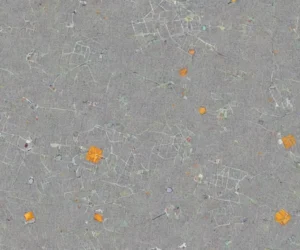
Utilizing Machine Learning for Contextual Language Translation Enhancements
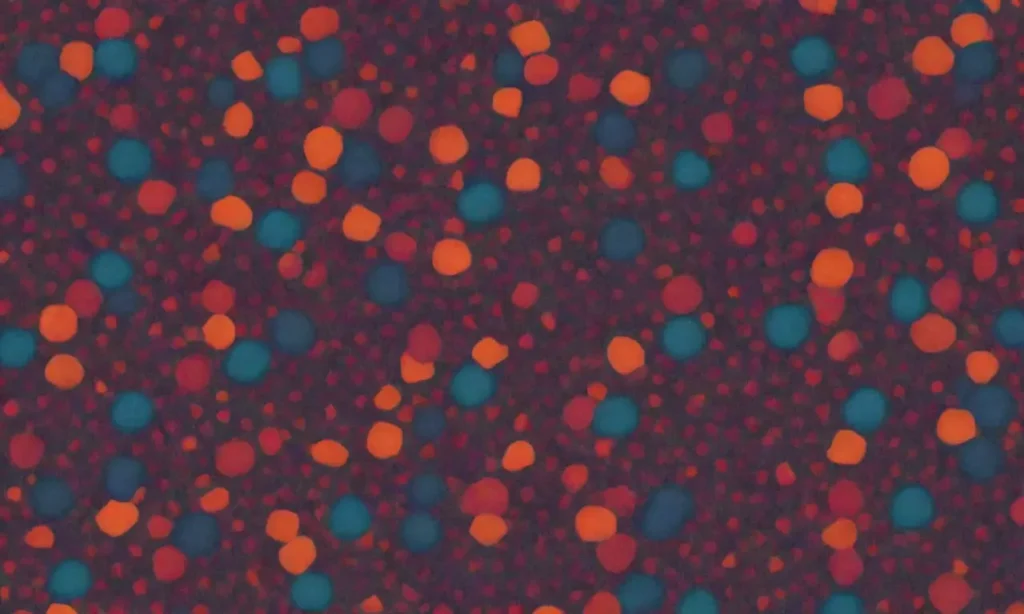
Introduction
In today's globalized world, language translation has become increasingly important as businesses, technologies, and cultures interconnect across borders. Traditional translation methods often fall short of providing nuanced understanding and context, leading to misinterpretations and ineffective communication. The advent of machine learning (ML) has opened new avenues for enhancing language translation, enabling more accurate and contextually relevant outputs, which are crucial in bridging linguistic gaps.
This article delves into how machine learning techniques are revolutionizing the field of language translation. We will explore various machine learning models, their roles in contextual translation, the challenges they address, and the implications for businesses and individuals. By understanding these advancements, readers will gain insight into the future of translation services and the ongoing integration of technology and linguistics.
The Role of Machine Learning in Language Translation
Machine learning has emerged as a powerful tool in the field of natural language processing (NLP), which is a critical component of language translation. Traditional rule-based translation methods often relied on a set of static rules that do not adapt to different contexts. In contrast, machine learning models learn from vast amounts of linguistic data, enabling them to recognize patterns and make informed decisions based on context. These models can analyze large datasets of bilingual texts, extracting meaning and relationships between words, phrases, and structures.
One of the most significant breakthroughs in machine learning for translation is the use of neural networks, particularly Recurrent Neural Networks (RNNs) and Transformer models. These frameworks enable the processing of texts not just word by word, but also by taking into account the surrounding context and overall structure of sentences. Unlike traditional methods, these networks can consider the entirety of a statement, allowing for more nuanced translation that captures the intent behind the words.
Interpretable Machine Learning in Language Translation AlgorithmsMoreover, transfer learning has proven incredibly effective in improving the performance of translation models. By pre-training on large corpuses of data in one language and then fine-tuning on a specialized dataset in another, models can leverage previously learned knowledge to enhance their translation accuracy. This approach has markedly improved the handling of low-resource languages, which typically lack extensive bilingual corpuses for training.
Enhancing Contextual Understanding through Deep Learning
Deep learning represents an advanced subset of machine learning that significantly boosts the performance of translation models. By employing multi-layer neural networks, deep learning models can automatically learn representations of data, allowing them to better understand the intricate relationships between different words and phrases in various contexts. This ability to extract abstract features from data enhances the model's performance in capturing contextual meanings that larger vocabularies or complex structures necessitate.
For instance, the Transformer architecture, introduced in the paper "Attention is All You Need" by Vaswani et al., has fundamentally transformed the field of machine translation. The key innovation here is the attention mechanism, which enables the model to weigh the importance of different words in a sentence relative to one another. This means that when processing an English sentence, the model can effectively consider relevant words in a translation to, say, Spanish, rather than treating each word in isolation. This capability significantly mitigates errors that arise from homonyms, idioms, or culturally specific references.
By using attention mechanisms, deep learning models can also better handle long-range dependencies in language, which are common in complex sentences. For instance, in sentences that contain multiple clauses, a model utilizing attention can refer back to important earlier components, improving overall coherence in the translation. This enhancement is particularly beneficial in cases where the meaning of a word can shift dramatically depending on its context within a sentence.
Understanding the Impact of ML on Automated Language TranslationChallenges in Contextual Language Translation

Despite the remarkable advancements machine learning has brought to language translation, various challenges remain. One significant hurdle is dealing with polysemy, which refers to a single word having multiple meanings based on the context. Machine learning models, if not properly trained or evaluated, may struggle to differentiate between these meanings, leading to inaccurate translations. For example, the word “bank” can refer to a financial institution or the side of a river, and failing to discern the intended meaning can create confusion for the reader.
Another challenge is the cultural context of language, which extends beyond simple word replacement. Idiomatic expressions, slang, and cultural references often do not translate directly from one language to another. This presents a dilemma for machine learning models that rely on literal translations. These subtleties necessitate a deeper understanding of cultural nuances and the purpose behind the words chosen. Many machine learning systems including those based on deep learning can falter when facing such culturally rich content without additional context from human input.
Furthermore, there’s the issue of data bias in training sets. If a translation model is trained predominantly on texts from a specific region or demographic, it may produce results that do not accurately represent the broader variation of a language. This can lead to translations that are skewed or missing critical localized meanings. Researchers and developers are consistently working to ensure that training datasets are as diverse and comprehensive as possible to minimize this bias and improve the model's adaptability in dynamic contexts.
Best Practices for Implementing ML in Language Translation ToolsFuture Insights & Innovations in Machine Translation
The future of machine learning in language translation seems poised for remarkable innovation. One notable trend is the integration of unsupervised learning techniques into translation systems. In contrast to traditional supervised learning, which requires large amounts of labeled data, unsupervised learning enables models to understand patterns and relationships in languages without explicit guidance on what the output should be. This approach can significantly reduce reliance on pricey and cumbersome bilingual corpuses while enhancing the model's flexibility.
Moreover, the incorporation of reinforcement learning could yield further improvements in translation accuracy. This technique involves training models through a feedback mechanism, where the system learns to correct itself based on the success or failure of its translations. By implementing such dynamic training strategies, models can evolve continuously, refining their capacity to translate with greater contextual awareness.
Furthermore, multimodal translation represents an exciting frontier. By synthesizing not just textual data but also visual, auditory, and contextual information, future models may grasp the full spectrum of human communication. For example, a model might utilize video or audio alongside written text to generate translations that consider tone, gestures, and visual representations, thereby enriching the understanding of the text.
Developments in explainable AI are also likely to influence machine translation. This refers to creating models that transparently explain their reasoning and translation processes, enhancing user trust and understanding. By revealing how translations arise from input data, developers can identify potential biases or errors, ensuring a higher standard of accountability and accuracy in translations.
Practical Applications of NLP in Language Translation AlgorithmsConclusion
The use of machine learning in language translation has dramatically transformed how we approach linguistic differences and cultural nuances. As models become increasingly sophisticated through deep learning, attention mechanisms, and innovations in training methods, the ability of these systems to produce accurate and context-sensitive translations will only improve.
However, ongoing challenges, particularly those related to polysemy, cultural context, and data bias must be adequately addressed. The field continues to evolve, promising insights into more comprehensive and adaptable translation solutions. The potential for future innovations, such as unsupervised learning, reinforcement learning, and multimodal translation, positions machine translation as a key player in driving effective global communication.
As we navigate an interconnected world where effective communication is paramount, the integration of machine learning into language translation stands to foster not just business efficiency but also cross-cultural understanding. Ensuring that these advancements are pursued responsibly and inclusively will pave the way for a future where language is no longer a barrier but a bridge connecting the diverse tapestry of human experience.
Understanding Machine Translation Evaluation Metrics in ML ModelsIf you want to read more articles similar to Utilizing Machine Learning for Contextual Language Translation Enhancements, you can visit the Language Translation Tools category.
You Must Read