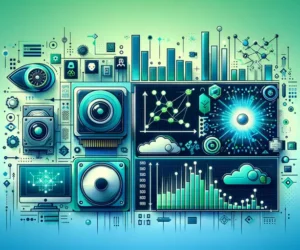
Understanding the Impact of ML on Automated Language Translation
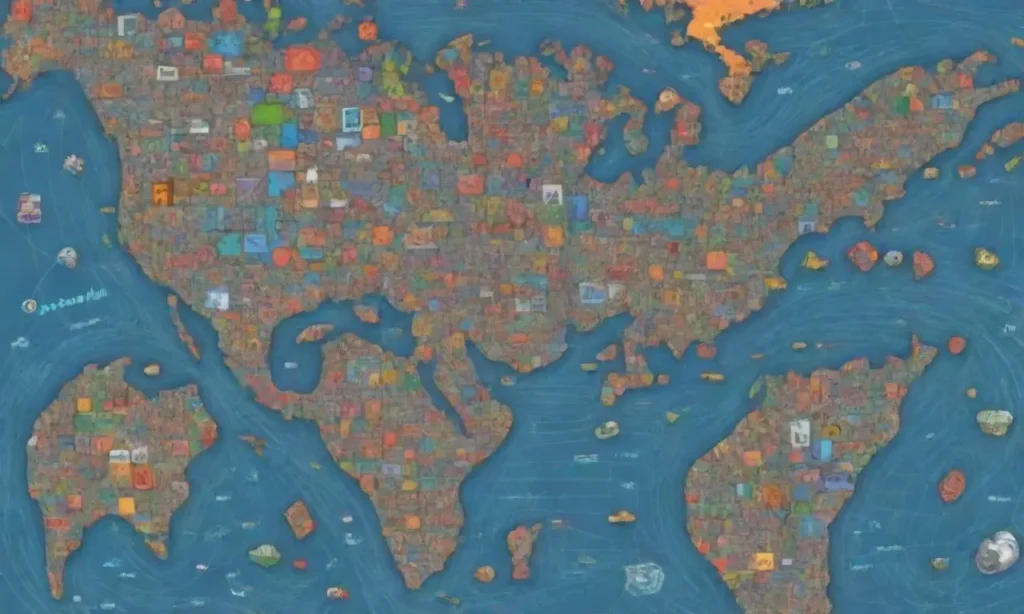
Introduction
In today's interconnected world, language translation plays a crucial role in facilitating communication across diverse cultures and languages. The evolution of machine learning (ML) has had a transformative impact on automated language translation, moving from rule-based systems to sophisticated neural networks that significantly improve translation accuracy and fluency. This article explores the underlying technologies, methodologies, and implications of ML on automated translation, highlighting its benefits, challenges, and future directions.
As we delve into this intriguing subject, we will cover various aspects of how machine learning enhances translation, including algorithmic processes, performance advancements, real-world applications, and potential ethical considerations. By understanding these facets, we can appreciate the strides made in language translation and their implications for global communication.
The Evolution of Language Translation Technologies
From Rule-Based to Statistical Methods
Traditionally, automated language translation relied on rule-based systems, which utilized dictionaries and a strict set of linguistic rules to translate text. While these systems laid the groundwork for automated translation, their limitations became evident as they struggled with idiomatic expressions, contextual nuances, and varying sentence structures. As a result, translations often lacked fluidity and coherence, making them challenging for users.
The introduction of statistical machine translation (SMT) in the early 2000s represented a significant leap forward. SMT relied on large corpuses of bilingual text, utilizing statistical models to predict the probability of a particular translation given a source sentence. This approach allowed for more flexibility and improved translation quality, especially with the emergence of parallel texts—documents translated into multiple languages. However, SMT still faced challenges concerning context and understanding subtleties.
Best Practices for Implementing ML in Language Translation ToolsNeural Networks and Deep Learning
The real revolution in automated language translation came with the advent of neural machine translation (NMT), powered by deep learning techniques. Rather than relying solely on statistical patterns, neural networks enabled the model to learn from data in a more human-like manner, understanding context and relationships between words and phrases. This shift allowed for end-to-end learning, where a single deep learning model could manage the entire translation process, from reading the source language to generating the target language output.
NMT systems, such as Google's Transformer model, use layers of neurons to process and refine translations, allowing for contextual awareness and better retention of meaning across sentences. As a result, translations generated by NMT are often more fluent and accurate than their SMT predecessors. Furthermore, innovations like attention mechanisms have further improved the ability of neural networks to focus on specific words or phrases, thereby enhancing the translation quality.
Real-World Applications of Machine Learning in Translation
The advances in ML-powered translation have not only improved the quality of individual translations but have also expanded the applications of these technologies. Today, automated language translation is ubiquitous, found in various platforms and tools that we encounter in our daily lives. From personal communication apps like WhatsApp to software platforms like Microsoft Translator, machine learning is at the core of enabling users to communicate effortlessly across language barriers.
In business contexts, NMT systems are used to facilitate communication across global teams, allowing for smoother collaboration and exchange of ideas regardless of employees’ native languages. Additionally, ML-driven translation services are increasingly utilized in customer support, enabling companies to provide assistance to international clientele in real-time.
Practical Applications of NLP in Language Translation AlgorithmsMachine learning also empowers the translation of content for websites and marketing materials, ensuring that brands resonate with audiences worldwide. By providing contextually relevant translations, companies can maintain a consistent voice and message while leveraging the unique connections and preferences of diverse markets.
The Advantages of Machine Learning in Language Translation
Enhanced Accuracy and Fluency
One of the most significant benefits of ML-based translation is the marked improvement in accuracy and fluency. As neural networks have evolved, translated content increasingly mirrors human-like understanding of language, reducing awkwardness and peculiar phrasing that often plagued earlier systems. This has empowered businesses and individuals to utilize automated translation tools without fearing miscommunication due to translation errors.
Moreover, the capability of NMT systems to adapt and learn over time enhances their performance. By feeding the models continuous streams of data—including user feedback and real-word applications—these systems grow increasingly sophisticated, continually refining their algorithms to produce better translations.
Contextual Understanding and Idiomatic Expressions
Another substantial advantage of ML is its ability to grasp the context of phrases and handle idiomatic expressions. Traditional translation systems often failed to understand the nuances associated with colloquial speech, leading to literal translations that could confuse users. However, ML-enabled systems can contextualize phrases based on surrounding sentences, enhancing their ability to interpret idioms or metaphors.
Understanding Machine Translation Evaluation Metrics in ML ModelsFor instance, consider the English phrase "kick the bucket," which means to pass away. A machine learning system trained on a diverse dataset would recognize this as an expression rather than a literal action and render it in a culturally appropriate equivalent in the target language, avoiding confusion and ensuring clarity.
Expanding Language Options and Accessibility
Machine learning has also democratized language translation, allowing for the inclusion of lesser-spoken languages previously overlooked by automated systems. Organizations such as Google Translate now offer translation services for hundreds of languages, making significant strides in addressing the needs of marginalized linguistic communities.
Furthermore, accessibility has improved tremendously; individuals without professional language expertise can leverage ML-powered tools to break down barriers and engage with content in various languages. This empowers cultural exchange, education, and global understanding, paving the way for a more interconnected world.
Challenges and Limitations of Machine Learning in Translation
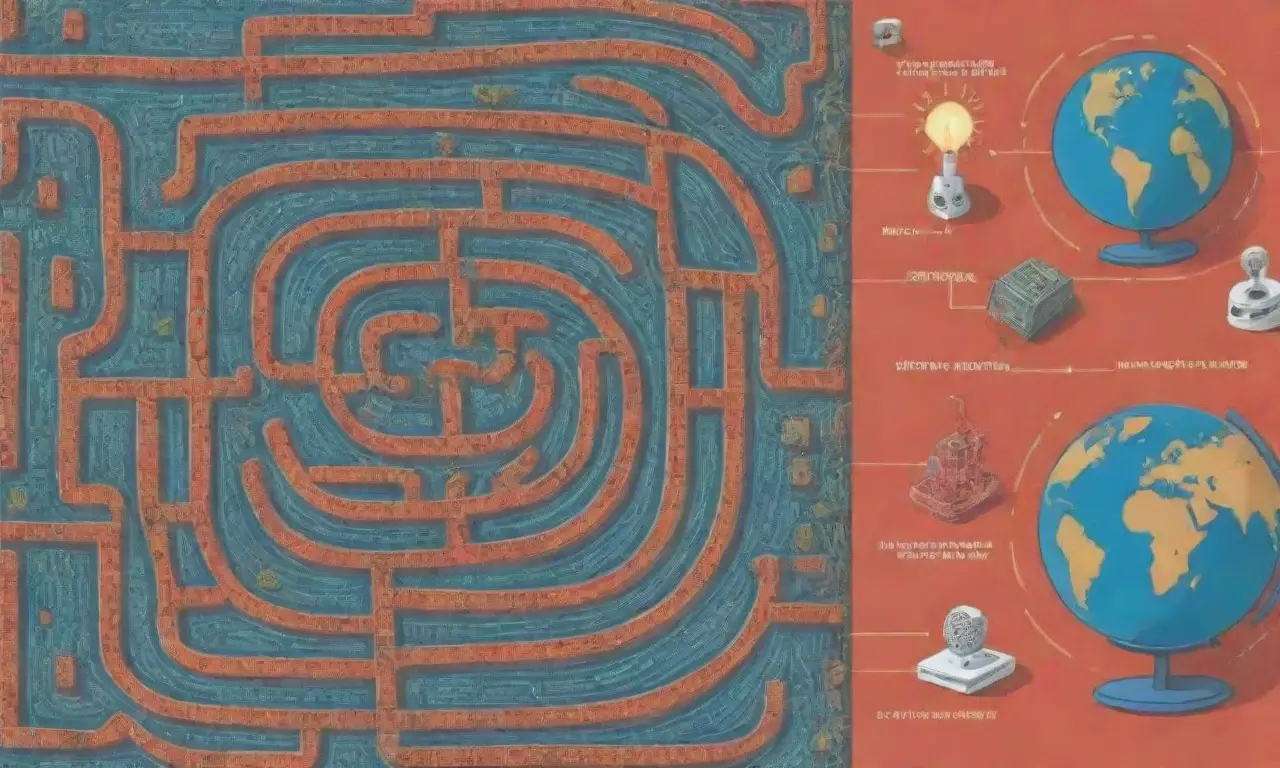
Lack of Cultural Sensitivity
While machine learning has made substantial strides in language translation, it still faces challenges regarding cultural sensitivity. Automated systems might misinterpret cultural nuances or context, leading to translations that lack consideration for the target audience's cultural values or norms. Failure to appropriately address these subtleties can result in misunderstandings, cultural faux pas, or even offense.
To mitigate this limitation, developers must ensure that their neural networks are trained on diverse datasets that reflect varied cultures and languages. Collaboration with native speakers and cultural experts may also help enhance the training processes, ensuring that the output is not only linguistically accurate but culturally appropriate.
Dependence on Quality Data
Machine learning models rely heavily on the volume and quality of data they are exposed to during the training process. If the training data is biased or lacks representation from diverse linguistic contexts, the resulting translations may reflect these biases. This dependence on data quality remains a significant barrier for languages with limited digital content, as these languages may not have enough parallel texts in the training datasets to train effective models.
Addressing this challenge requires the concerted effort of developers, linguists, and policymakers to promote the digitization of language resources and provide robust support for lesser-used languages as part of ongoing translation development.
Utilizing Machine Learning for Contextual Language Translation EnhancementsEthical Considerations and Privacy Issues
As ML-powered translation tools become more integrated into daily life, ethical concerns arise regarding data privacy and the potential misuse of these technologies. Translations often involve sensitive or confidential information, especially in business and healthcare contexts. It is crucial to ensure that user data remains secure and is not improperly leveraged to compromise individuals' privacy.
To address these ethical concerns, developers should adopt stringent data protection measures and transparency initiatives, informing users how their data is used and implementing safeguards to prevent unauthorized access.
Conclusion
The integration of machine learning into automated language translation has profoundly transformed the landscape of communication, facilitating connections across linguistic divides and fostering global collaboration. The shift from traditional rule-based systems to advanced neural networks has yielded profound enhancements in accuracy, fluency, and contextual understanding. As a result, language translation has become more accessible and reliable, empowering individuals and businesses to communicate effectively in a multilingual world.
Nevertheless, the journey is accompanied by challenges that must be navigated thoughtfully. Ensuring cultural sensitivity, addressing dependence on quality data, and grappling with ethical implications remain substantial hurdles that developers, linguists, and policymakers must overcome. By fostering inclusive and well-rounded approaches, stakeholders can maximize the potential of machine learning in translation while safeguarding the values of accuracy and respect for diversity.
Interpretable Machine Learning in Language Translation AlgorithmsIn summary, the future of language translation remains promising, with machine learning steering the way towards greater accuracy, efficiency, and global connectivity. As we continue to embrace these advancements, it is paramount to remain conscious of the intricate web of language and culture, ensuring that translation technologies evolve in ways that genuinely enhance communication and understanding across the globe.
If you want to read more articles similar to Understanding the Impact of ML on Automated Language Translation, you can visit the Language Translation Tools category.
You Must Read