
The Role of Predictive Analytics in E-commerce Performance Boost
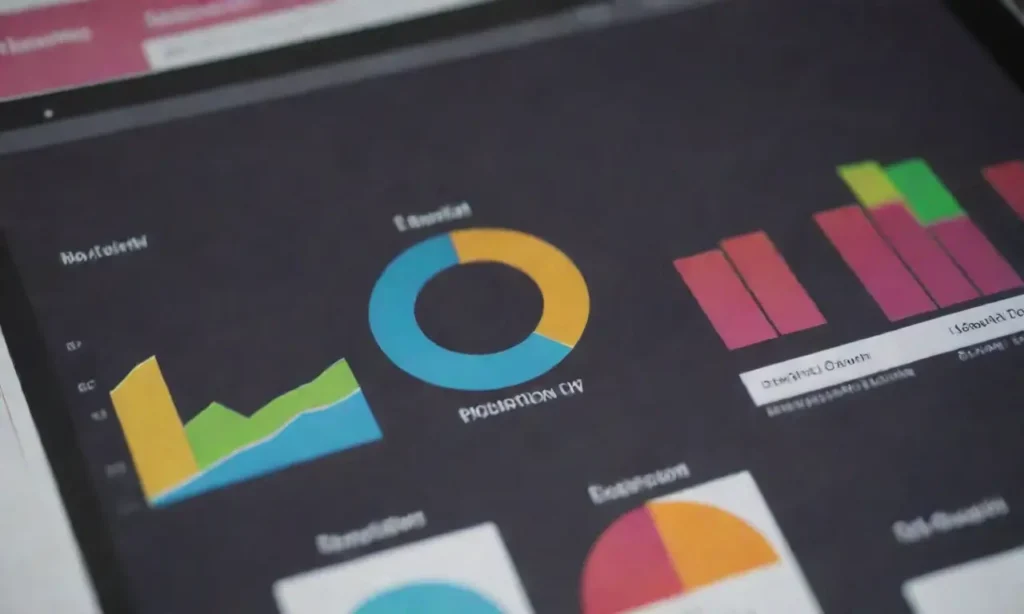
Introduction
In recent years, the e-commerce landscape has undergone significant transformation, largely driven by rapid advancements in technology and changing consumer behavior. Predictive analytics—the use of historical data, statistical algorithms, and machine learning techniques—has emerged as one of the most powerful tools that e-commerce businesses leverage to optimize performance, enhance customer experience, and drive profitability. Understanding how predictive analytics can impact e-commerce performance is essential for retailers seeking a competitive edge in a crowded marketplace.
This article delves into the role of predictive analytics in e-commerce, exploring its core concepts, applications, and benefits. We will discuss how e-commerce businesses can harness the power of data to gain actionable insights, improve forecasting, enhance decision-making, and ultimately boost their performance in the digital marketplace. By the end of this article, you'll develop a clearer understanding of predictive analytics' significance in e-commerce and how it can reshape business strategies for sustained growth.
Understanding Predictive Analytics
Predictive analytics combines various techniques from statistics, data mining, and machine learning to analyze current and historical facts to make predictions about future outcomes. This approach allows e-commerce businesses to sift through vast quantities of data from sales transactions, customer interactions, social media, and web traffic to identify patterns and trends. The goal is straightforward: forecast customer behavior and make informed business decisions based on those forecasts.
At its core, predictive analytics employs sophisticated algorithms that identify relationships among various data points. For example, an e-commerce store can analyze sales data alongside customer demographics and purchasing behaviors to predict future buying trends. As companies accumulate more data over time, their predictive models can refine and improve, providing insights that are increasingly accurate and relevant. The integration of artificial intelligence (AI) and machine learning further enhances the efficacy of predictive analytics, as these technologies continuously learn from new data.
The Role of Natural Language Processing in E-commerce PlatformsMoreover, predictive analytics is not limited to large organizations with vast data resources. Smaller e-commerce enterprises can also harness its power by using accessible tools and platforms that allow them to analyze data effectively, thus competing against larger players. The adaptability of predictive analytics makes it an invaluable resource for businesses of all sizes.
Key Applications of Predictive Analytics in E-commerce
Improving Customer Segmentation
One of the most significant applications of predictive analytics in e-commerce is improving customer segmentation. By analyzing past buying behaviors, businesses can identify distinct customer groups and tailor their marketing efforts accordingly. Predictive analytics goes beyond traditional demographic segmentation (age, gender, etc.) by incorporating behavioral data, such as customers' browsing history, purchase frequency, and response to promotional campaigns.
For instance, an e-commerce company can utilize predictive models to identify a segment of customers who are likely to purchase during the holiday season based on their previous shopping patterns. By targeting this group with tailored promotions and personalized offers, businesses can significantly enhance conversion rates. Additionally, predictive analytics can help identify potential churn risk among customers, allowing businesses to intervene with customized marketing communications to retain these clients before they disengage.
Moreover, predictive analytics can help identify new market opportunities by discerning emerging consumer trends. By aggregating data from various sources, e-commerce retailers can recognize shifts in customer preferences or detect new purchasing trends that may not yet be evident in traditional market analysis. For example, if a certain product category starts gaining traction in online forums or social media, predictive analytics can prompt businesses to capitalize on that trend before their competitors catch on.
Picking the Right Machine Learning Framework for Retail SolutionsOptimizing Inventory Management
In e-commerce, maintaining optimal inventory levels is crucial for avoiding overstocking or stockouts, both of which can result in lost sales and diminished customer satisfaction. Predictive analytics plays a pivotal role in optimizing inventory management by forecasting demand based on historical sales data, seasonal trends, and external factors such as economic indicators or major events.
For instance, an online clothing retailer can use predictive analytics to anticipate spikes in demand for specific items ahead of the summer season. By understanding purchasing patterns from previous years, the retailer can adjust inventory levels accordingly and ensure that popular items are in stock while minimizing excess inventory for less popular products.
Another critical aspect of inventory management benefited by predictive analytics is the ability to perform automated reordering. Based on forecasted demand, the analytics can signal when it is necessary to replenish stock, ensuring that businesses maintain sufficient inventory levels to meet customer demand without overspending on unused inventory. This data-driven approach minimizes inventory holding costs and increases operational efficiency, allowing e-commerce businesses to allocate resources more effectively.
Enhancing Customer Experience
Delivering an exceptional customer experience is paramount to achieving long-lasting success in e-commerce. Predictive analytics enables businesses to create personalized experiences for their customers, enhancing engagement and loyalty. By analyzing customer data, businesses can recommend products based on past purchases, preferred product categories, and browsing behavior.
How to Select the Right Machine Learning Tools for Retail NeedsFor example, when a customer visits an e-commerce site, predictive algorithms can assess their interests and recommend products that are likely to resonate with them. This personal touch not only increases the chances of conversion but also fosters a sense of connection between the customer and the brand, building loyalty over time.
Additionally, predictive analytics can be utilized to optimize marketing strategies by identifying the most effective channels and timing for reaching customers. By analyzing how customers engage with various marketing campaigns, e-commerce businesses can refine their communication strategies for better engagement. Email campaigns can be tailored based on customer preferences, while social media outreach can be directed toward segments of customers that are more likely to respond.
Furthermore, predictive analytics can enhance the overall customer journey by anticipating potential obstacles and proactively addressing customer concerns. For example, by predicting when customers are likely to abandon their shopping carts, retailers can implement targeted strategies to follow up with these customers—be it through reminders or personalized promotions—thereby reducing cart abandonment rates and boosting sales.
Conclusion
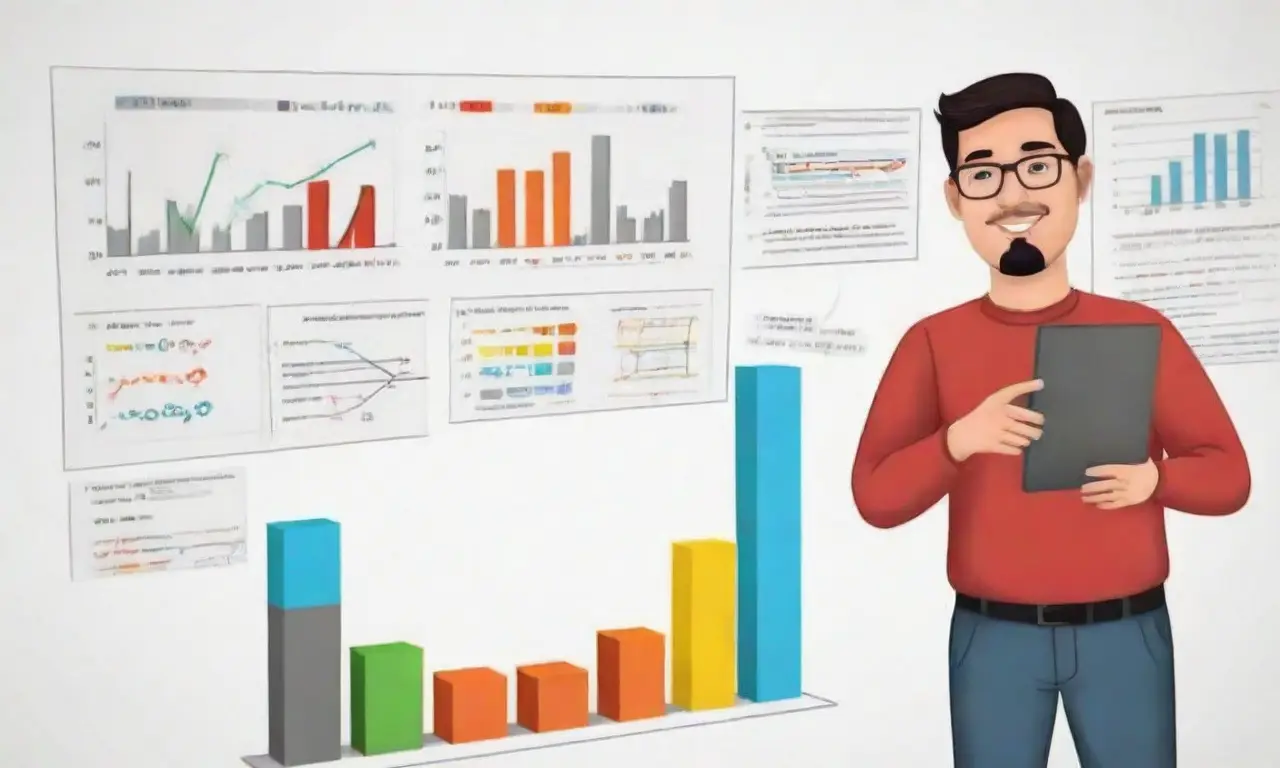
The implementation of predictive analytics within e-commerce has the potential to transform how businesses operate, interact with their customers, and make informed strategic decisions. By providing insights that drive improved customer segmentation, optimized inventory management, and personalized customer experiences, predictive analytics empowers e-commerce retailers to enhance their operational efficiency while maximizing growth opportunities.
As the e-commerce landscape continues to evolve, businesses that adopt predictive analytics are likely to remain at the forefront of industry trends and consumer expectations. The ability to harness data effectively not only helps retailers respond proactively to market changes but also strengthens their overall brand-stakeholder relationships.
In conclusion, investing in predictive analytics capabilities is a strategic move for e-commerce businesses seeking to optimize performance and gain a competitive edge. By embracing these data-driven insights and technological advancements, retailers can not only improve their current operations but also pave the way for sustained success in the future. As e-commerce becomes increasingly data-centric, those who prioritize predictive analytics will undoubtedly achieve superior performance in a rapidly evolving market.
If you want to read more articles similar to The Role of Predictive Analytics in E-commerce Performance Boost, you can visit the E-commerce Optimization category.
You Must Read