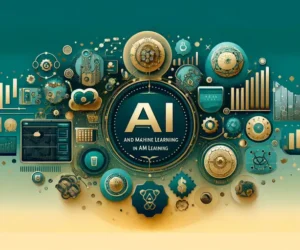
Unleashing Creativity: How Machine Learning Algorithms Transform Art

Introduction
The intersection of art and technology has long been a fertile ground for innovation, pushing the boundaries of human creativity and expression. In recent years, the emergence of machine learning algorithms has revolutionized the way we create, perceive, and engage with artistic works. From generative art to algorithmic music, machine learning has taken traditional forms of art and imbued them with a new life, offering safety nets for creatives while also challenging the definition of artistry itself.
This article delves deep into how machine learning is reshaping artistic practices across various mediums, illuminating the multifaceted relationship between humans and machines in the creative process. We'll explore the underlying technology, discuss notable examples of machine-generated art, and evaluate the implications of these advancements for the future of the artistic landscape.
Understanding Machine Learning Algorithms
At its core, machine learning refers to a subset of artificial intelligence (AI) focused on creating algorithms that enable computers to learn from and make predictions based on data. Unlike traditional programming, where developers provide explicit instructions, machine learning systems enable computers to adapt and improve over time without direct human intervention. They achieve this by analyzing large datasets to identify patterns and relationships.
Types of Machine Learning
There are three primary types of machine learning: supervised learning, unsupervised learning, and reinforcement learning.
From Pixels to Paints: The Evolution of AI in Art ProductionSupervised Learning involves training an algorithm on labeled data, which means that the algorithm learns from examples that have been tagged with the correct answer. For example, if we want to train an algorithm to recognize different styles of painting, we would feed it an array of artworks labeled by their styles. Over time, the algorithm learns to identify patterns specific to each style.
Unsupervised Learning operates differently; it works with data that has not been labeled. The algorithms attempt to identify inherent patterns without any prior knowledge of the output. This type is particularly prevalent in artistic realms where the goal may not be distinct categorization, but rather a deeper understanding of the data and the discovery of new trends or characteristics within the art.
Reinforcement Learning entails training an algorithm through trial and error, rewarding it for actions that bring it closer to a desired outcome. Think of it as teaching a dog new tricks by giving treats for good behavior. In art, this could relate to how an algorithm evolves based on feedback from the public or critics.
Applications in Art
Machine learning algorithms are being used creatively in various ways. Generative art is perhaps the most compelling application, where algorithms create new artwork based on learned patterns from existing works. Programs like DeepArt use neural networks to transform photographs into artwork that emulates famous artist styles, opening up new avenues for creative expression.
How Deep Learning is Revolutionizing the World of Artistic DesignAnother significant application is in music, where algorithms can compose, remix, and even produce entirely new tracks based on learned techniques from thousands of songs across genres. For instance, OpenAI’s MuseNet can generate musical pieces that blend different styles, offering musicians a unique partnership for innovation.
Case Studies in Machine-Generated Art
The "Next Rembrandt" Project
One astonishing example of machine learning in art creation is the "Next Rembrandt" project. This initiative aimed to produce a new painting in the style of Rembrandt van Rijn by utilizing historical data as its foundation. Researchers analyzed over 300 of Rembrandt's works, focusing on elements such as color palettes, brush strokes, and composition styles.
The data was fed into a machine learning algorithm, which was then programmed to understand the nuances of Rembrandt's artistic technique. The outcome? A unique piece of artwork that bore striking similarities to Rembrandt's original paintings, perfectly capturing the essence of the master’s style. This project not only showcased the capabilities of machine learning but also raised questions around originality and creativity—can a machine truly reproduce the emotional depth of human-created art?
AI-Generated Art Exhibitions
In recent years, numerous exhibitions have showcased AI-generated art, further solidifying its place in contemporary galleries. Christie's auction house made headlines by auctioning off an AI-created painting titled "Portrait of Edmond de Belamy," which was generated by a machine learning algorithm trained on historical portraits. This event marked a turning point in the art world as it sparked debates about ownership, authenticity, and the role of human artistry in a domain increasingly influenced by technology.
Diving into the World of AI: Enhancing Art with AlgorithmsOrganizations like Obvious Art have continued pushing the boundaries by creating collections entirely composed of AI creations. Such initiatives have led to increased recognition of AI as a legitimate artist, with works attracting high prices and garnering critical acclaim. This evolution prompts reexamination of what it means to create and who can be considered an artist in an age characterized by advancements in algorithmic creativity.
Music and Machine Learning
Machine learning doesn’t just thrive in the visual arts; it also permeates the world of music composition and production. Endlesss, for example, is a collaborative music platform that utilizes machine learning to help users create and remix tracks in real-time. By analyzing user input, the platform can suggest harmonies, rhythms, and melodies based on learned musical tendencies, effectively democratizing music creation and enabling musicians to explore sounds beyond their training.
Furthermore, artists like Holly Herndon have developed works using machine learning to generate vocal harmonies and complex sonic tapestries. Her innovative use of technology in creating music not only presents a modern take but also challenges traditional concepts of performance, composition, and collaboration.
Challenges and Limitations
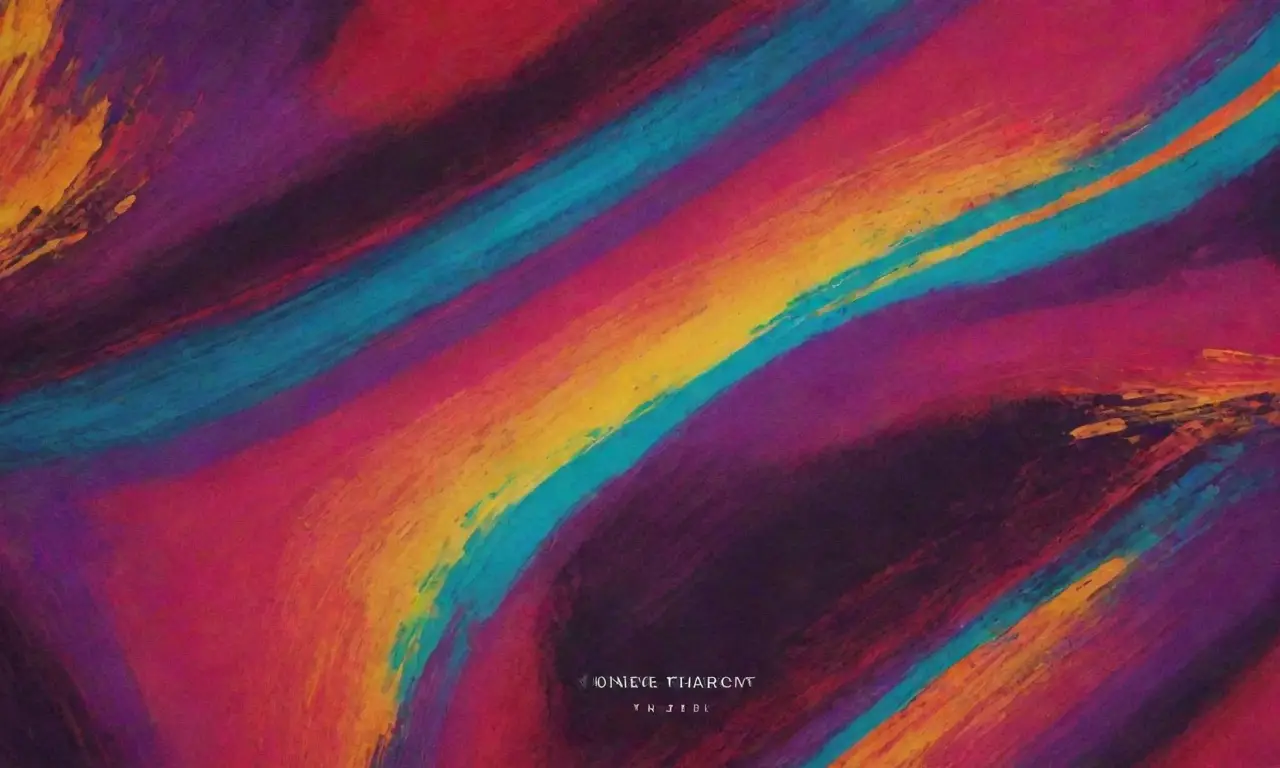
Despite the promising benefits of machine learning in art, several challenges remain. The ethical implications of machine-generated art have come to the forefront of discussions. Questions arise regarding authorship, as artists who leverage machine learning must navigate the murky waters of whether the value lies in the original algorithm’s designs or the artist's vision for the work.
Data Bias
Another notable concern is data bias. The quality of a machine learning algorithm heavily relies on the data it is trained on. If the dataset lacks diversity or reflects harmful stereotypes, the algorithm can inadvertently produce skewed or offensive content. This challenge is particularly relevant in visual art and design, where cultural representations can greatly influence public perception.
Emotional and Human Connection
Moreover, there is an ongoing debate about the emotional resonance of machine-generated art. While algorithms can produce aesthetically pleasing or technically skilled works, critics argue that they lack the emotional depth inherent in human artistry. Emotion, narrative, and context are often pivotal in art, and while algorithms can mimic styles, they do not possess genuine experiences or emotions to infuse into their creations.
Conclusion
The integration of machine learning algorithms into the realm of art is transformative, ushering in an era where creativity is shared between humans and machines. As we have seen, machine learning empowers artists by expanding their creative toolkits, fostering experimentation, and inspiring innovative forms of expression. Projects like the “Next Rembrandt” and auctioned AI-generated art challenge our understanding of authorship and originality, igniting spirited conversations around the future of creativity.
AI and the Democratization of Art: Opening New Avenues for CreatorsAs machine-generated works continue to find their place in galleries and on digital platforms, the implications for both artists and audiences are profound. We are forced to reconsider long-standing definitions of artistry, emotional engagement, and artistic merit. While these technologies offer exciting possibilities, it is essential to approach them with mindfulness, critically engaging with ethical considerations and the implications of automation in creative industries.
In summary, while the merger of machine learning and art presents us with thrilling opportunities, it also reminds us of the integral role of the human spirit in the creative process. The dialogue between artists and algorithms is just beginning, and as we navigate this brave new world, we must carefully decide how best to utilize these powerful tools while staying true to the essence of art—and humanity itself.
If you want to read more articles similar to Unleashing Creativity: How Machine Learning Algorithms Transform Art, you can visit the AI-Based Art Creation category.
You Must Read