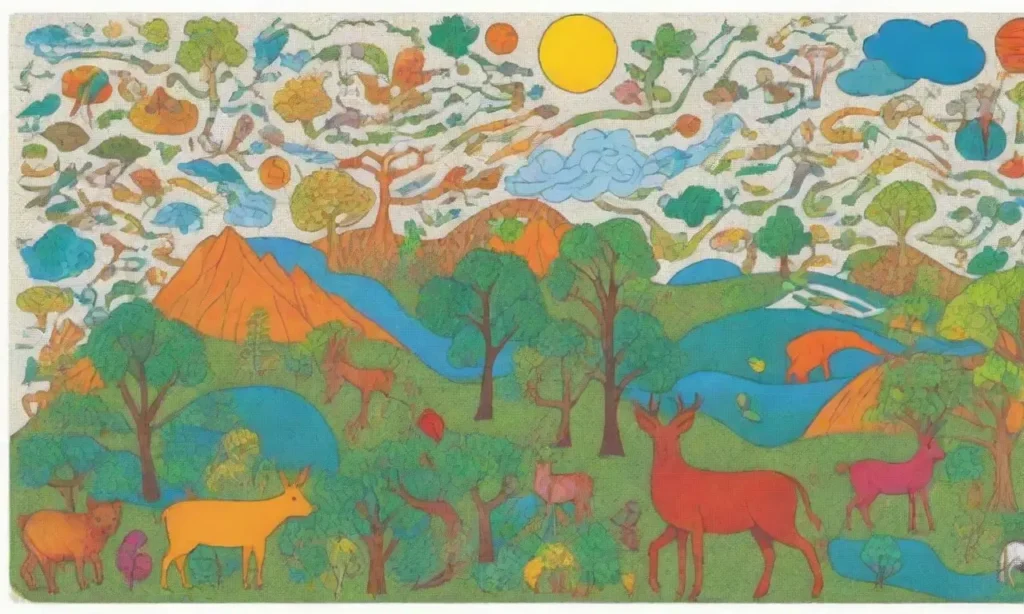
Introduction
In recent years, the integration of machine learning (ML) into various fields has sparked transformative changes, significantly enhancing our ability to process complex data and derive actionable insights. One particularly influential area benefiting from these technological advancements is ecological risk assessment, a discipline dedicated to understanding and mitigating the impacts of environmental hazards on natural ecosystems. This intersection of ML and ecological assessments is not merely a trend; it heralds a new era of environmental management that promises to bolster the accuracy and efficiency of risk assessments.
This article aims to delve deep into the intricate relationship between machine learning and ecological risk assessment. By exploring the methodologies involved, the implications for biodiversity and conservation, as well as the challenges that come with their integration, we hope to provide a comprehensive understanding of how this modern synthesis is shaping our approach to environmental sustainability and risk management.
The Foundations of Ecological Risk Assessment
Ecological risk assessment is a systematic process designed to evaluate the potential adverse effects of environmental stressors on living organisms and their habitats. This methodology often focuses on various stressors, including pollutants, invasive species, and climate change, aiming to understand their potential impacts on ecosystems. Traditionally, risk assessments involve qualitative and quantitative evaluations, relying on established ecological principles and empirical data.
One core element of ecological risk assessment is the conceptual model, which serves as a roadmap to identify key ecological processes and potential risks associated with specific stressors. Conceptual models help in formulating hypotheses about the interactions between stressors and ecological components, guiding the collection and analysis of relevant data. As such, they form the basis for systematic investigations, allowing researchers to track pathways through which stressors impact ecosystems and to establish correlations between different variables.
Risk characterization typically follows the identification and analysis phases. This involves integrating the collected data to assess the likelihood of an adverse effect occurring, identifying sensitive species or habitats, and estimating the potential consequences of specific stressors. The challenge arises when vast volumes of data – often complex and multidimensional – need to be processed and interpreted, which is where machine learning technologies become highly relevant.
Machine Learning: Tools and Techniques
Machine learning refers to a subset of artificial intelligence that allows computer systems to learn from data patterns without being explicitly programmed. The overarching goal is to enable machines to improve their performance over time as they are exposed to more data, ultimately leading to insights that can drive better decision-making in various domains, including ecological risk assessment.
Several machine learning techniques are particularly relevant to ecological studies. For instance, supervised learning algorithms—such as decision trees, support vector machines, and neural networks—can be employed to classify data into categories, helping ecologists discern patterns in ecological responses to different stressors. These algorithms use labeled data to learn relationships between inputs (e.g., pollutants, habitat characteristics) and outputs (e.g., species health, population dynamics), allowing researchers to make predictions about future ecological scenarios.
Another valuable method is unsupervised learning, which allows scientists to explore large datasets and discover hidden structures without pre-defined labels. Clustering algorithms, such as k-means clustering and hierarchical clustering, help ecologists identify groups of organisms sharing similar responses to environmental changes, while dimensionality reduction techniques like principal component analysis simplify complex datasets to reveal the most significant variables affecting ecological outcomes.
The potential of these ML approaches lies in their ability to process and analyze complex, high-dimensional datasets—including satellite imagery, environmental monitoring data, and biodiversity surveys—allowing for more robust and predictive ecological assessments.
Applications in Ecological Risk Assessment

The integration of machine learning in ecological risk assessment has already shown promising results across various applications. For instance, researchers are utilizing ML algorithms to predict species distributions and habitat suitability under different environmental scenarios, which is crucial for understanding the risks posed by territorial changes, climate change, and human activities. These predictions enable conservation planners to identify at-risk species and prioritize areas for protection or restoration efforts.
Ecotoxicology is another field where machine learning is revolutionizing risk assessment methodologies. By analyzing large ecotoxicity datasets, scientists can identify patterns of toxicity across different organisms due to specific chemicals or pollutants. ML techniques facilitate the development of predictive models that correlate exposure to various stressors with the probability of adverse ecological effects, thus aiding in regulatory decision-making processes regarding chemical safety and environmental protection.
Moreover, machine learning can enhance the understanding of habitat fragmentation and connectivity, which are critical components of ecological risk assessment. By analyzing spatial data combined with species movement patterns, predictive models can assess how landscape changes—such as urban development or deforestation—affect vital ecological networks. Such insights are essential for creating effective conservation strategies aimed at preserving biodiversity and ensuring ecosystem resilience against emerging threats.
Challenges and Limitations
While the integration of machine learning into ecological risk assessment delivers promising advantages, it is not without its challenges and limitations. One of the primary concerns lies in the quality and availability of data. ML algorithms require large datasets to learn effectively, yet ecological data is often sparse, inconsistent, or biased due to logistical constraints. Ensuring data quality and comprehensiveness is critical, as inadequate or misrepresentative data can lead to erroneous conclusions and misguided conservation strategies.
Additionally, there is a risk of overfitting—a situation where a machine learning model captures noise instead of the underlying data distribution. This can lead to models that perform well on training data but fail to generalize to new, unseen situations. Implementing robust validation techniques and ensuring transparency in model development are necessary steps to mitigate this risk. Furthermore, balancing model complexity with interpretability is essential, as ecologists need to understand the reasons behind specific predictions in order to apply them effectively in practical scenarios.
Lastly, there is the challenge of integrating machine learning findings into traditional ecological frameworks and making them accessible for stakeholders, including policymakers and conservation practitioners. To maximize the impact of such technologies, effective communication strategies that translate complex ML outcomes into actionable insights are necessary.
Conclusion
The intersection of machine learning and ecological risk assessment stands at the forefront of a transformative era in environmental studies. By harnessing data-driven insights and advanced computational techniques, ecologists can tackle complex environmental challenges with greater precision, ultimately leading to more effective risk assessments and improved conservation outcomes. The promise of these advancements rests on their ability to process vast amounts of ecological data, identify hidden patterns, and predict potential risks, thereby empowering stakeholders to make informed decisions.
Nonetheless, the journey is fraught with challenges that require careful navigation. Ensuring data quality, addressing the risk of overfitting, and facilitating effective communication between scientists and policymakers represent critical hurdles on this path. As interdisciplinary collaboration flourishes, integrating domain expertise with technological innovation, we can envision a future where ecological risk assessments become more accurate, efficient, and accessible than ever before.
Ultimately, as machine learning continues to evolve, so too will our capacity to understand and protect our ecosystems amid growing environmental pressures. The future of ecological risk assessment signals a promising horizon where data science and ecological integrity intersect, paving the way for resilient ecosystems that can withstand the pressures of environmental change and human activity. In this modern landscape, cultivating a harmonious relationship between technology and nature is not just essential; it is our responsibility as stewards of the planet.
If you want to read more articles similar to The Interplay Between Machine Learning and Ecological Risk Assessment, you can visit the Environmental Monitoring Tools category.
You Must Read